AI and ML applications transform numerous industries and create new solutions to provide end products more quickly, efficiently, and reliably. Such methodologies as AIOps (Artificial Intelligence Operations) and MLOps (Machine Learning Operations) help teams work smarter, automating tasks and making processes run smoother, which saves time and boosts productivity. While, at first glance, they may seem similar, these two concepts focus on different results.
What are MLOps and AIOps? What is the difference between MLOps vs AIOps? What are the examples of both approaches in action?
The difference between MLOps vs AIOps
MLOps is a set of practices and methodologies that aim to streamline the end-to-end Machine Learning lifecycle. It applies principles from DevOps to the specific challenges of developing and maintaining ML models in production environments.
The primary goal of MLOps services is to enhance collaboration and communication between different teams involved in the ML process, such as data scientists, ML engineers, and IT operations. MLOps incorporates automation, version control, monitoring, and other best practices to make the development of ML models more efficient, reliable, and scalable.
AIOps is a transformative practice at the intersection of AI and IT operations. In response to the escalating complexity and scale of modern IT environments, AIOps applies ML and other technologies to improve how organizations manage and optimize their IT systems. This practice aims to enhance IT operations with the help of intelligent automation, advanced data analytics, and predictive capabilities.
AIOps strategy goes beyond traditional methods of IT management, offering a forward-looking approach that responds to incidents, proactively identifies potential issues, and automates routine tasks. It aims to transform IT operations into a proactive, data-driven, and highly efficient ecosystem.
MLOps vs AIOps: in-depth comparison
MLOps and AIOps involve managing and optimizing systems operations incorporating ML or AI components. Despite sharing this overarching goal, these approaches differ in their application of AI/ML technologies and their goals. Here's a brief overview of the key differences:
Key aspects:
MLOps:
- Encourages collaboration between data scientists, ML engineers, and operations teams to ensure a seamless transition from model development to deployment.
- Applies version control to Machine Learning models, data, and code to track changes and ensure reproducibility.
- Automates repetitive tasks in the Machine Learning pipeline, such as data preprocessing, model training, and deployment, to improve efficiency and reduce the risk of errors.
- Implements Continuous Integration and Continuous Deployment (CI/CD) practices to automate Machine Learning model testing, validation, and deployment.
- Establishes robust monitoring and logging mechanisms to track the performance of Machine Learning models in production, detect anomalies, and facilitate timely responses to issues.
- Implements practices to ensure governance and compliance of Machine Learning models, including tracking model usage, managing data privacy, and adhering to regulatory requirements.
- Treats infrastructure configurations for Machine Learning environments as code, enabling consistency and reproducibility across different stages of the ML lifecycle.
- Maintains comprehensive documentation for Machine Learning models, including model specifications, training data, and performance metrics, to facilitate understanding and knowledge sharing.
AIOps:
- Collects and analyzes data for identifying patterns and trends.
- Uses ML algorithms to detect anomalies in IT infrastructure and applications.
- Automates root cause analysis to help IT operations teams pinpoint the source of issues and reduce mean time to resolution (MTTR).
- Automates routine and repetitive tasks, including incident response, remediation, and resource provision, allowing IT teams to focus on more complex and strategic activities.
- Forecasts potential issues with predictive analytics before they impact the performance or availability of IT systems.
- Integrates with IT Service Management tools to provide a holistic view of IT operations.
- Learns continuously and adapts ML models to ensure ongoing accuracy and effectiveness as new data becomes available.
- Establishes collaboration and communication between IT teams by providing a unified monitoring, analysis, and incident response platform to break down silos and foster an integrated and efficient approach to IT operations.
Scope
MLOps primarily focuses on the end-to-end lifecycle of Machine Learning models, including development, deployment, monitoring, and maintenance. It centers on streamlining and automating Machine Learning model development and deployment processes.
AIOps encompasses a broader set of operations related to IT infrastructure and operations. AIOps leverages AI and ML to enhance the management and monitoring of IT systems, including applications, networks, and infrastructure. AIOps empowers organizations to optimize system performance, preempt potential issues, and ensure heightened reliability in their IT operations.
Objective
MLOps aims to improve Machine Learning workflows' efficiency, scalability, and reliability. It also involves proactive monitoring mechanisms that help to track model performance metrics in real-time. This practice facilitates the timely identification and resolution of any deviations that may arise during production deployment.
AIOps enhances IT operations using AI and ML to analyze and respond to large volumes of data generated by various IT systems. AIOps automate routine tasks, predict issues, and facilitate proactive incident resolution. With its ability to correlate data from disparate sources and detect complex patterns, AIOps provides organizations with valuable insights into their IT infrastructure, enabling them to make data-driven decisions.
Focus area
MLOps focuses on the challenges and workflows involved in the end-to-end lifecycle management of Machine Learning models. This includes addressing complexities in data preprocessing, model training, deployment, and ongoing maintenance to ensure optimal model performance and reliability in production environments.
AIOps takes a broader perspective, considering the entire IT infrastructure landscape to enhance system performance, reliability, and responsiveness. It goes beyond the scope of individual Machine Learning models to analyze and optimize various components of IT operations, including applications, networks, and infrastructure, using AI-driven insights for proactive management and optimization.
Components
Key components of MLOps include mechanisms for model versioning to track changes and ensure reproducibility and model deployment tools for integration into production environments. Monitoring solutions are essential for real-time tracking of model performance metrics, while collaboration tools improve cooperation between data scientists and IT operations teams.
AIOps incorporates advanced components, including automated root cause analysis to identify underlying issues leading to system anomalies, predictive analytics for forecasting potential disruptions, and anomaly detection to detect deviations from expected behavior. Incident management tools enable swift resolution of issues, while integration with ITSM systems ensures seamless coordination and alignment with organizational processes.
MLOps and AIOps real-life applications
Transaction processing with MLOps
N-iX implemented MLOps as an ML-powered automated transaction processing for a finance company. Our client, a major player in the UK's prepaid cards and current accounts sector, needed to centralize their transaction decision-making.
With a significant customer base and high transaction volumes, they sought a solution to ensure instant payment processing while adhering to regulatory standards such as sanctions, anti-money laundering, and fraud regulations.
N-iX combined the customer’s previously used ML models into a single product and perfected the automation of transaction processing. Our solution enhanced operational cost efficiency, minimized manual effort, and increased the customer base by 20%.
AIOps in Gogo’s predictive maintenance solution
Gogo's case is a prime illustration of successful AIOps use cases undertaken by N-iX. As a leading provider of in-flight connectivity globally, Gogo manages a vast array of hardware, particularly satellite antennas aboard aircraft. Gogo faced considerable challenges in handling maintenance requests for this equipment. A notable issue was the prevalence of no-fault-found (NFF) requests, resulting in service downtimes and additional expenses.
Together, we designed a predictive maintenance solution that uses Data Science, ML, and AIOps. The tool accurately predicts equipment failures with over 90% precision up to 20-30 days in advance. This foresight enables our client to schedule maintenance at optimal times, such as when specific aircraft are not scheduled for flights. Through implementing AIOps strategies, Gogo has significantly elevated service quality and eliminated the financial burden associated with service downtime.
Conclusion
Comparing MLOps vs AIOps highlights overlapping applications of AI/ML in both areas, but they still differ in scope, focus, and other criteria. While MLOps excels in optimizing the Machine Learning lifecycle and enhancing data-driven decision-making, AIOps aids in IT operations and drives proactive infrastructure management.
Understanding these differences is crucial for companies to choose the right methodology and align it with organizational goals. Besides the choice between MLOps vs AIOps, you may need a reliable partner with a proven track record of delivering successful projects in your industry. N-iX has provided software development and consulting services for 22 years and delivered 30+ AI and data projects last year.
With N-iX's MLOps expertise, your company will gain optimized Machine Learning lifecycles, streamlined model deployment, and enhanced data-driven decision-making. On the other hand, our AIOps solutions empower organizations with revolutionized IT operations, proactive system failure prediction and prevention, and streamlined infrastructure management. With N-iX as your partner, you can expect unparalleled expertise of 200 Data and AI professionals who provide innovative solutions and tangible business outcomes in MLOps and AIOps.
WHITE PAPER
Explore the AI landscape of 2025—get the guide with top trends!
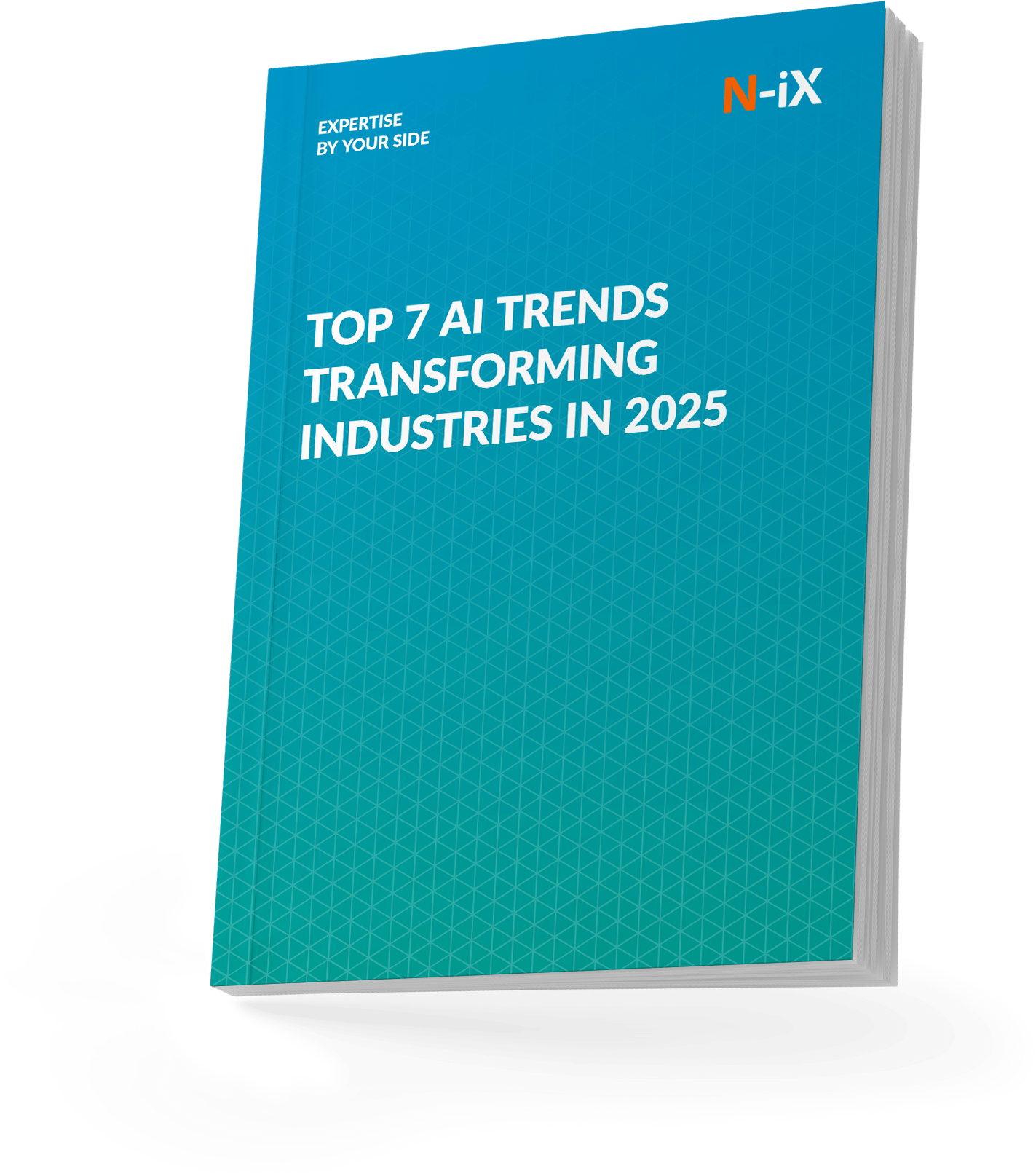
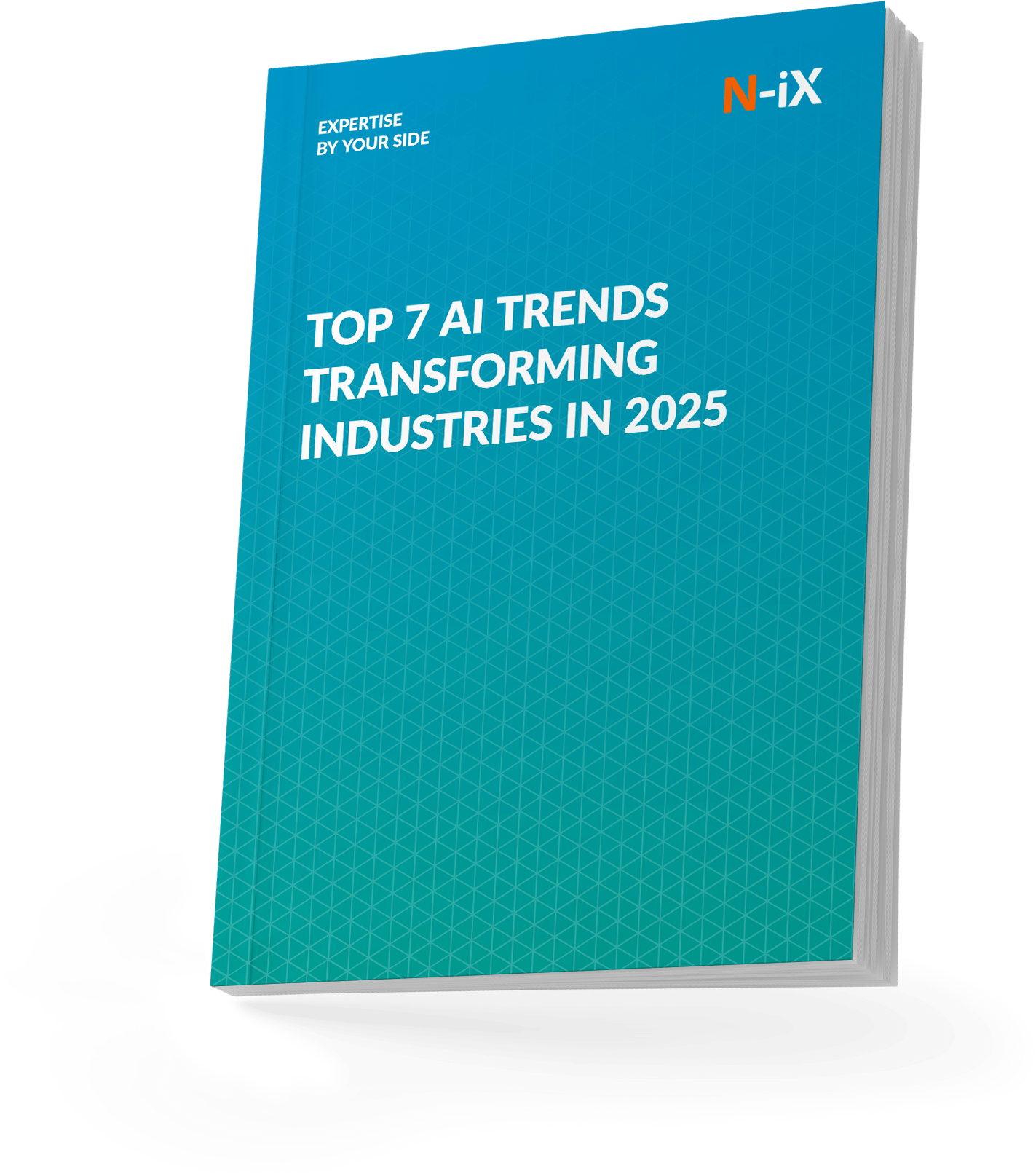
Success!
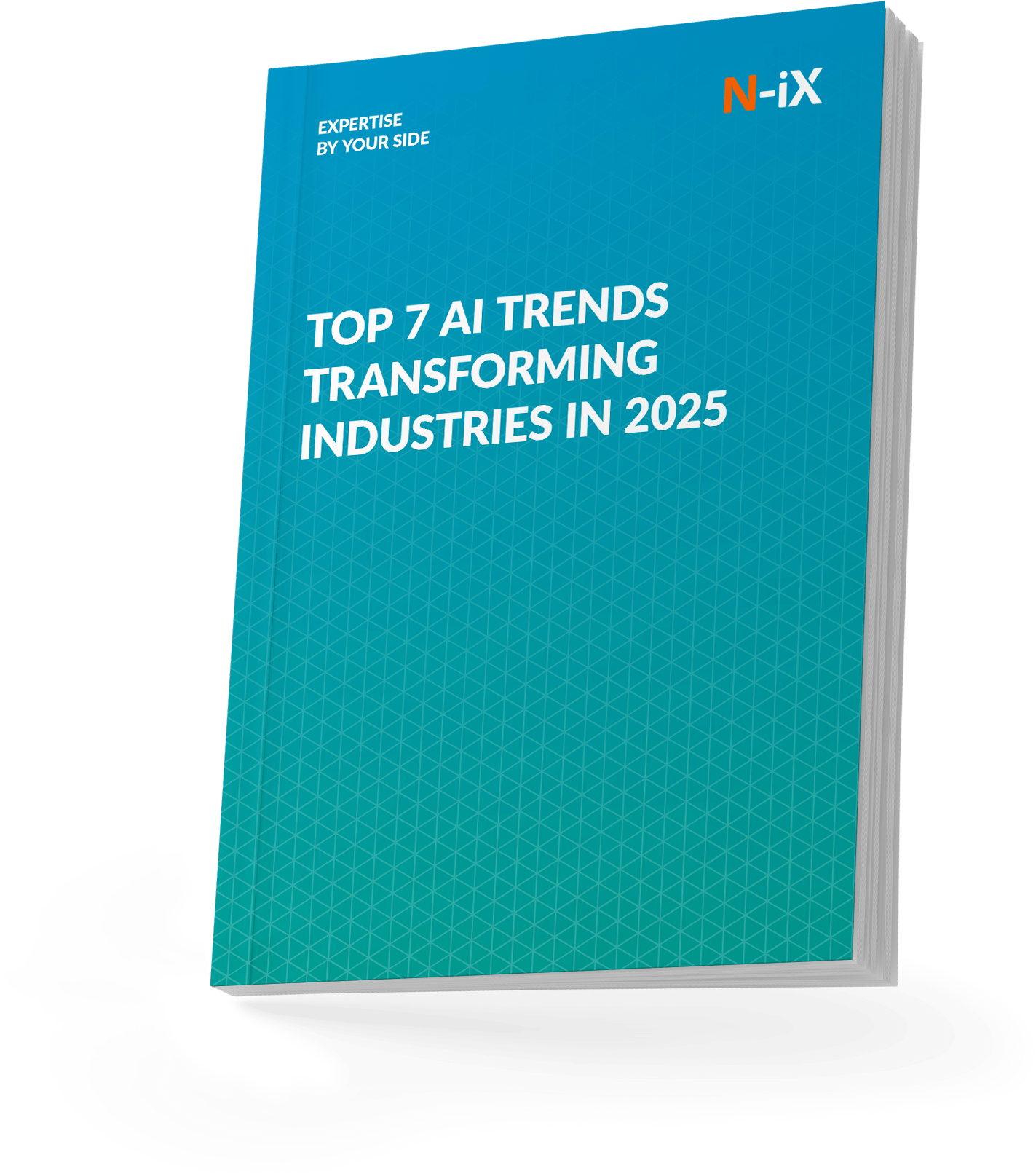
Have a question?
Speak to an expert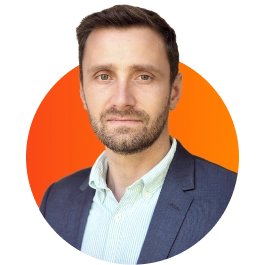