Data is everywhere in your business, but is it working for you? How many different systems in your company store customer records? Are product details similar across sales, marketing, and supply chain platforms? How often do teams fix data inconsistencies instead of focusing on strategy? Without a structured master data management (MDM) strategy, these problems pile up fast. The problem isn't a lack of data: it's the lack of control over it. A well-defined master data management strategy ensures that all critical business data is accurate, consistent, and accessible when needed. Let's break this down.
In this guide, we'll explore the key components of an effective strategy, the challenges enterprises face when implementing it, and how to ensure long-term success. If your organization struggles with unreliable data, this is where you can start with data management services.
What is a master data management strategy?
A structured master data management strategy, supported by well-defined processes and governance, enables organizations to effectively manage their critical data assets across all business functions effectively. Unlike data management, which focuses on technical aspects like storage and security, MDM strategy takes a broader view. It aligns all data initiatives with business goals to drive operational efficiency, informed decision-making, and regulatory compliance.
What can enterprises expect from implementing an MDM strategy? Beyond improving data organization, it drives efficiency, compliance, and business growth. Here's what MDM delivers at an advanced level:
- Establishes policies, roles, and responsibilities for data management and compliance.
- Defines data quality rules, removes duplicates, and enforces consistency.
- Creates a single source of key business data.
- Unifies data across ERP, CRM, and other systems for real-time access.
- Organizes and maintains structured data for usability and governance.
- Tracks data lineage for transparency and compliance.
- Implements security controls to protect sensitive data.
- Automates data validation, deduplication, and enrichment.
- Enables cross-departmental data sharing for strategic initiatives.
But how do you turn these benefits into a sustainable, enterprise-wide approach? To build a strong MDM framework, organizations should focus on six key elements:
- Governance: Defining policies, roles, and accountability structures to maintain data consistency and reliability. This process ensures that data is managed responsibly across the enterprise.
- Measurement: Continuously assessing data quality through performance metrics to ensure accuracy and completeness. Tracking progress against predefined goals helps identify and resolve issues proactively.
- Organization: Assembling the right teams, including data stewards, governance committees, and IT specialists, to support and enforce MDM initiatives. Without clear ownership, data management efforts can become fragmented.
- Processes: Standardizing workflows for data entry, validation, and integration to ensure consistency across all business units. Well-defined processes help prevent inconsistencies and errors.
- Technology: Implementing MDM tools and platforms to automate data cleansing, deduplication, and synchronization.
- Change management: Driving cultural and organizational adoption by educating stakeholders, securing executive buy-in, and aligning MDM with business objectives.
Discover more: Data management strategy 101: A guide to unlocking business efficiency
Key components of master data management strategy
The following are the key pillars of a robust, enterprise-grade MDM strategy:
Data governance
Effective data governance provides the structure to ensure that master data remains accurate, consistent, and compliant across an enterprise. It extends beyond policy documentation and regulatory adherence-it is the foundation for operational efficiency. Strong governance frameworks define clear ownership of data assets, establish accountability mechanisms, and enforce data stewardship across business units. Data governance policies define who can create, modify, and access master data while enforcing policies that maintain consistency across the enterprise.
Compliance is also a critical pillar for master data management strategy industries governed by GDPR, HIPAA, or other regulatory mandates, which must embed governance into their MDM strategies to avoid legal and financial risks. Advanced governance mechanisms incorporate metadata management and lineage tracking, making data more transparent and traceable.
Take 7 steps to design a winning data governance strategy—get the guide now!
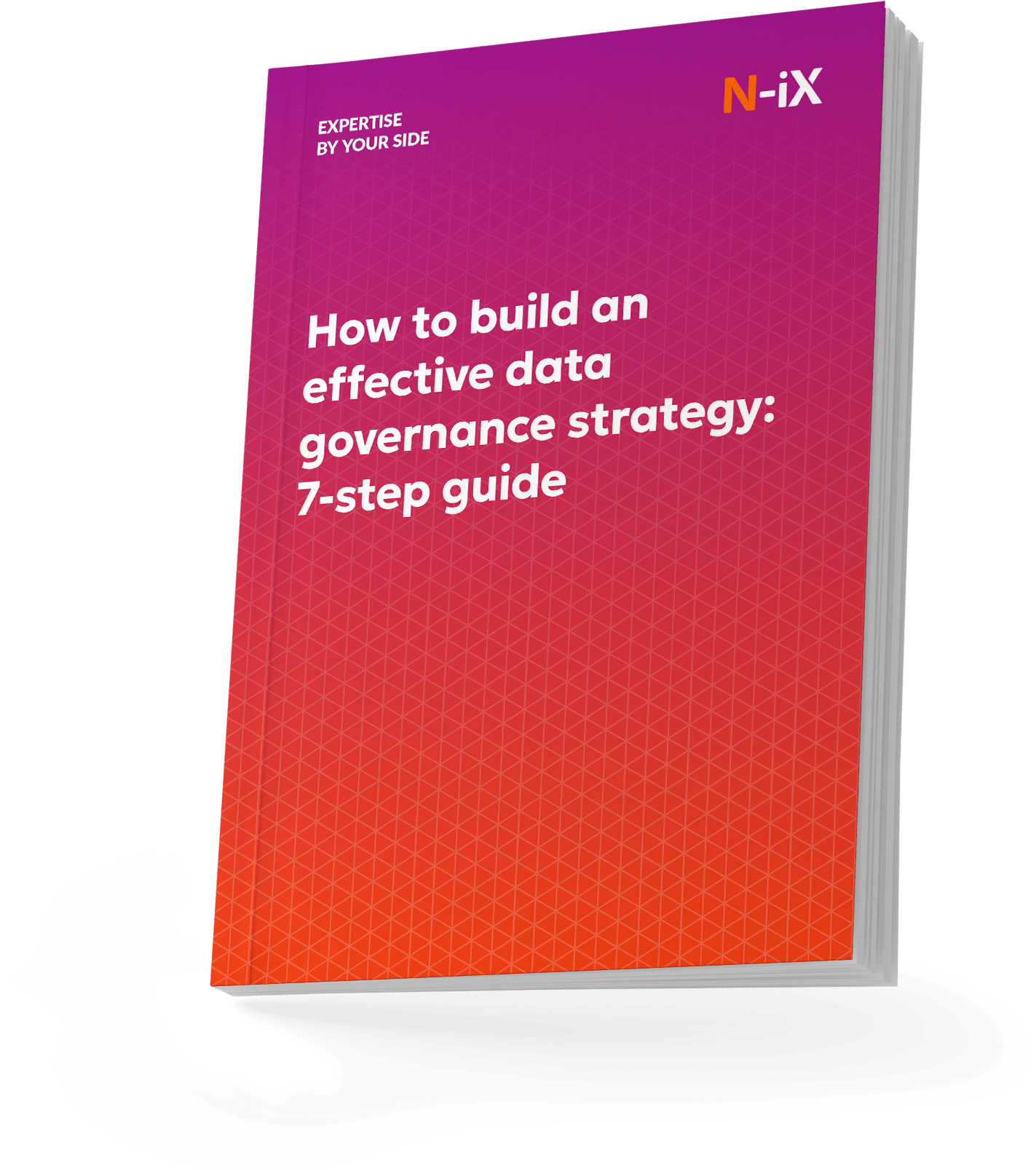
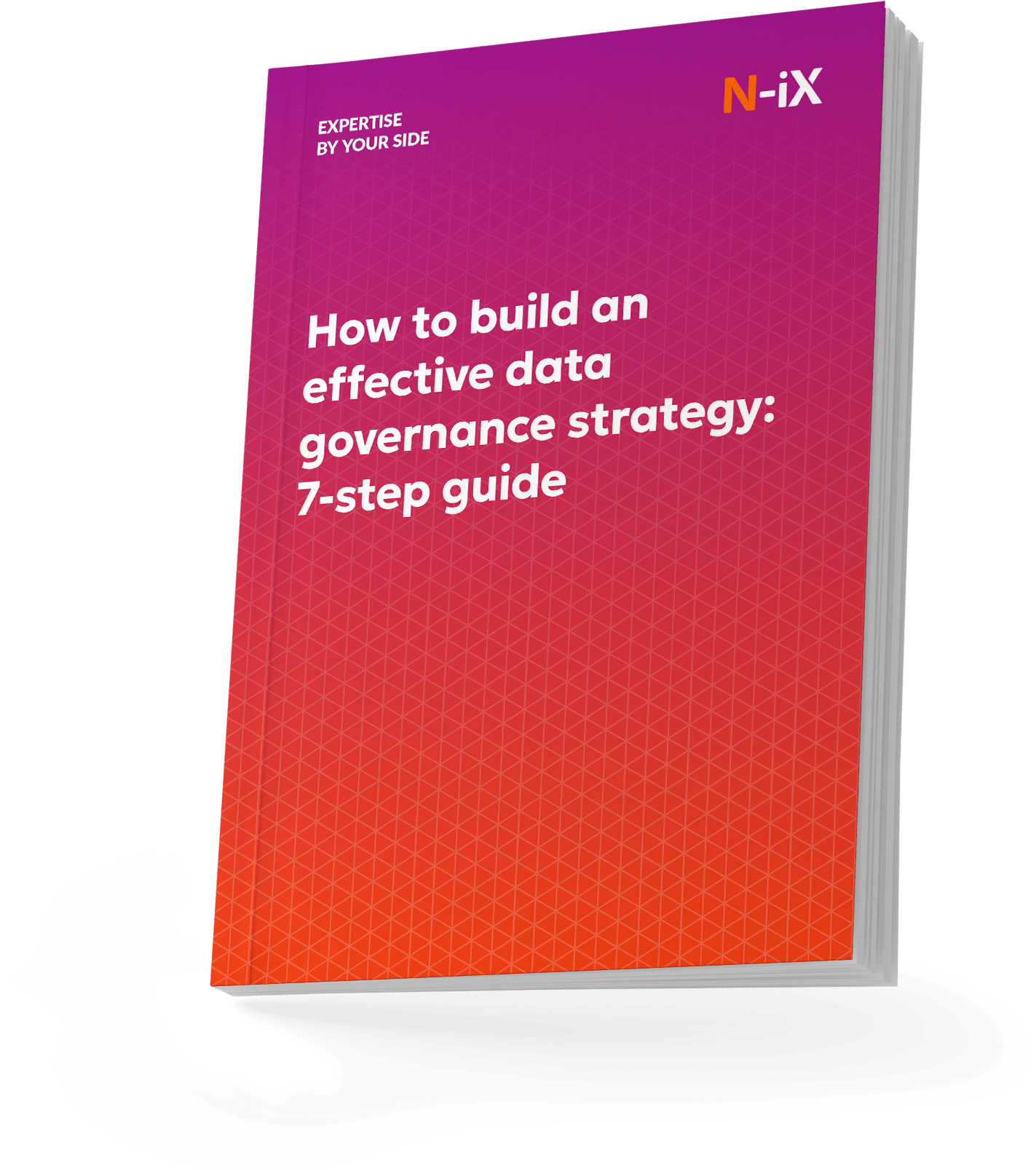
Success!
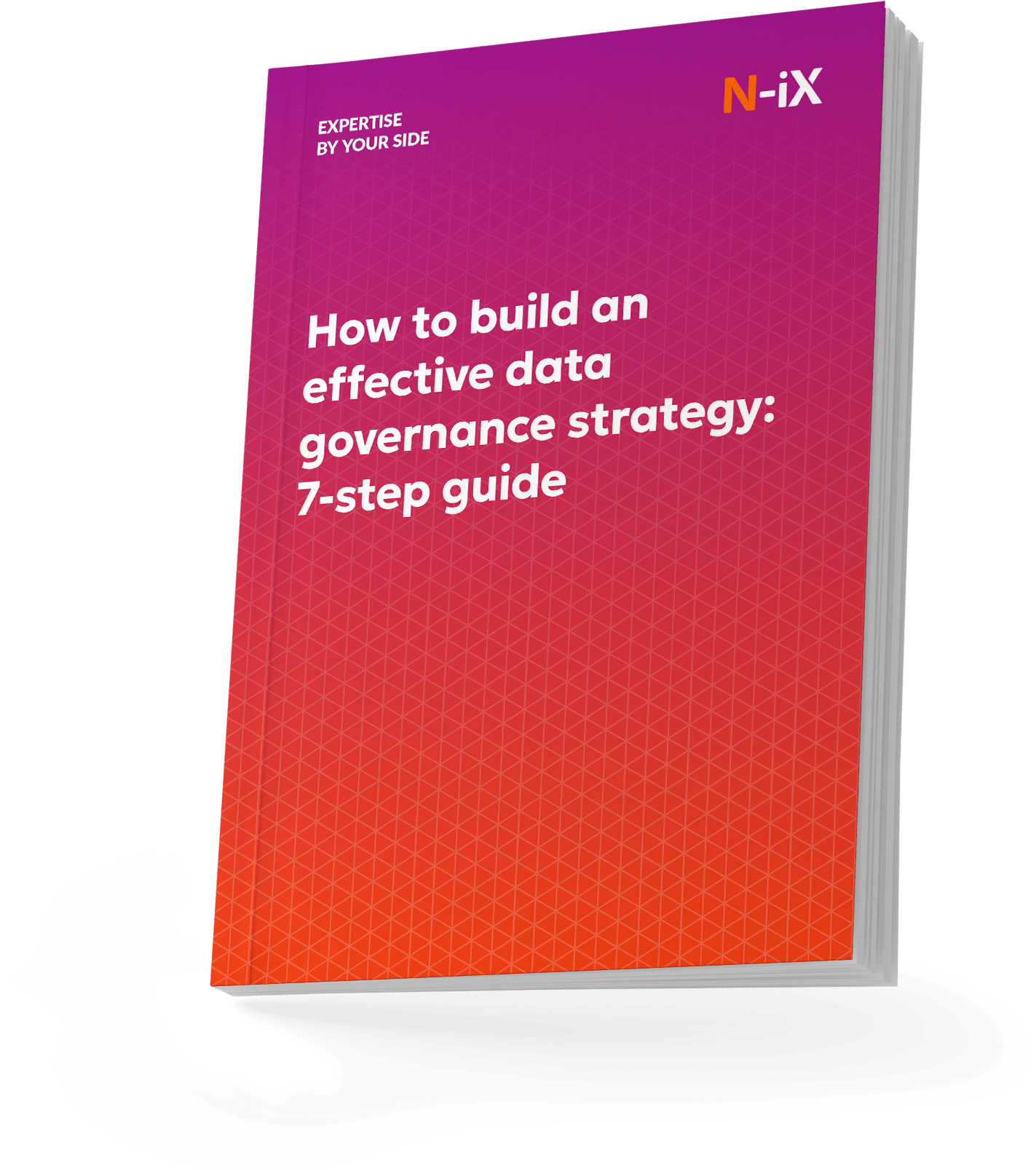
Master data domains & modeling
What defines a customer, a product, or a supplier within an enterprise? These fundamental entities, master data domains, must have well-defined structures to maintain consistency, interoperability, and accuracy across systems. Master data modeling ensures data remains structured, eliminating duplication and preventing fragmentation.
Effective modeling extends beyond database design; it must reflect business logic, workflows, and operational needs. Hierarchies, relationships, and classifications should align with how data is used across finance, sales, supply chain, and customer service functions. A unified master data model enables seamless integration across ERP, CRM, and analytics platforms with a single, trusted version of the truth. Data engineers work on relational data modeling, hierarchical structuring, and semantic standardization to maintain system interoperability.
As enterprises expand, data models must evolve without disrupting operations. Embedding validation rules, approval workflows, and governance protocols directly into the model prevents uncontrolled modifications that lead to inconsistencies. Industry-specific taxonomies, such as GS1 standards in retail or ICD-10 in healthcare, further reinforce standardization through embedding validation rules and business logic into the model.
Data quality management
What happens when decision-makers rely on incomplete or inaccurate data? The consequences range from operational failures to compliance violations and strategic missteps.
Enterprises need proactive mechanisms such as real-time data profiling, anomaly detection, and automated enrichment techniques to prevent errors from propagating across systems. Modern MDM platforms leverage AI and ML to enhance data quality, using probabilistic matching to eliminate duplicates and self-learning algorithms to detect outliers. Techniques like fuzzy logic matching and graph-based entity resolution help organizations refine data relationships, making entities like customers or suppliers accurately linked across disparate datasets.
In the master data management strategy roadmap, real-time monitoring is critical. Automated dashboards and alerting mechanisms provide visibility into data completeness, accuracy, and timeliness. These insights address quality issues before they impact downstream analytics, reporting, or AI-driven initiatives. Without a robust DQM framework, even the most advanced MDM strategies will provide unreliable insights and fragmented operational data.
Data integration
Enterprise data does not reside in a single system, and it is dispersed across ERP, CRM, data lakes, third-party platforms, and legacy databases. Organizations face fragmentation, duplication, and inconsistencies that erode trust in analytics and operational processes without seamless data integration. A structured data strategy harmonizes structured and unstructured data from multiple sources while preserving context and meaning.
Integration must support both batch and real-time data movement. Traditional ETL processes remain essential for structured transformations. Still, modern enterprises also require event-driven architectures that enable real-time data streaming, API-based connectivity, and machine learning-driven data reconciliation.
Standardization is another critical challenge when building a master data management strategy. Inconsistent data formats, naming conventions, and taxonomies create friction in enterprise-wide data consumption. Master data integration must enforce strict schema mapping, metadata management, and interoperability with industry standards such as HL7 in healthcare, SWIFT in finance, and GS1 in retail.
Ownership and accountability
Master data drives core business processes like finance, sales, procurement, and customer engagement, so its accuracy and reliability are mission-critical. Every master data domain must have assigned data stewards responsible for data integrity. Their role extends beyond validation; they enforce governance rules, resolve discrepancies, and maintain compliance standards. A well-structured MDM framework also includes a cross-functional data governance council to align MDM policies with evolving business strategies.
To prevent uncontrolled modifications, the MDM strategy roadmap must involve strict access controls, workflow-driven approval mechanisms, and audit trails. Accountability also means having structured escalation paths for resolving conflicts. When discrepancies arise-such as mismatched financial reports or inconsistent customer data records-there must be a process to correct them before they impact business decisions. Poor accountability leads to unreliable forecasts, compliance risks, and operational slowdowns.
Data lifecycle & archival strategy
Master data evolves, and without lifecycle management, enterprises accumulate redundant, outdated, or non-compliant records, inflating storage costs and degrading data quality. A structured data lifecycle strategy ensures that only relevant, high-value data remains active while obsolete records are archived or purged.
In MDM strategy and roadmap, data lifecycle policies must be defined:
- Data creation and validation: How master data is onboarded, enriched, and verified before entering operational systems.
- Active usage phase: Ensuring data remains accurate and up to date during business operations.
- Retention and archival: When and how inactive data is moved to lower-cost storage while maintaining accessibility for regulatory or historical analysis.
- End-of-life disposal: Securely purging data once it is no longer needed, in compliance with retention policies.
A data lifecycle framework verifies relevant, high-quality master data that remains active. Aging policies dictate when records should be flagged for review, while automated archival mechanisms transition outdated data to low-cost storage solutions or de-identify records for compliance purposes. While inactive records should not consume operational system resources, they must remain retrievable for audits, reporting, and AI-driven analytics.
Explore more about: Data modernization strategy: Rethink enterprise success
Main architecture approaches to master data management
The optimal strategy depends on business complexity, regulatory requirements, and integration needs. Below, we explore three main MDM approaches-centralized, hybrid, and registry-based-detailing how they work and when businesses should consider them.
Centralized MDM
Centralized MDM consolidates all master data into a single repository, creating a unified source across the enterprise. All business units, applications, and processes pull data from this authoritative hub. A dedicated MDM system is the sole repository for ingesting, cleansing, and distributing master data.
When do businesses need centralized architecture MDM?
- If regulatory compliance (GDPR, HIPAA, SOX) requires a single version of the truth
- When organizations need high data accuracy and governance across multiple business units
- If fragmented data silos cause inconsistencies, reconciliation issues, and operational inefficiencies
- When enterprises require centralized control over product, supplier, or customer data
- If real-time data updates and synchronization across all applications are critical
Hybrid MDM
Hybrid MDM balances centralization with flexibility, allowing data to reside in a central hub and across distributed systems. This approach integrates master data across departments while enabling local systems to maintain autonomy where needed. Master data is partially centralized, with core entities-such as customers, products, or suppliers-managed in a central repository, while specific attributes remain decentralized.
When do businesses prefer hybrid MDM?
- If multiple business units require shared data but operate under different processes and regulations
- When an organization has legacy systems that cannot fully integrate into a centralized MDM framework
- If regional or subsidiary-specific data variations need to be maintained alongside standardized global data
- When enterprises require flexibility in adopting MDM while still enforcing governance standards
- If business units must balance autonomy with enterprise-wide data consistency
Registry-based MDM
Registry-based MDM links master data across systems without storing it in a central repository. Instead of consolidating records, it creates a metadata index that maps and synchronizes data across different applications. A registry is a reference index pointing to master records within various source systems. It links distributed records through metadata, unique identifiers, or probabilistic matching algorithms.
When do businesses apply registry-based MDM?
- If enterprises want to enhance data consistency without replacing existing systems
- When system autonomy must be preserved across departments or subsidiaries
- If organizations need a lightweight MDM solution with minimal implementation disruption
- When integrating diverse data sources while avoiding duplication and data movement
- If real-time cross-system data harmonization is needed without full-scale consolidation
Building a master data management strategy in 4 steps
N-iX has seen firsthand that a successful MDM strategy is about aligning data with business priorities, governance, and scalability. Our data engineering and governance expertise helps us to build MDM strategies that align with business objectives, enforce data integrity, and scale with enterprise needs. Let's explore how to build a master data management strategy from our experience.
- First, we define roles and responsibilities to create accountability. We establish data stewardship programs where data owners, custodians, and governance leads are responsible for accuracy, compliance, and alignment with corporate strategy. Then, we assess enterprise data maturity to understand existing challenges. Our team analyzes how data is structured, used, and shared across systems, uncovering inside inconsistencies.
- After that, we work with stakeholders to establish clear data ownership rules, ensuring department accountability. This approach standardizes how critical data is managed and maintained. Next, we establish a governance framework to enforce policies at scale. We design frameworks that align with industry regulations, internal policies, and data security standards.
- As part of the strategy-building process, we guide enterprises in evaluating centralized, hybrid, and registry-based MDM solutions, balancing performance, flexibility, and total cost of ownership. We implement data validation rules, approval workflows, and audit mechanisms that enforce data quality standards.
- Finally, we create a phased implementation roadmap to ensure seamless adoption. We design roadmaps prioritizing high-impact domains first, enabling quick wins while minimizing business disruption.
Challenges of implementing a master data management strategy
Without addressing key challenges, enterprises risk inefficiencies, compliance issues, and data inconsistencies that undermine decision-making. We help organizations navigate these complexities with proven methodologies and enterprise-grade solutions.
Lack of enterprise-wide data ownership
Too often, MDM initiatives struggle because no one truly owns the data. Business units operate in silos, each managing their datasets without centralized accountability. This fragmentation leads to duplicate records, inconsistencies, and conflicts across systems.
Our recommendation: N-iX establishes data ownership structures with clear roles and responsibilities. We also work with enterprises to define data stewardship models.
Inconsistent data governance across business units
Even with an MDM strategy, department governance inconsistencies lead to varying data standards, policies, and validation rules. Enterprises struggle with data quality issues such as unreliable data, regulatory non-compliance, and increased operational risks without alignment.
Our recommendation: We implement a centralized governance framework that enforces standardized policies while allowing flexibility for business-specific requirements. Our experts integrate metadata management, data lineage tracking, and automated policy enforcement to maintain compliance and data integrity.
Integration complexity with legacy systems
Legacy systems often complicate MDM adoption. Outdated ERP, CRM, and data warehouses create different integration challenges, leading to fragmented records, manual reconciliation efforts, and mounting maintenance costs.
Our recommendation: N-iX enables seamless integration by leveraging middleware, APIs, and cloud-native architectures. We modernize data pipelines, implement data virtualization where necessary, and ensure interoperability between legacy and modern systems.
Data quality and standardization issues
Poor data quality-duplicate records, missing attributes, and inconsistent formatting-remains among the most significant roadblocks in data strategy execution. If left unaddressed, it affects analytics accuracy, compliance, and operational efficiency.
Our recommendation: We deploy automated data profiling, cleansing, and enrichment solutions to maintain high-quality, standardized master data. Using machine learning-driven anomaly detection and validation rules, we help enterprises establish data accuracy at scale.
Scalability challenges for growing data volumes
As firms expand, their master data grows exponentially across geographies, products, and customer touchpoints. Traditional MDM systems struggle to scale, leading to performance bottlenecks, increased storage costs, and slow data processing.
Our recommendation: We architect scalable MDM solutions using cloud-native platforms, distributed processing, and high-performance data storage systems. Our engineers optimize indexing, partitioning, and caching mechanisms without compromising performance.
Final thoughts
Implementing MDM without a strategy is like constructing a building without a blueprint: it may stand for a while, but cracks will inevitably appear. Without a clear plan, governance, and alignment with business objectives, even the most advanced MDM solutions will fail to deliver real value.
At N-iX, we've seen what separates short-term fixes from lasting success. N-iX has delivered over 60 data projects and has more than 200 data experts on board. We have worked with leading enterprises and Fortune 500 companies, helping them launch and scale their Data Analytics directions. We have expertise in the most relevant tech stack for implementing Big Data engineering, BI, Data Science, and AI/Machine Learning solutions. If your business is ready to establish a future-proof data foundation, let's start the conversation.
Have a question?
Speak to an expert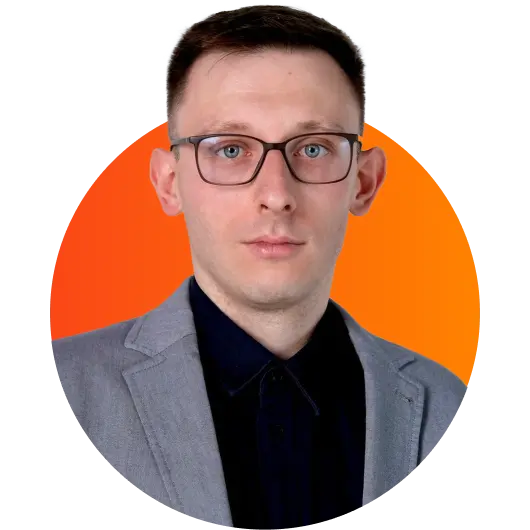