Predictive analytics in insurance is transforming the industry, helping companies address challenges such as fraud detection, risk assessment, and customer personalization with data-driven precision. According to Nasdaq, in the US alone, insurance fraud results in an estimated $308.6B loss annually, increasing premiums by an average of $900 per consumer.
Insurers can enhance operational accuracy and agility by leveraging historical and real-time data through advanced data analytics services and insurance software development. This use of predictive insights not only protects against fraud but also improves customer experience and enables insurers to adapt more effectively to market shifts, delivering substantial value across the sector.
Why do you need to implement insurance predictive analytics? What are the best ways to apply it? How to mitigate the challenges of predictive analytics implementation? Let’s find out.
Benefits of predictive analytics in insurance
Adopting predictive analytics in insurance industry offers transformative advantages that enhance operational agility, precision, and customer-centric service. Through the analysis of large datasets, insurers gain the ability to respond proactively to current challenges while preparing for future risks and opportunities. Here’s what insurers get from predictive analytics.
Greater accuracy in decision-making and risk assessment
Predictive analytics empowers insurers to make informed decisions based on clear patterns in risk factors, customer behaviors, and market trends. This precision improves underwriting, pricing, and policy management. For example, advanced risk models incorporate factors like location and lifestyle to create accurate risk profiles, leading to tailored policy pricing that balances profitability with customer value.
Cost efficiency and resource optimization
Automation and data-driven insights help insurers streamline processes, reducing operational costs and manual intervention. Predictive analytics also aids in prioritizing resources, such as directing fraud detection efforts to high-impact cases. This approach cuts expenses while enhancing responsiveness and service speed for customers.
Personalization and improved customer retention
Predictive analytics in insurance offers a deeper understanding of individual customer needs, allowing them to tailor policies and engagement strategies. Personalized products and incentives align with specific customer profiles, such as safe-driving discounts for young drivers, which help boost satisfaction and loyalty.
Proactive risk management and loss prevention
Predictive models enable insurers to foresee risks and take preventive actions, reducing claims and promoting safety. Historical data analysis helps insurers anticipate incidents and advise customers on risk-reducing measures. This proactive approach strengthens insurer-client relationships and reinforces insurers’ role in safeguarding policyholders.
Competitive advantage through innovation
Predictive analytics allows insurers to introduce such products as dynamic pricing and usage-based policies. Real-time data insights let insurers quickly adapt to market shifts and respond to evolving customer needs. This adaptability helps insurance companies to stay competitive and offer value-added services to their clients.
Discover more about intelligent automation in insurance
Key applications of predictive analytics in insurance
Predictive analytics enhances operational efficiency, improves decision-making, and boosts customer engagement in the insurance industry. Insurers can optimize processes and drive more accurate and effective outcomes by leveraging diverse data sources and advanced ML models. Here are the key applications of predictive analytics in insurance.
Risk assessment and policy optimization
Accurate risk assessment is a must for effective underwriting. Predictive analytics refines this process by analyzing diverse data points—such as lifestyle, health history, and location—to create precise risk profiles. Insurers can use these insights for predictive underwriting and policy customization, tailoring coverage and pricing to each policyholder. For instance, health insurers estimate the likelihood of future claims using applicants’ medical data, enabling personalized coverage options that align with individual risk levels.
Fraud prevention and risk mitigation
Predictive models trained on historical fraud data identify unusual patterns in claims, flagging high-risk profiles for further investigation. This proactive fraud detection saves resources by focusing on claims with the highest potential impact. Additionally, risk mitigation tools embedded in predictive analytics allow insurers to address vulnerabilities, minimizing the likelihood of fraudulent claims and boosting overall operational security. In such a way, insurance predictive analytics reduces unnecessary payouts and protects profitability.
Claims management
Predictive analytics optimizes claims processing by triaging claims according to complexity, potential cost, and risk, enabling insurers to allocate resources effectively. High-value or complex claims are prioritized for expert review, while simpler cases are processed rapidly, reducing bottlenecks and improving customer satisfaction. Moreover, predictive insights identify unusual claims that require additional scrutiny, ensuring that resources focus on claims with the highest potential impact.
Dynamic customer engagement and personalized services
Customer retention is one of the major foci in insurance, and predictive analytics equips insurers to identify potential attrition risks through patterns in interactions, claims, and feedback. This data-driven approach enables insurers to reach clients with personalized offers, policy adjustments, or discounts. This application focuses on strengthening long-term relationships through a deep understanding of customer behavior and needs, fostering loyalty and reducing churn.
Proactive risk management
Integrating predictive analytics with IoT technology allows insurers to anticipate risks and take preventive actions. IoT sensors in homes, vehicles, and industrial equipment transmit real-time data that insurers analyze to detect potential threats. To avoid costly claims, insurers can advise policyholders on preventive measures, such as fixing a malfunctioning device or addressing environmental risks. This proactive risk management reduces claim frequency and fosters a preventive relationship with policyholders.
Forecasting customer risk and health trends
Predictive analytics in insurance allows for forecasting customer behaviors and health trends for more strategic planning and product design. Analyzing factors such as age, health records, and lifestyle choices helps insurers anticipate potential health risks, supporting informed decisions about policy adjustments and wellness-focused services. These predictive foresights are especially valuable for health insurers, who can use them to align coverage options with emerging health trends and customer needs.
Read about the top 6 technology trends in the insurance industry for 2024
Implementation of predictive analytics with N-iX
Implementing predictive analytics in insurance requires a structured approach to ensure alignment with business goals, seamless integration with existing systems, and reliable performance. N-iX follows a comprehensive process to guide insurers through each phase, leveraging deep domain expertise and technical proficiency to deliver impactful results.
1. Discovery Phase
Data specialists from N-iX begin by aligning predictive analytics initiatives with your business objectives. Our experts engage with stakeholders, identify goals for underwriting, fraud detection, and customer retention, and evaluate your existing technology landscape. N-iX’s team assesses data sources, infrastructure, and other prerequisites to ensure the project is built on a solid foundation.
2. Data assessment and preparation
Next, we conduct a detailed audit of your data to identify gaps, inconsistencies, and enhancement opportunities. Our experts consolidate data from various sources, clean, and transform it to meet analytics requirements so that it is ready for modeling. Through normalization and enrichment, we create a dataset that captures essential insights into risk and customer behavior, tailored to the insurance industry.
3. Model design and customization
N-iX data scientists develop predictive models tailored to your needs, whether for optimizing underwriting, detecting fraud, or enhancing customer engagement. These models use advanced ML algorithms to extract actionable insights from large datasets. Model customization focuses on aligning with specific insurance processes to provide highly accurate predictions that support informed decision-making.
4. Pilot testing and validation
Our data experts test each predictive model in a controlled environment, ensuring it performs accurately and consistently across different scenarios. This pilot phase fine-tunes model parameters and validates performance in realistic conditions, minimizing the risk of inaccuracies in production. Pilot testing confirms that the models meet business expectations and regulatory requirements before being deployed enterprise-wide.
5. Deployment and integration
Our team integrates the validated models into your core insurance systems, seamlessly embedding them in underwriting, claims management, and customer service workflows. This integration enables real-time insights across operations, allowing teams to make data-driven decisions at every step. The experts from N-iX configure each model to function smoothly within your existing processes, maximizing operational efficiency and enhancing service delivery.
6. Continuous monitoring and optimization
After the deployment, N-iX monitors model performance and adapts the models to reflect new data patterns, industry changes, or evolving risk factors. Regular recalibration ensures the models maintain high accuracy and relevancy as market conditions shift. N-iX’s continuous optimization approach aligns your predictive analytics solutions with business goals and regulatory standards, providing long-term value.
Learn how to empower insurtech innovation through strategic partnership
Challenges in implementing predictive analytics for insurers and N-iX’s solutions
While predictive analytics in insurance can drive substantial value, businesses often face challenges in implementation. N-iX addresses each obstacle with targeted solutions, ensuring a smooth transition to data-driven operations.
Legacy systems and data silos
Many insurers rely on outdated, standalone systems that were not designed to work together. These legacy systems create data silos, where valuable information remains isolated in different departments or databases. As a result, insurers struggle to gain a comprehensive view of customer risk, claims history, and fraud patterns.
N-iX solution: We address this issue by modernizing systems and consolidating data into centralized warehouses or data lakes. These data environments provide an integrated infrastructure that supports seamless data integration, making it easier to build accurate predictive models with comprehensive, high-quality data.
Inconsistent data and governance limitations
Data must be clean, complete, and consistent for predictive analytics to provide reliable insights. However, insurers often deal with incomplete records, outdated information, and data inconsistencies. Poor data quality directly impacts the accuracy of predictive models, leading to unreliable predictions and flawed decision-making.
N-iX solution: N-iX tackles this issue by implementing data cleansing processes that standardize and enrich datasets. We also set up data governance frameworks to maintain data quality and compliance over time, ensuring that predictive models work with trustworthy data.
Lack of transparency in model operations
Advanced ML and AI algorithms greatly improve the capabilities of predictive analytics in insurance. However, the complex nature of these models can obscure the logic behind predictions, posing challenges for insurers who require clear, explainable insights to meet regulatory and operational needs.
N-iX solution: Our data experts address this challenge by designing advanced yet interpretable AI models. Our AI algorithms provide explainable outputs, allowing insurers to see why specific predictions are made. This approach builds trust in predictive insights and aligns with regulatory requirements, allowing insurers to harness the power of advanced AI with clarity and control.
WHITE PAPER
Build an effective Generative AI strategy for your business – get the step-by-step guide!
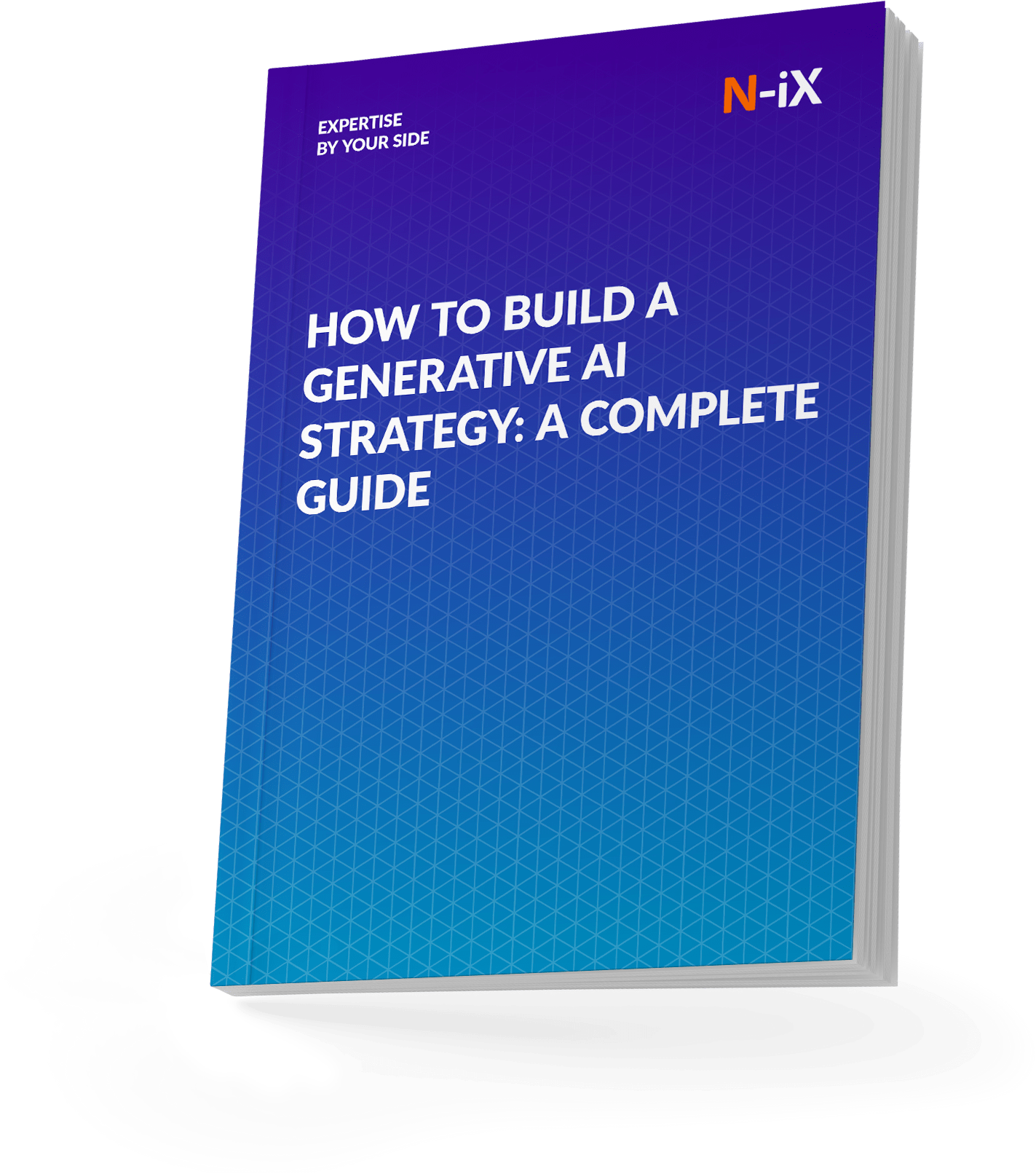
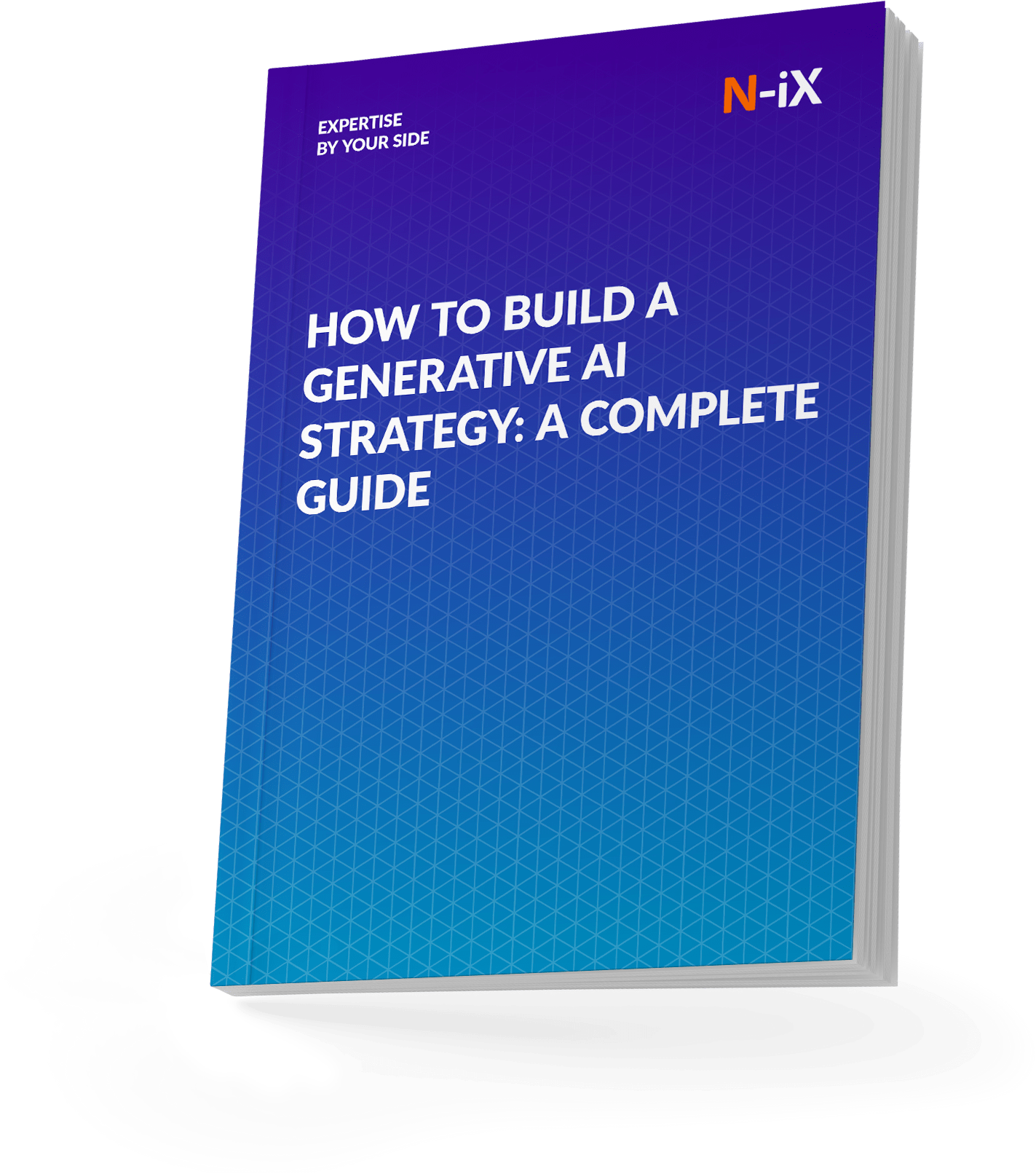
Success!
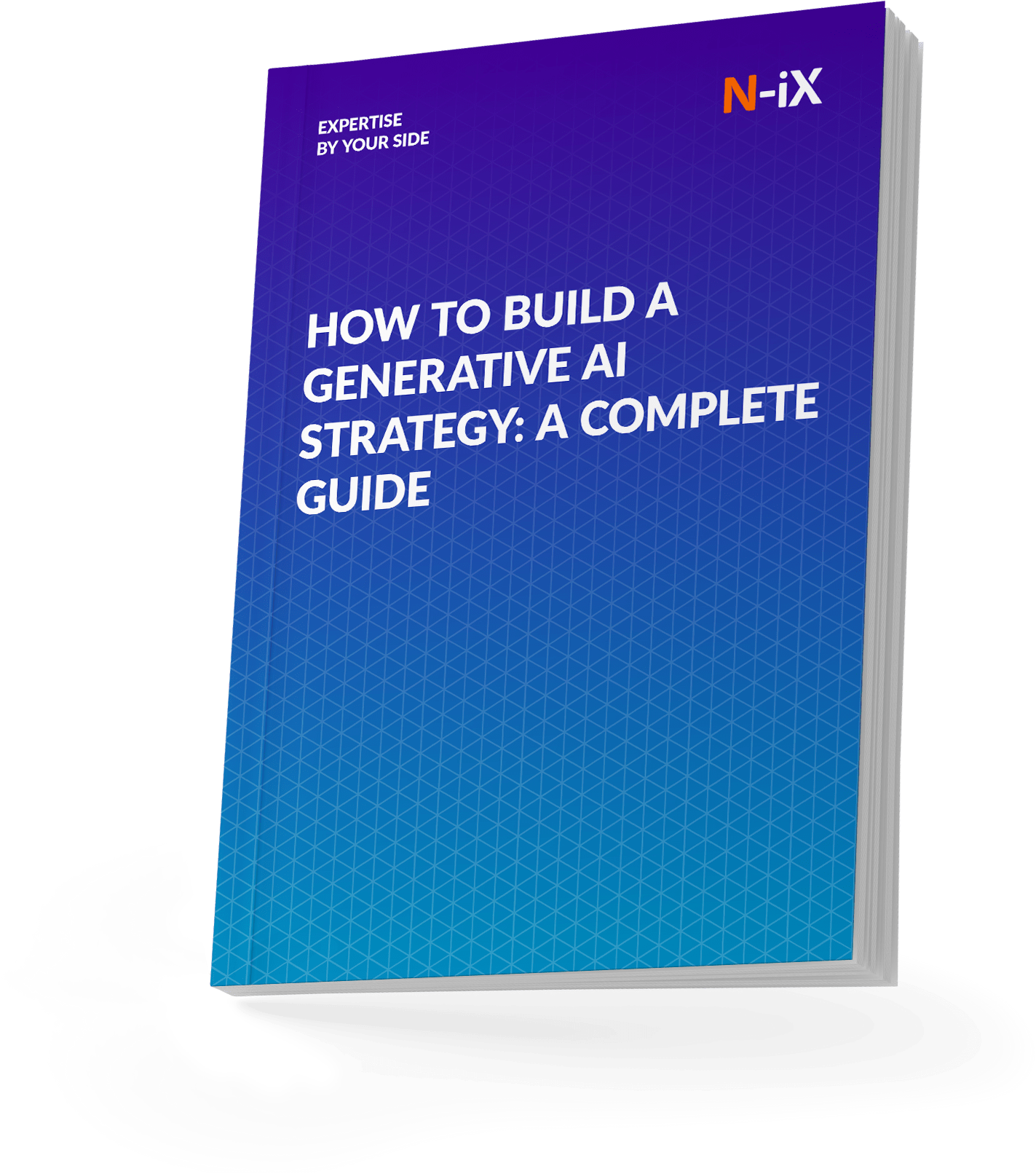
Limited scalability and operational performance
As insurers begin to use predictive analytics more extensively, the volume and complexity of data grow. Predictive models can suffer from slow processing times and limited capacity without scalable infrastructure, making them less effective in high-demand situations.
N-iX solution: N-iX helps insurers implement scalable cloud solutions and high-performance infrastructure, ensuring that predictive models can handle increasing data loads without compromising on speed or accuracy. This approach allows insurers to scale predictive capabilities seamlessly as their data needs expand.
Read more about how to make the most of predictive analytics consulting
Wrap-up
Predictive analytics offers insurers tools to manage risks, enhance customer experience, and drive operational efficiency. Data-driven insights allow insurers to anticipate risks, streamline claims processing, and develop innovative products that respond to changing market demands. This proactive approach strengthens insurer-client relationships and boosts operational efficiency, making predictive analytics an essential asset for insurers seeking a competitive edge.
At N-iX, we help insurers leverage predictive analytics in insurance for sustainable growth and competitive advantage. With a team of 2,200 professionals, including 200 data specialists, we bring 22 years of industry experience to every project. Our expertise is backed by a successful track record of over 60 data-focused projects, enabling us to address complex challenges in insurance.
N-iX provides a comprehensive suite of predictive analytics tools, including real-time fraud detection, personalized pricing models, and risk assessment solutions. We also offer customer lifecycle management systems to streamline onboarding and claims, advanced cybersecurity frameworks, and automated document processing for improved operational efficiency.