In order to be effective and profitable, any company in the retail industry requires careful planning to ensure customer satisfaction and subsequent sales. When a customer walks into the store or browses the website, they expect to find the items they need readily available. Stocking items involves ordering them well in advance, which can be challenging to balance with demand. That is why retailers need predictive data analytics to forecast customer buying patterns. Besides inventory management, personalized item recommendations, and pricing optimization, retail predictive analytics gives decision-makers answers to strategic questions such as the need for keeping or restocking product lines, profitability of stores, or expansion potential.
So, let's take a closer look at use cases of predictive analytics in retail and find the best way to unlock its business value while avoiding common challenges.
Understanding predictive analytics for retail
The more insights a business has about its customers, the better it can meet their needs. Predictive analytics in retail industry is like a crystal ball that can foresee future trends and customer behaviors. By implementing it, you can anticipate what your customers want next week, month, or even season.
At its core, predictive analytics in retail involves analyzing historical data to make informed assumptions about future events. This data can come from various sources, such as sales records, customer feedback, social media trends, and more. By leveraging advanced algorithms and Machine Learning techniques, retailers can uncover patterns that are not immediately obvious.
What key tasks do data analytics perform to gain insights from your data? Once experts define what data is needed for your unique purposes, they move on to the following steps:
- Description. During this step, data analysts summarize the gathered data and present it to decision-makers to help them better view the current retail operations. The output of this stage is relatively simple, consisting of straightforward summaries, charts, and graphs.
- Extrapolation. The key objective of this task is to predict what your data will look like in the future, relying on historical and current data and applying statistical techniques.
- Inference stands for analyzing causal relationships within the data to understand the impact of various factors. For example, determining how a 10% discount influences the overall sales volume.
Related: A fresh look at Machine Learning in retail: Top 10 applications
Use cases of predictive analytics in retail
Now that we know how raw data is translated into actionable insights, we can explore diverse predictive analytics for retail examples and use cases across the industry:
Inventory management
One of the applications of predictive analytics retail benefits the most from is inventory management. According to MYOS, addressing overstocking and understocking issues can lead to a 10% reduction in inventory costs. That is why retailers want the shelves always to be stocked just right—never too full, never too empty. When demand and supply are not adequately assessed, it can cause the absence of high-demand products, ultimately decreasing sales.
This, in turn, results in inaccurate data for future analysis and leads to distorted insights about demand. Predictive analytics for retail helps businesses forecast product demand, ensuring they stock the right amount of inventory to meet customer needs without overstocking or understocking. Retailers can leverage advanced algorithms and ML models to analyze historical sales data, forecast what products to stock, and pick the best locations, times, and prices for selling these products. With data-driven insights, retailers can reduce inventory costs, prevent lost sales opportunities, and boost revenue.
Revenue prediction
How can retailers know if they are ready for significant investments such as potential store expansion? With retail predictive analytics, businesses can extract insights from historical data, current sales trends, and market conditions to project future revenue. By utilizing these insights, retailers can assess their financial health and determine whether they have the necessary cash flow and profitability to support expansion. In addition, predictive models can simulate various what-if scenarios. By changing variables, you can understand the impact of external factors, such as seasonal fluctuations, on revenue streams. That way, relying on predictive analytics, retailers can strategically plan and execute growth initiatives with confidence.
Customer segmentation
Segmenting customers is crucial for creating targeted marketing strategies and improving customer engagement. According to Emergen Research, 80% of enterprises that utilize segmentation report increase in sales. Predictive analytics enables retailers to analyze vast amounts of customer data and identify segments based on purchasing behavior, preferences, and demographics. After the data has been segmented, retailers can then tailor your marketing efforts to each specific group. This allows for creating personalized promotions and targeted advertising campaigns that resonate with each customer segment.
Here's one of predictive analytics in retail examples that illustrates its role in customer segmentation. Suppose, a fashion retailer used predictive analytics to segment its customer base into frequent shoppers, seasonal buyers, and trendsetters groups and design marketing campaigns for each. For frequent shoppers, they offered exclusive early access to new collections and special loyalty rewards. Seasonal buyers received targeted promotions during key shopping periods like back-to-school and holiday seasons. Finally, trendsetters were engaged with sneak peeks of upcoming trends and personalized style recommendations. By addressing the specific needs of each segment, the retailer enhanced the shopping experience and built customer loyalty.
Personalized recommendations
People expect tailored experiences—as stated in the McKinsey research, 76% of customers become frustrated when retailers don't offer personalized recommendations. Therefore, implementing retail predictive analytics for personalization is a win-win for businesses and customers. While retailers can benefit from increased customer loyalty and higher sales, customers enjoy a shopping experience uniquely suited to their preferences.
How does it work? Predictive analytics engines collect data such as customers' purchase history, browsing behavior, social media interactions, and demographic information and use it to make recommendations. When an item is out of stock, these engines can also suggest alternative products. In addition to item recommendations, these engines anticipate when a customer is ready to make a repeat purchase and send timely reminders or offers.
More on the topic: Personalization in retail: Trends, technologies, and tips
Pricing optimization
Understanding which products will be in demand is one challenge; determining the optimal price customers are willing to pay for them is another. Some customers are willing to buy their preferred items regardless of cost, while others hold out for discounts or buy in larger quantities. With predictive analytics, retailers can determine the optimal prices for each customer and pinpoint the most effective pricing strategies to boost sales.
According to Competera, predictive analytics for retail can lead to up to 15-20% improvements in pricing efficiency. How does it work in practice? First, Machine Learning systems can calculate the optimal timing for price reductions or increases based on historical sales data and current market trends. Moreover, predictive analytics can assess the impact of sales frequency on customer behavior. If certain products are often discounted, customers might delay purchases until sales occur. Businesses can determine an optimal sales frequency that will benefit both customers and retailers by analyzing sales data.
Boost your competitive edge – explore the top price monitoring tools for retail and ecommerce!
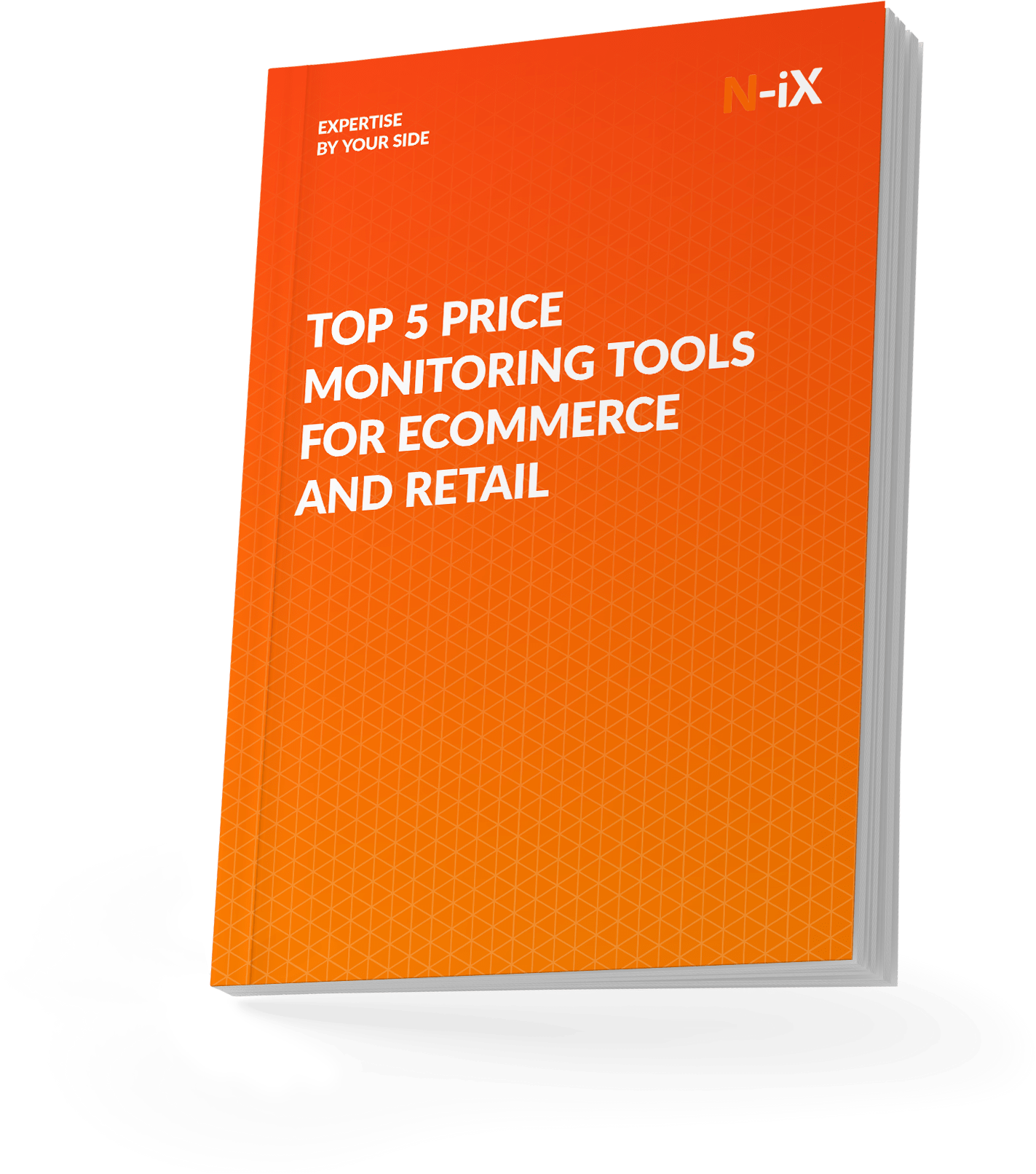
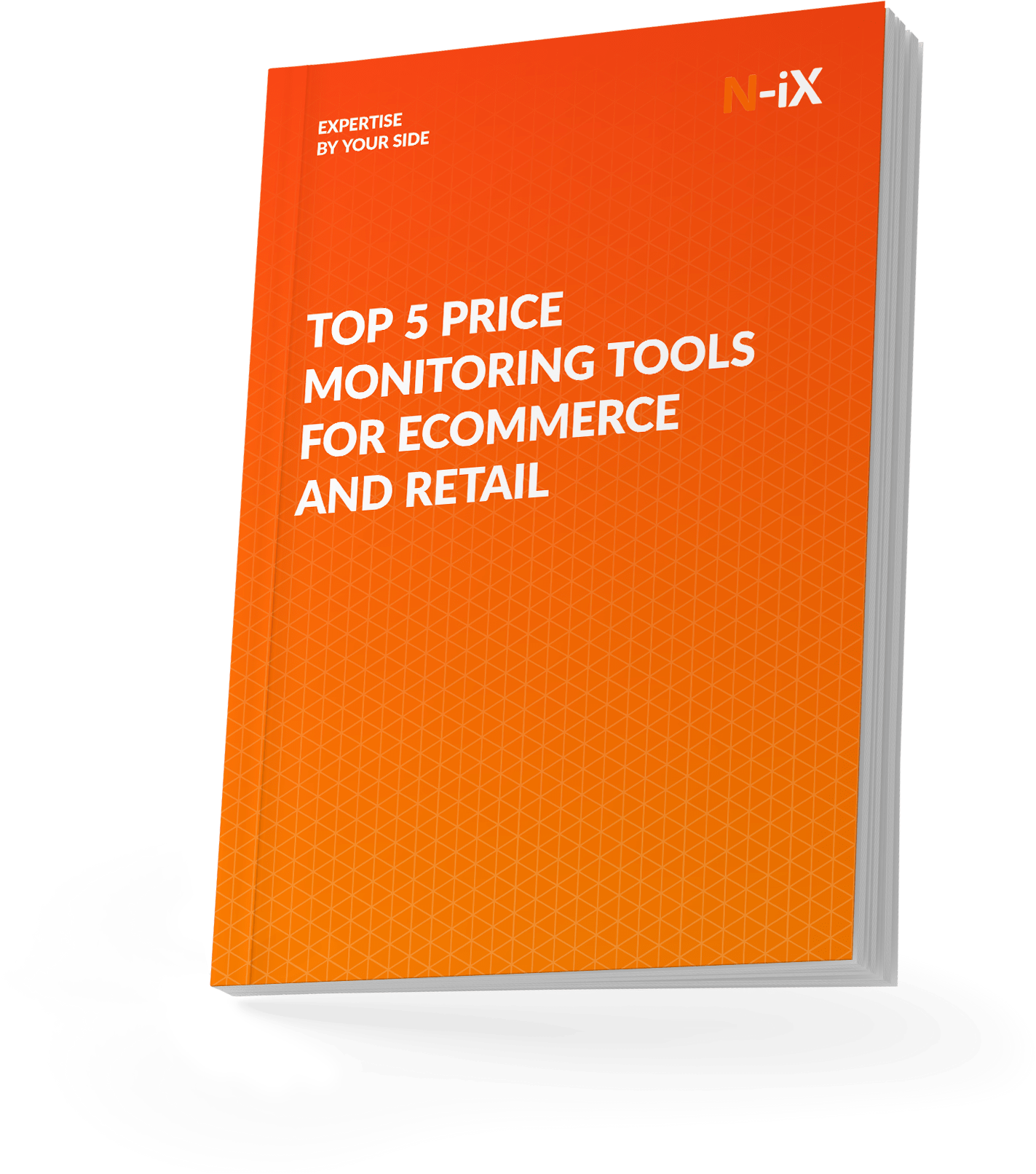
Success!
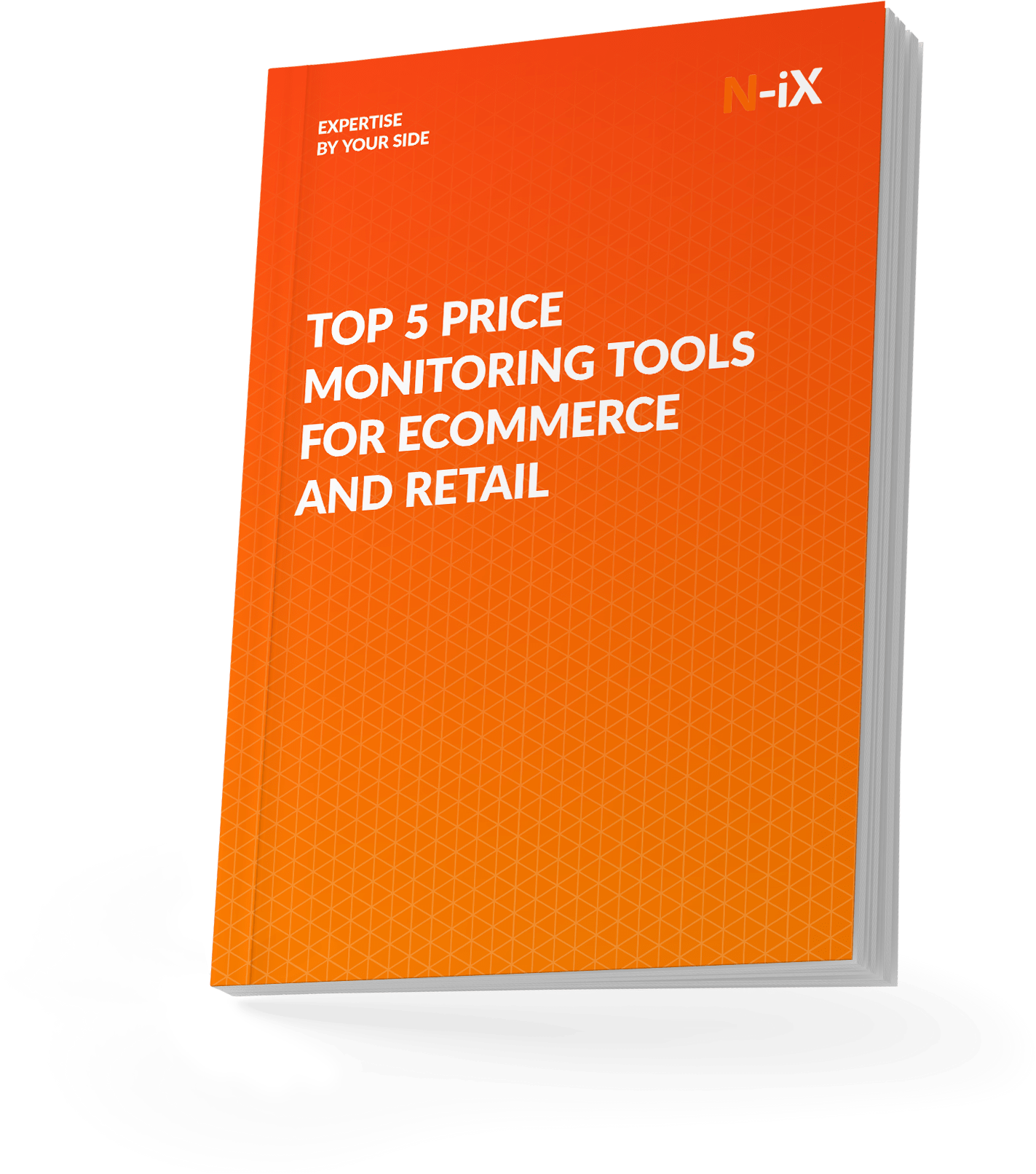
Product bundling
In every retail sector, there are products that are often purchased together, such as shampoo and conditioner, bicycles and helmets, smartphones and cases. Customers are likely going to buy them together anyway. So why invest time, money, and effort to market these products separately? One of the use cases of retail predictive analytics is product bundling. Predictive analytics helps retailers discover hidden connections between products that may not be immediately obvious.
Store expansion
Before you decide to open stores in new locations, you must analyze multiple factors such as demographic trends, competitor presence, and economic conditions. Predictive models can forecast potential sales and profitability for proposed store locations, helping retailers make informed decisions about where to invest. For example, a retailer might use predictive analytics to identify an under-served area with a high concentration of their target customers, ensuring that new stores are strategically located for maximum impact.
Targeted marketing campaigns
One of the key ways for retailers to maximize ROI is to reach their customers with accuracy, delivering personalized messages that resonate with their unique preferences and behaviors.
Predictive retail analytics will help you optimize your marketing and advertising campaigns, delivering the right messages to the right customers. For instance, customers who regularly purchase luxury fashion items are more likely to respond to exclusive previews of new designer collections. In contrast, those who tend to buy clothing during sales might respond better to messages about upcoming discounts. That way, retailers ensure your budget is allocated to the most effective keywords and audience segments, reducing wasted spend on underperforming or irrelevant ads.
Fraud detection
As the Juniper Research report states, ecommerce businesses lose around $48B to fraud every year. Fraud detection and prevention is one of the predictive analytics use cases in retail. Predictive models can detect unusual patterns and mark potentially fraudulent transactions by analyzing vast amounts of transaction data in real-time. These models use Machine Learning algorithms to identify anomalies and compare them with known fraud indicators, such as irregular purchasing behaviors, atypical transaction amounts, and unusual account activity. By integrating predictive analytics into your fraud detection strategy, you can significantly reduce the risk of financial loss, protect your reputation, and ensure a safer customer shopping experience.
Explore more insights on fraud detection with Big Data analytics and Machine Learning
Key challenges of adopting predictive analytics in retail industry and their solutions
Predictive analytics offers great potential for transforming the retail industry, but its implementation comes with significant challenges. Let's delve into the complexities faced when integrating predictive analytics into retail operations and discover their solutions:
Data silos
Data silos occur when data is isolated in systems within an enterprise, making it difficult to access and analyze comprehensively. In the retail industry, data might be spread across various channels, such as online sales, in-store purchases, supply chain management, and customer service. This fragmentation can hinder your ability to get a helicopter view of your retail operations, leading to incomplete or inaccurate predictive models.
Our solution: To help our clients overcome the challenge of blind spots and data inconsistency, we provide comprehensive data integration services. Our data experts employ advanced ETL (Extract-Transform-Load) processes and tools to consolidate data from various sources into a unified data warehouse or data lake. That way, retailers can gain a 360-degree view of their operations, enabling more accurate predictive models and improving decision-making.
Outdated infrastructure
Among businesses aiming to implement predictive analytics for retail, examples of outdated legacy systems occur quite frequently. Such IT infrastructure lacks the capacity to support advanced predictive analytics. Legacy systems may not have the necessary processing power, storage capabilities, or software compatibility to handle large volumes of data and complex analytical processes. This can result in slow performance, limited scalability, and increased maintenance costs.
Our solution: Our way to address the limited capabilities of clients' legacy systems is to upgrade or build new ones from scratch so that these systems support modern data analytics tools and platforms. We can also help retailers move to cloud-based infrastructure that can provide scalable, flexible, and cost-effective solutions for data storage and processing. Cloud platforms (AWS, Google Cloud, and Microsoft Azure) offer advanced analytics services that can be integrated with existing systems.
Privacy concerns
The first thing that pops up when you browse any retail website are cookies. That's how customers give consent to the collection and use of their data. Since predictive analytics in retail involves gathering and analyzing large amounts of customer data, this raises significant privacy concerns. Regulations such as the General Data Protection Regulation (GDPR) impose strict requirements on collecting, storing, and using personal data. Non-compliance with these regulations can result in severe penalties and damage the brand's reputation.
Our solution: We prioritize data protection, helping retailers build customer trust. N-iX data analytics practices are fully compliant with GDPR and other relevant regulations. This not only protects your business from legal repercussions but also enhances customer loyalty and confidence.
More on the topic: Getting the most out of Big Data in retail: Benefits and use cases
Wrapping up
By introducing predictive analytics, retail businesses can transform and optimize their operations, enhance customer experiences, and drive significant growth. To harness the full potential of this powerful tool, it is crucial to eliminate any potential hurdles that could impede its effectiveness.
While the journey to integrate predictive analytics might appear daunting, it is an essential step in today's competitive market. Partnering with N-iX will help you tackle any challenges head-on, making the implementation process more efficient and less complex.
With a strong data team of 200 experienced data engineers and 22 years of market experience, N-iX can implement a wide range of analytical solutions powered by Data Science, Machine Learning, and AI. Our specialists are well-versed in the development of versatile software for the retail industry, including demand planning tools, personalized search engines, competitive pricing intelligence platforms, trend search applications, and other solutions. N-iX's client portfolio includes prominent retail and ecommerce leaders such as PrettyLittleThing and Lebara, among others. Get in touch, and let's discuss how our vast experience can make your retail predictive analytics case study a success story!
Have a question?
Speak to an expert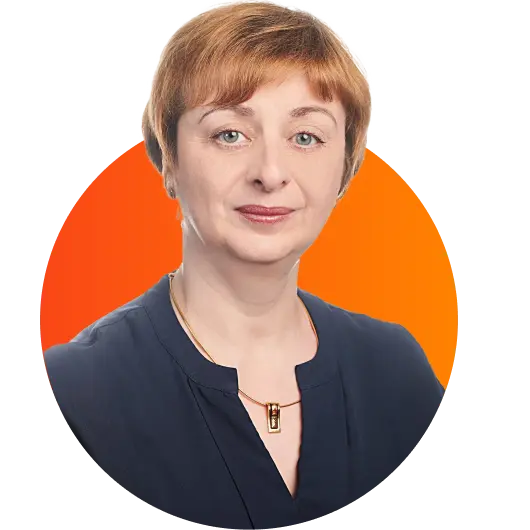