The healthcare industry generates a massive amount of textual data, from doctor's notes to patient records. Finding insights within this data can transform patient care. NLP, or Natural Language Processing, provides advanced tools to analyze this text, enabling more accurate diagnoses, personalized treatment plans, and optimized healthcare delivery. As a result, healthcare organizations are increasingly seeking specialized healthcare software development services to integrate NLP solutions and harness this potential.
NLP teaches machines to comprehend and generate human language, relying on techniques from Machine Learning and its subset, Deep Learning.
Whether managing data overload or communication gaps, NLP can tackle these challenges by summarizing complex medical records and patient narratives into clear, concise formats. This improves communication and collaboration between healthcare providers and allows for better analysis of vast amounts of clinical data. By automating repetitive procedures such as data entry and coding, NLP frees up time to focus on patients and leverage insights gleaned from the data. Additionally, NLP can simplify complex medical terminology, generating patient-friendly summaries of diagnoses and treatment plans. This fosters better patient understanding and engagement, ultimately empowering healthcare providers to work more efficiently and deliver better patient care. Let's look in more detail at the key applications and use cases of NLP in healthcare.
Key applications of NLP in healthcare
NLP techniques include Optical Character Recognition (which transforms physical documents into a digital format) and Named Entity Recognition (which identifies key details like patient information, locations, and medications). NLP tackles healthcare challenges with tools like sentiment analysis, text classification, and topic modeling. Let's explore how healthcare providers can apply NLP applications in healthcare to reduce costs and improve patient care.
Clinical documentation analysis
Clinical documentation analysis is a cornerstone application of NLP in healthcare. By processing large volumes of unstructured text data within medical records, NLP automates time-consuming tasks and unlocks valuable clinical insights.
- Automated coding: NLP automates assigning standardized medical codes to diagnoses, procedures, and medications within medical records. This improves coding accuracy, streamlines billing processes, and facilitates healthcare data analysis.
- Risk prediction: By analyzing clinical data, NLP can pinpoint patients at risk for a particular health condition. This enables early intervention and preventive measures.
- Clinical decision support: NLP can extract relevant information from clinical documents to support decision-making processes. By identifying patterns and correlations within patient data, NLP helps healthcare providers make evidence-based decisions, improving the quality of care.
- Documentation quality improvement: NLP tools can review clinical documents for completeness and consistency, flagging missing or ambiguous information. This ensures higher-quality documentation, which is essential for accurate diagnosis and treatment.
Patient engagement
NLP in healthcare fosters improved patient engagement through interactive tools and insightful analysis of patient feedback.
- Chatbots: NLP-powered chatbots provide patients with 24/7 access to information, answer basic healthcare questions, schedule appointments, and offer medication reminders. Research published in JAMA found that participants favored responses from ChatGPT over physician responses nearly 80% of the time. Moreover, they rated the AI-generated responses as higher quality and more empathetic.
- Sentiment analysis of patient feedback: NLP analyzes patient feedback surveys and reviews to understand patients' sentiments about care experiences. This allows healthcare providers to identify areas for improvement and enhance patient satisfaction.
Drug discovery and development
By drawing on large amounts of scientific literature, NLP accelerates drug discovery and development.
- Analyzing medical literature: NLP efficiently processes vast datasets of medical research papers to identify potential drug targets and treatment pathways.
- Identifying potential drug targets: By analyzing scientific text, NLP can pinpoint promising molecules with therapeutic potential, expediting drug discovery.
Personalized medicine
One of the promising NLP use cases in healthcare is tailoring treatment plans for individuals based on their unique data.
- Tailoring treatment plans: NLP can analyze a patient's genetic information, medical history, and other necessary data to recommend personalized treatment approaches that optimize outcomes.
- Adverse event detection: By processing patient records and reports, NLP can detect and analyze adverse drug events, helping to improve drug safety and post-market surveillance. NLP can analyze various medical data sources to predict potential drug interactions, helping to ensure patient safety and optimize therapeutic strategies.
Remote monitoring and telemedicine
Using NLP, remote patient monitoring and telemedicine consultations can be enhanced in a number of ways.
- Processing data from remote monitoring devices: NLP analyzes data from wearable devices and sensors to track vital signs, medication adherence, and potential health risks, enabling timely interventions.
- Telemedicine consultations: NLP can analyze transcripts from telemedicine consultations to identify key points, generate summaries, and facilitate more efficient communication between patients and providers.
Improved analysis of medical imaging analysis
In medical imaging, NLP is emerging as a powerful tool, automating analysis and generating textual descriptions to assist healthcare professionals. This enriched analysis can improve radiologists' work and refine scan interpretation. By analyzing X-rays and MRIs, NLP can potentially find patterns and anomalies that human eyes might miss, resulting in earlier and more precise diagnoses. Additionally, advancements in AI-powered imaging technology have yielded significant benefits. As reported by GE Healthcare, AI has accelerated image processing speed by 30% while improving image quality and resolution. This translates to faster turnaround times and potentially even more accurate diagnoses.
Fraud detection and claims processing
Using NLP in healthcare safeguards against fraud and streamlines the claims processing process.
- Identifying potentially fraudulent claims: NLP analyzes medical records to identify patterns suggesting fraudulent billing practices, protecting healthcare resources.
- Claims processing: NLP automates tasks within the claims processing workflow, such as extracting key information and identifying potential errors, leading to faster and more accurate claim reimbursements.
NLP's capabilities can enable healthcare stakeholders to become more efficient, improve communication, and provide better patient care.
Read more: Conversational AI in healthcare: use cases
Challenges and considerations: navigating the roadblocks of NLP in healthcare
While NLP offers many benefits for healthcare, its implementation comes with challenges that require careful consideration. Here, we delve into these roadblocks and explore strategies for navigating them.
Data security and privacy
Patient data is highly sensitive, and it is paramount to ensure its security and privacy. Implementing robust security measures and adhering to data privacy regulations are crucial when utilizing NLP tools on medical records.
Accuracy and reliability
A NLP model's accuracy and reliability are critical in healthcare settings. Factors like training data quality and model interpretability play a vital role. Mitigating these challenges requires using high-quality, diverse datasets and ensuring models are transparent in their decision-making processes.
Bias and fairness
Training data can harbor biases that can be reflected in NLP model outputs. Mitigating bias requires using diverse datasets and actively monitoring models to ensure equitable patient outcomes, regardless of demographics.
Regulatory hurdles and ethical considerations
The use of NLP in healthcare is a relatively new field, and navigating the evolving regulatory landscape can be complex. Additionally, ethical considerations regarding data ownership, bias mitigation, and potential misuse of NLP models need to be addressed.
Standardization issues
Medical data formats often lack standardization, creating challenges for NLP algorithms. Strategies like data normalization and collaboration across healthcare systems can help overcome these hurdles.
Skilled talent shortage
Developing and maintaining sophisticated NLP models requires specialized expertise. Investing in training and fostering a skilled workforce is key to successfully leveraging NLP in healthcare.
Explainability
Building trust with healthcare providers and patients necessitates ensuring NLP models are interpretable. This means understanding how the models arrive at their conclusions, allowing for human oversight and intervention when necessary.
Wrap-up
In conclusion, while NLP presents exciting possibilities for healthcare, acknowledging these challenges is crucial for successful implementation. By prioritizing data security, ensuring model accuracy, addressing ethical considerations, and fostering a skilled workforce, healthcare organizations can unlock NLP's true potential and pave the way for improved care delivery.
N-iX's track record includes various successful projects in the healthcare domain where we have applied NLP and other advanced technologies to solve real-world problems. Whether you need to streamline data extraction from medical images, automate diagnosis assistance, or leverage patient feedback for improved care, N-iX's NLP expertise can help you tap the full potential of your data and transform patient care.
Get your ultimate guide on Generative AI use cases and applications!
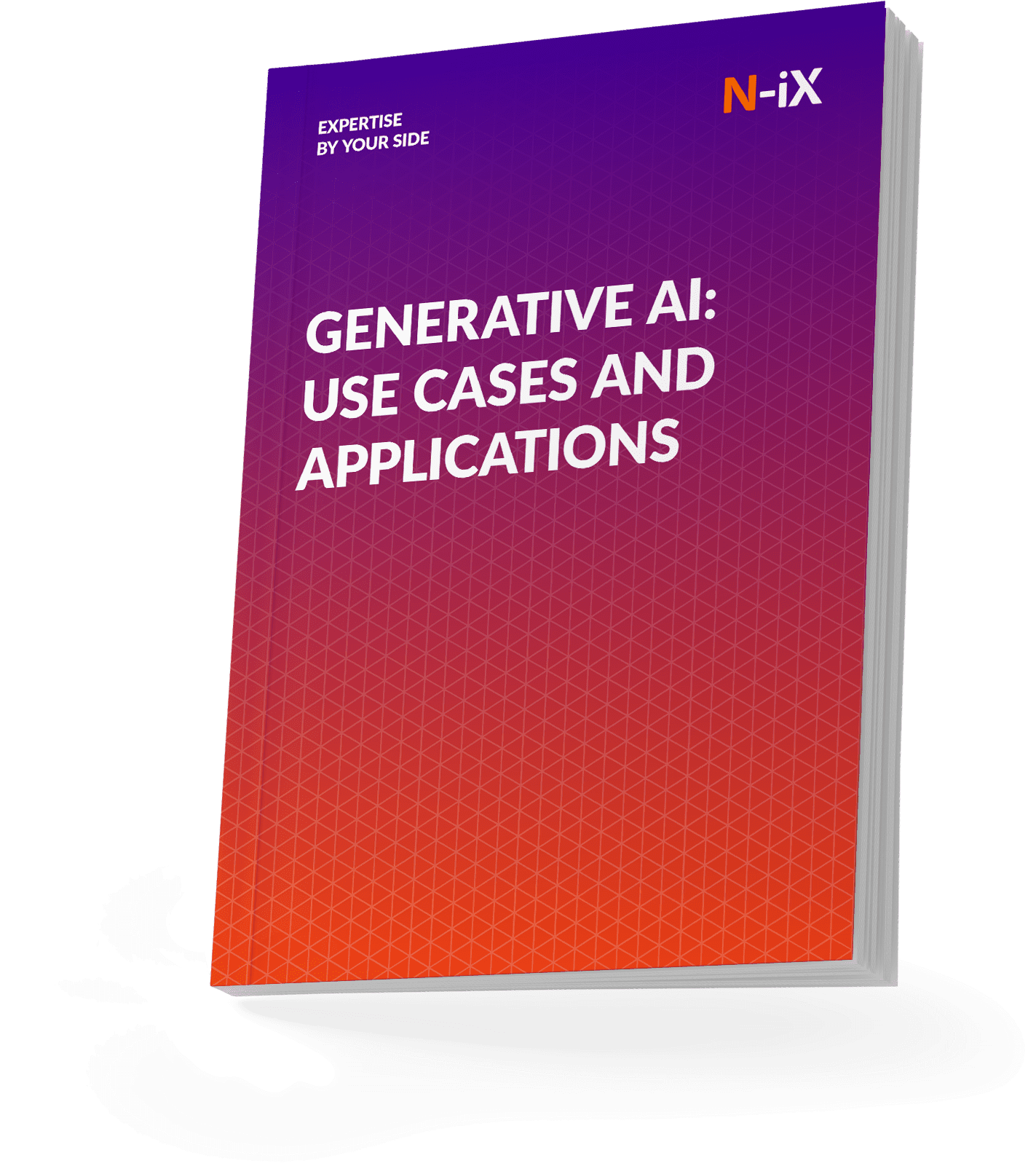
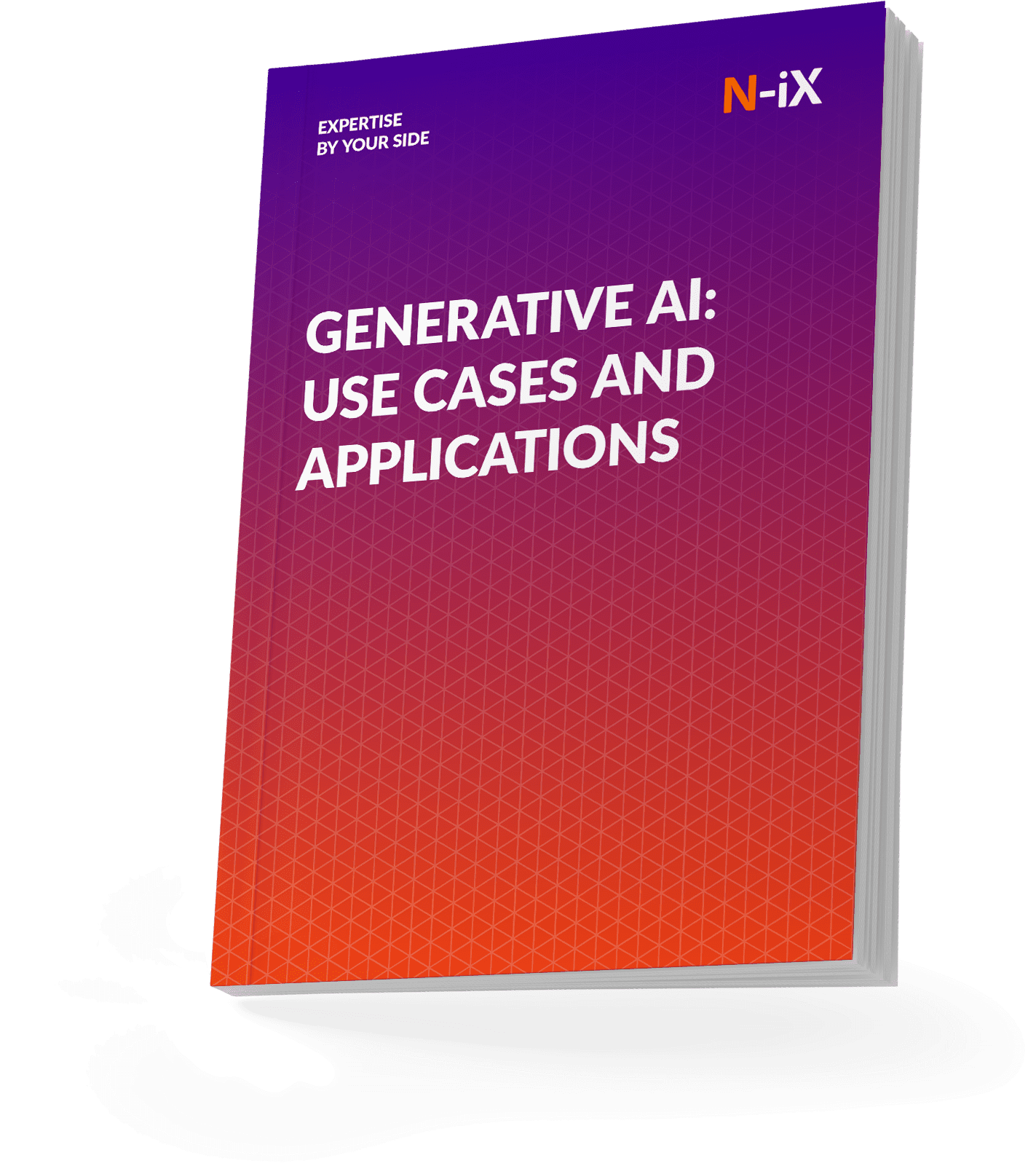
Success!
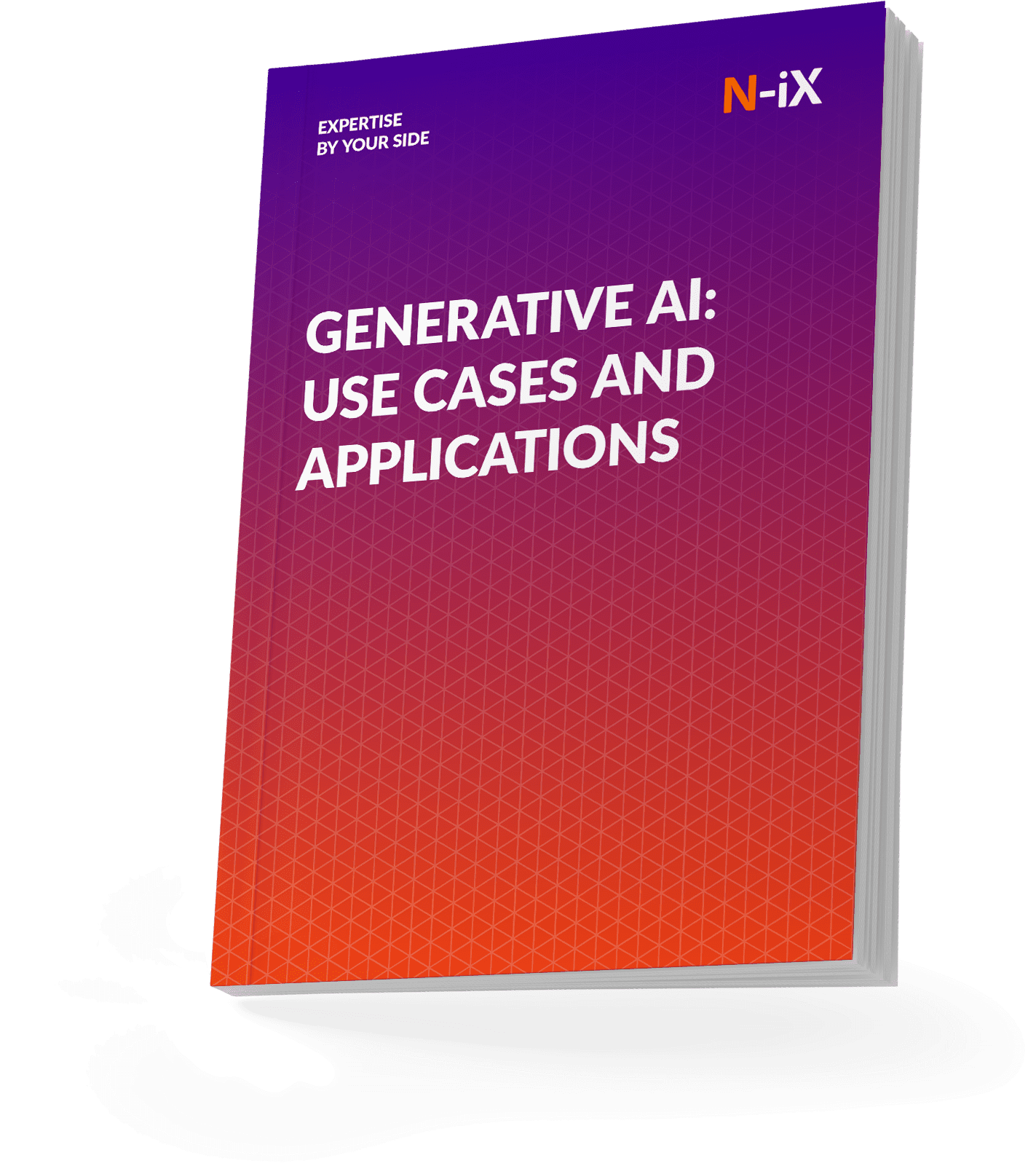