Unplanned downtime costs the manufacturing industry an estimated $50B annually [1]. As a result, manufacturers face recurring equipment downtime, inefficient processes, unpredictable supply chain disruptions, and inconsistent product quality-all of which directly impact profitability and competitiveness.
But the solution is within reach. Manufacturing data analytics integrates several technologies-predictive analytics, AI, big data analytics, IIoT, Machine Learning, and edge computing-into scalable solutions that provide real-time insights. Data analytics services involve collecting data from machines, sensors, production lines, supply chains, and customer feedback and processing and interpreting data generated across all manufacturing stages-from raw material sourcing to finished product delivery. The intended goals of data analytics in manufacturing are to optimize production processes and improve operational efficiency and product quality within the enterprise.
How does data analytics in manufacturing industry work? What are its main use cases? Let's discover together.
Use cases of data analytics in manufacturing
Predictive maintenance
To remain competitive and ensure high final product quality, it is a smart choice for manufacturers to shift from reactive repairs to proactive intervention-data solutions with predictive maintenance. At its core, predictive maintenance application leverages historical data, sensor inputs, and Machine Learning (ML) algorithms to forecast when machinery is likely to fail.
Predictive maintenance can help decrease equipment downtime by up to 50% [2]. It relies on the continuous collection of data from equipment sensors-monitoring parameters (temperature, vibration, and performance metrics.) After ML models analyze the input to identify patterns that indicate wear and tear or imminent failure. Based on these insights, maintenance teams can act ahead of time, avoiding unscheduled interruptions that disrupt production. This is vital for large-scale operations, where even small disruptions can result in significant financial losses.
By quickly identifying potential breakdowns before they happen, business leaders can
- schedule maintenance at optimal times,
- anticipate potential equipment failures,
- avoid costly production interruptions.
One compelling example is our collaboration with Gogo, a global provider of in-flight broadband services, to set up predictive maintenance for their satellite antennas. We enabled the company to predict antenna failures with over 90% accuracy, reducing equipment downtime to 40%. We built a solution using a set of data sources, including environmental factors and equipment logs. This approach allowed the company to predict antenna failures weeks in advance and schedule maintenance during non-operational hours, resulting in substantial savings and operational efficiency. Explore more details from this case study on Big Data analytics for improved maintenance here.
Supply chain monitoring
Supply chain analytics allow companies to monitor and optimize every aspect of their operations-from sourcing raw materials to delivering finished products. This holistic approach reduces the risk of disruptions, ensures optimal inventory levels, and enhances supplier collaboration.
When supply chain data is seamlessly integrated with manufacturing operations, manufacturers can proactively manage raw material shortages, optimize production schedules, and improve order fulfillment. Supply chain analytics enable manufacturing companies to visualize their entire supply chain in real time, helping them manage lead times and forecast demand more accurately. With advanced analytics, businesses can predict how external factors-weather, geopolitical events, or supplier disruptions-might affect their operations, allowing them to adjust procurement and production schedules accordingly.
For instance, N-iX has worked with a Fortune 500 industrial supply company to optimize its supply chain using a scalable cloud-based big data platform. Migrating the client's on-premises data to the cloud, N-iX helped integrate over 100 data sources, including supplier data, inventory levels, and demand forecasts. This unified platform allowed the client to predict inventory-related costs, improve demand forecasting accuracy, and streamline procurement processes. The result was a significant reduction in operational costs and improved delivery timelines.
Read more about: Scalable big data analytics platform for leading industrial supply company
Warehouse management
Inventory management directly affects operational efficiency and cost control. Data analytics can optimize inventory management and provide real-time insights into stock levels, demand patterns, and supplier performance. As a result, manufacturers can forecast demand more accurately, ensuring they have the right amount of inventory at the right time.
In addition to optimizing stock levels, analytics can help manufacturing companies identify inefficiencies in their supply chain, such as delays in supplier deliveries or excessive lead times. With this data, they can adjust inventory strategies, such as implementing just-in-time inventory practices or developing contingency plans for supply chain disruptions. Effective inventory management lets business owners have suitable raw materials and finished goods to match production and customer demands without overstocking or running into shortages. Manufacturing data analytics provides insights into demand patterns, allowing for more accurate inventory forecasting and reducing stockouts and excess inventory.
Quality control
Data analytics can identify defects in real time and improve quality control processes. Manufacturers can detect anomalies early and make immediate adjustments after collecting and analyzing data from various points in the production process-such as sensors on production lines, inspection cameras, or environmental factors. Advanced analytics can detect deviations from quality standards, identify root causes, and suggest process adjustments to minimize defects.
Leading-edge analytics technologies, such as Machine Learning and Computer Vision, are used to predict defects even before they occur. By correlating variables like temperature, pressure, and material quality, businesses can determine the root cause of defects and implement corrective actions to prevent future occurrences. This results in higher production accuracy and fewer defective products reaching customers, directly improving customer satisfaction.
Energy and resource optimization
Energy costs represent a significant portion of manufacturing expenses, and sustainability is increasingly becoming a top priority for manufacturers. Data analytics solutions give the insights they need to optimize energy usage, reduce emissions, and meet sustainability goals.
Advanced analytics tools allow for tracking energy consumption patterns across their facilities and identifying inefficiencies. When integrating data from production systems and energy meters, manufacturing businesses can optimize resource usage-reducing electricity consumption during non-peak hours or minimizing waste in the production process. So, data analytics for manufacturing can help identify inefficiency, such as excessive energy consumption.
Read more: Big Data in manufacturing made simple: Trends, tips, success stories
After exploring the use cases, it's clear that data-driven insights can transform operational efficiency across various areas, from energy optimization to quality control. However, manufacturers must integrate advanced data processing and analytics into their existing infrastructure to maximize the full potential of data analytics in manufacturing industry.
Key integrations for manufacturing data analytics
The critical integrations enable businesses to collect, process, and analyze data in real time, facilitating more intelligent decision-making, optimizing resources, and improving overall performance. Here are the key integrations essential for effective manufacturing data analytics:
Data storage & processing integration
Data storage and processing systems form the foundation for effective analytics in manufacturing. Key components include:
- Automated data ingestion: Integrating IoT devices, production systems, and inventory reports allows continuous data collection. In turn, this enables to gather structured and unstructured data, such as sensor readings from equipment or sales transactions.
- Batch and real-time data processing: Integration with batch and real-time data processing capabilities supports operational flexibility. For instance, historical data can be processed in batches for long-term analysis, while real-time data ensures instant insights for immediate action.
- Data aggregation: With integrations that consolidate data from various departments, manufacturers can generate a unified source of truth. This aggregated data ensures that all stakeholders-production managers and executives-work with consistent, reliable information.
Data analysis & reporting integration
Manufacturing analysis systems rely on integrated tools to enhance data reporting and visualization:
- Online analytical processing: Businesses can perform complex multidimensional analysis. Such work includes slicing data, such as investigating defective products by shift or production line, to uncover trends and optimize performance.
- KPI calculation: Integrated data systems automatically calculate Key Performance Indicators (KPIs) across operations, tracking metrics like production throughput, downtime, and OEE.
- AI-powered predictive and prescriptive analytics: Integrating ML models into manufacturing systems facilitates predictive maintenance and operational optimization. These models review historical and real-time data to forecast future trends and suggest improvements.
Enhance manufacturing efficiency with actionable BI insights – explore the guide!
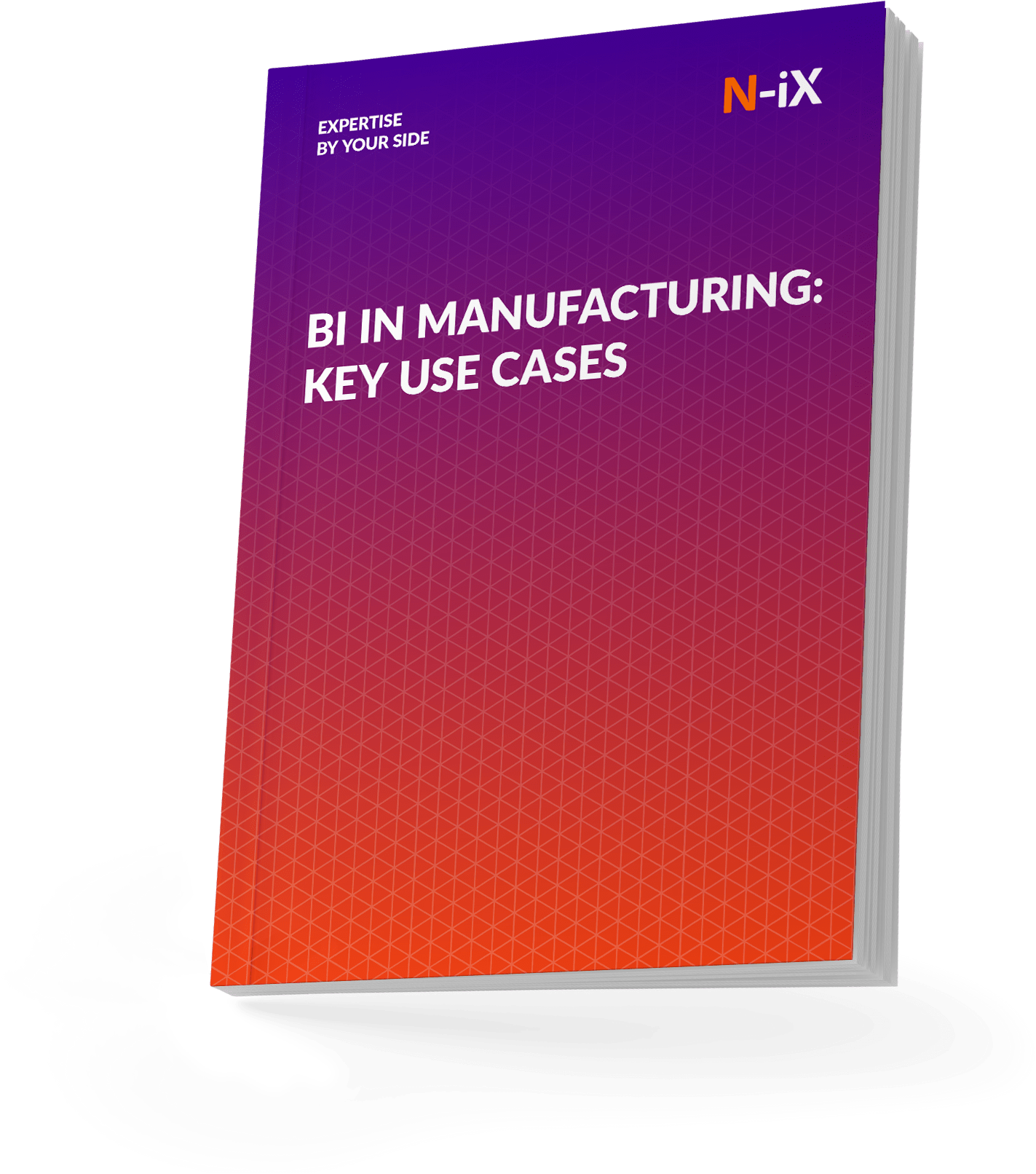
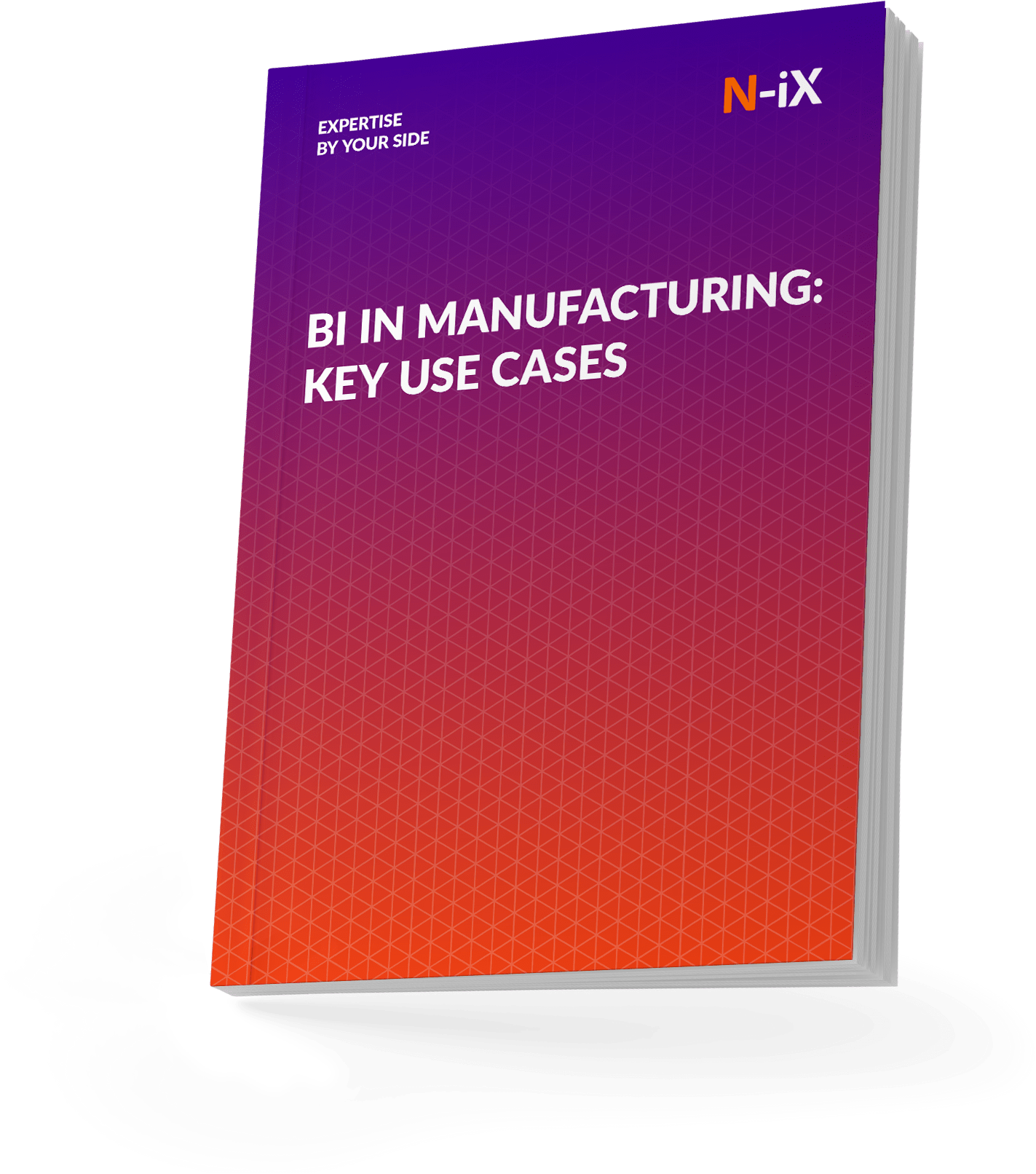
Success!
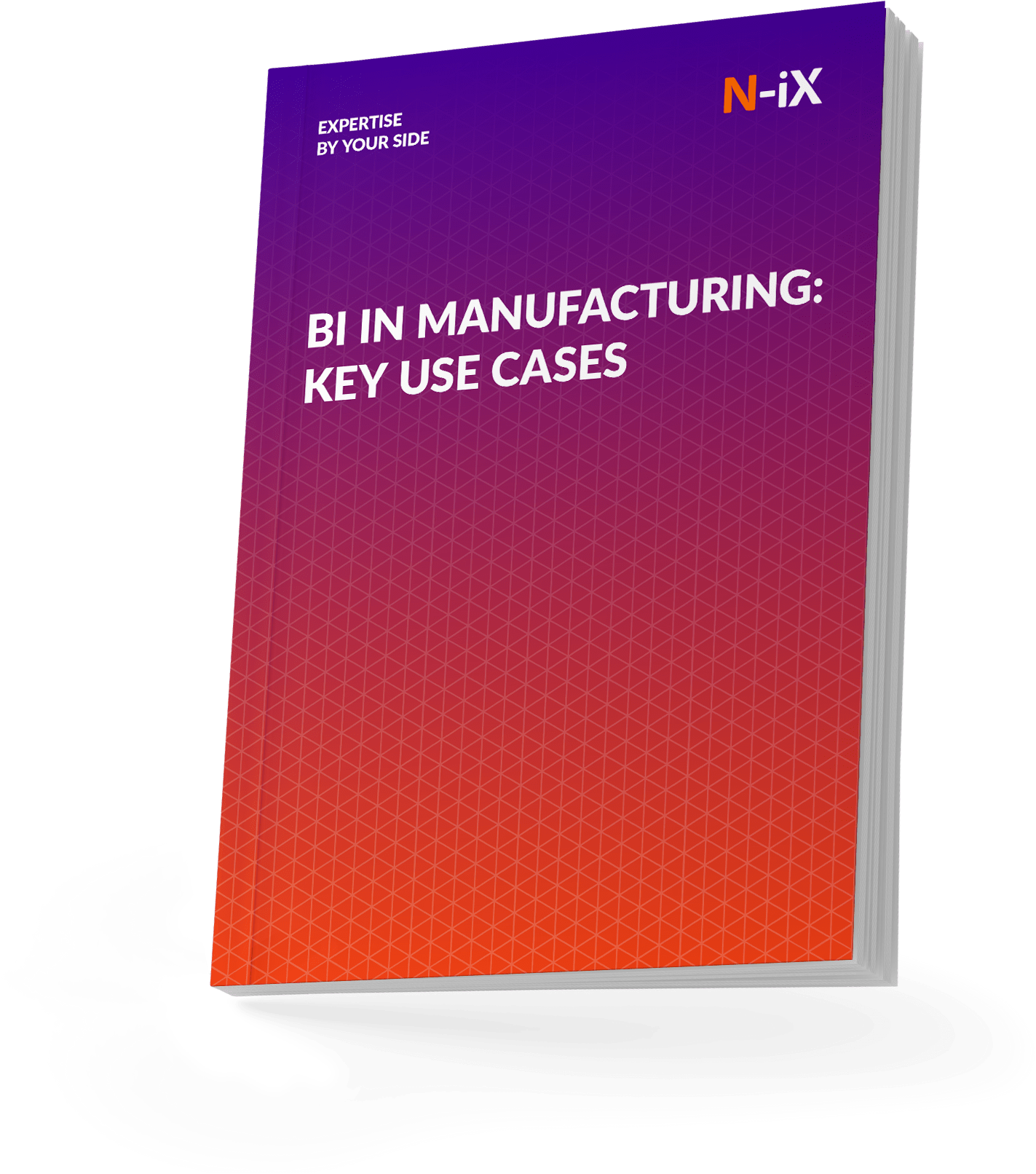
Cost analytics integration
Cost analytics integration helps companies monitor and optimize financial performance:
- Automated cost calculations: Systems that automatically factor in raw materials, labor, and overhead can integrate with manufacturing data to calculate costs dynamically.
- AI-based cost-saving insights: Integration with AI tools allows manufacturers to identify patterns in energy consumption or resource use, enabling targeted cost-reduction strategies.
Asset analytics integration
Asset management systems integrated with analytics platforms ensure optimal equipment performance:
- Real-time machine monitoring: Integrating IoT sensors and PLCs, manufacturers gain real-time insights into machine conditions, availability, and resource utilization. This data can trigger alerts when performance issues are detected, preventing unplanned downtime.
- Physics-based modeling: Integration with advanced modeling tools allows businesses to simulate machine behavior, determining the most efficient operating conditions and optimizing OEE.
Production analytics integration
Production analytics benefit from integrated data sources across multiple stages of the manufacturing process:
- Optimized production scheduling: Combining real-time data on machine availability, employee schedules, and material supply enables production systems to optimize workflows and prevent bottlenecks.
- Bottleneck identification: Integrated analytics platforms can analyze production flows to pinpoint and address bottlenecks that cause inefficiencies or slowdowns.
- What-if scenarios: ML models integrated into production systems allow manufacturing companies to simulate different production scenarios.
Supply chain analytics integration
Integrating supply chain data enables businesses to manage their logistics and procurement more effectively:
- Supplier performance analysis: With integrated data from procurement, businesses can track KPIs such as lead times, defect rates, and supplier reliability to optimize sourcing strategies.
- Demand forecasting: ML models integrated into supply chain systems predict future demand. From this integration, businesses can adjust production schedules and inventory levels accordingly.
Sales analytics integration
Integrating sales data into manufacturing systems sets up a direct relationship between market demand and production:
- Sales KPI tracking: Integrated systems track sales performance metrics, checking the correlation between sales goals and production capacity.
- AI sales forecasting: AI algorithms integrated into sales analytics provide precise demand forecasts. For large enterprises, operational teams gains more efficient production scheduling and inventory management.
Customer analytics integration
Customer analytics systems integrated into manufacturing operations provide valuable insights into market behavior:
- B2B and B2C customer segmentation: Manufacturers can segment customers based on behavior, demographics, and preferences. This process will impact targeted marketing and sales strategies.
- Product feedback analysis: Integrated customer feedback systems analyze warranty claims and reviews.
Best practices to adopt for manufacturing data analytics
To leverage the advantage of data analytics for manufacturing, it's essential to follow a set of proven best practices. Based on our expertise, here are the key practices we recommend for enterprises:
Starting small
We always advise starting with small, targeted pilot projects rather than trying to implement data analytics across the entire operation at once. This approach allows for focusing on areas where analytics can quickly demonstrate value, such as predictive maintenance on a particular production line or optimizing inventory management. Starting small enables teams to learn, iterate, and improve before scaling the solution organization-wide.
Scaling gradually
Once initial projects have proven successful, step by step scaling is the pathway to long-term success. We suggest developing a strategic roadmap for scaling. Our data engineering teams keep in mind the high-impact and prioritized areas like production optimization, supply chain management, and energy efficiency. Scaling should be done in stages to ensure that each expansion is built on solid insights and proven methods.
Align data analytics with business objectives
We agree that the implementation of manufacturing data analytics should be in line with the business's strategic goals. Every single data-driven initiative we implement is designed to support key performance indicators. Our experience across diverse industries has shown that the return on investment becomes clear when analytics are tied to measurable outcomes-such as increased uptime, reduced downtime, or higher product yield.
Ensure data quality
We've worked with manufacturing companies to implement data governance strategies that improved data quality and ensured regulatory compliance-particularly in sectors like automotive. We establish centralized data governance protocols and enable our clients to maintain complete control over their data assets while scaling their analytics operations.
Fostering a data-driven culture
At N-iX, we believe that true transformation happens when data becomes an integral part of the organization's decision-making process. From our experience, we can define that equipping teams across all levels-engineers, operators, managers-with the right data tools and training creates a more agile and responsive manufacturing environment. We provide tailored training programs to ensure staff can confidently interpret and apply data insights to their day-to-day operations.
Final thoughts
Approximately 75% of business data that requires data analytics goes unused [3]. It's no longer enough to collect data simply-manufacturers must leverage advanced analytics to unlock actionable insights that reduce costs and improve product quality. From predictive maintenance to supply chain optimization, integrating data analytics helps enterprises operate smarter, adapt faster, and innovate continuously.
A company adopting a data-driven approach can optimize everything from predictive maintenance to real-time supply chain visibility and energy efficiency. Manufacturers that take a proactive stance on data analytics are positioning themselves for future success in an increasingly digital world.
Don't wait to experience the full potential of data analytics for manufacturing. Partner with N-iX to take your manufacturing operations to the next level.
Reference
- Predictive maintenance and the smart factory - Delloite
- Predictive Maintenance - Delloite
- Data-Driven Business Agility Without Compromise - Oracle
Have a question?
Speak to an expert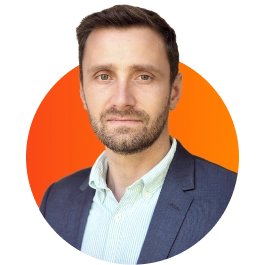