Machine Learning in healthcare is transforming the industry and helps to innovate disease discovery and treatment. Since communities expect their healthcare facilities to provide the best services—from medical treatments to surgical procedures to foreseeing future diseases, etc.—Machine Learning helps healthcare institutions live up to expectations. How is Machine Learning used in healthcare? What are the most promising Machine Learning use cases in healthcare? Let’s discover.
Most common ML use cases in healthcare
According to PwC, the areas with the most significant potential for the use of Machine Learning in healthcare are ML-based medical diagnosis, early identification of potential pandemics, tracking the incidence of the disease, and imaging diagnostics (radiology, pathology). Let’s take a look at these and other use cases in more detail.
1. Discovering and manufacturing drugs
ML in healthcare significantly accelerates and enhances various stages of drug development. With ML algorithms, the drug discovery process becomes more efficient, cost-effective, and capable of producing safer and more effective remedies. This approach accelerates the time to market for new drugs and enhances the overall success rate of drug development projects. Insider Intelligence states that the healthcare sector can save as much as 70% of drug discovery costs with the applications of ML in healthcare. Here is how ML can be used in drug discovery and manufacturing.
2. Disease identification and diagnosis
Another way how can Machine Learning be used in healthcare is the improvement of disease identification and diagnosis. By improving detection accuracy, enabling early intervention, and personalizing treatments, ML ultimately leads to better patient outcomes and more efficient healthcare delivery. For instance, Machine Learning algorithms in healthcare are used for image recognition and analysis of medical images such as X-rays, MRIs, CT scans, and ultrasounds. These models can accurately detect abnormalities like tumors, fractures, and lesions. As a result, they can identify early signs of diseases, such as small nodules in lung cancer or early-stage diabetic retinopathy, which human eyes might miss.
3. Better medical imaging
ML algorithms can process and analyze medical images much faster than human radiologists, leading to quicker diagnoses and reducing patient wait times. Moreover, Machine Learning can simultaneously handle large volumes of images, making it ideal for large-scale screening programs, such as mammography for breast cancer detection.
Apart from that, ML can be used for image enhancement and reconstruction. For instance, with ML, it is possible to reduce noise in medical images, improving clarity and detail without increasing radiation exposure, particularly in low-dose CT scans. ML algorithms can also enhance the resolution of medical images, providing more precise and more detailed views, which is especially useful in MRI and ultrasound imaging.
4. Treatment personalization
Nowadays, there’s a need for medicines to work well with individual patients. Instead of a one-size-fits-all approach, the healthcare industry wants to make medicines more personalized. As a result, it created room for the development of Machine Learning in the medical field.
In the US, the personalized medicine market was valued at $538.93B in 2022 and is projected to grow at a CAGR of 7.2% by 2030. The growth of the personalized medicine market is driven by the increasing demand for innovative drug discovery to address the rising incidence of cancers and other diseases worldwide. Additionally, numerous collaborations among researchers and industry stakeholders are expected to positively influence market expansion.
As projected, personal medical data of individual patients will be possible with ML applications and algorithms, meaning that health issues can be detected and assessed easier. And, based on the individual’s medical history, potential risks can be predicted and prevented.
5. Fraud detection
According to the Global Healthcare Anti-Fraud Network, approximately $260B is lost globally to health insurance fraud each year, which is equivalent to 6% of global healthcare spending. By analyzing vast amounts of data, Machine Learning algorithms can identify patterns and anomalies that may indicate fraudulent activities, thereby saving the industry billions of dollars and ensuring the integrity of healthcare systems.
Similarly, Machine Learning plays a pivotal role in identifying prescription fraud. By analyzing prescription patterns, ML algorithms can flag suspicious activities such as overprescribing, doctor shopping (where a patient visits multiple doctors to obtain more prescriptions), and unusual combinations of medications. For example, if a patient is prescribed multiple opioids by different doctors within a short period, the system can alert authorities to potential abuse or fraud. These insights help healthcare providers and pharmacies ensure that medications are prescribed and dispensed appropriately, thereby protecting patients and reducing the risk of drug abuse.
Read more about generative AI in healthcare
6. Smarter health records
Since there’s a rise in the need for personalized medicines and treatments, there’s also a growing need for smarter health records. With ML, healthcare facilities can keep up with health records without having to waste time keeping them up-to-date. Since ML strives to save people time, money, and effort, its market size has been increasing over the years in the healthcare industry.
Grand View Research projects that the market for electronic health records in ambulatory and hospital use will grow in value by 2027. Health records need to be smarter, too, to provide better diagnoses, clinical treatment suggestions, etc.
7. Smarter clinical trials and research
Before ML had risen to where it is today, clinical trials and research were done through time and money. However, with ML, trials were done in small circles because the technology and app options were limited at the time. Today, ML will help clinical trials and research in the following ways:
- To look for potential clinical trial candidates;
- To monitor candidates through clinical trials;
- To access candidates’ medical history;
- To select good testing samples;
- To avoid data-based errors, etc.
8. Robots in surgical procedures
With ML come innovations such as robotic surgery. Robots in surgical procedures have made their way into the healthcare industry. ResearchGate suggests that robotic surgical innovations have taken part in various surgeries, including general surgery, urology, and pediatric surgery.
9. Improved radiotherapy
Radiotherapy is another part of healthcare where ML will thrive. With medical imaging and analysis at the forefront of radiotherapy, there’s a need to ensure that they’re accurate, and that patients are safe when undergoing treatment. That’s why ML not only wants to ensure better radiotherapy, but also be able to detect things like lesions based on data that’s already at hand.
10. Remote monitoring and wearable devices
Wearable devices can collect data on vital signs, physical activity, and other health metrics. Meanwhile, ML algorithms analyze this data to detect anomalies and provide early warnings of conditions like arrhythmias, sleep apnea, and hypertension. What’s more, continuous monitoring helps in managing chronic diseases such as diabetes and heart disease by providing real-time feedback and personalized recommendations.
Conclusion
ML is transforming the industry by bringing about the benefits of Machine Learning in healthcare, such as enabling innovative disease discovery, personalized treatments, and efficient clinical processes. From drug discovery and medical imaging to personalized medicine and fraud detection, the applications of ML are vast and impactful. As technology continues to advance, the role of ML in healthcare will only grow, leading to improved patient outcomes and more efficient healthcare delivery.
Have a question?
Speak to an expert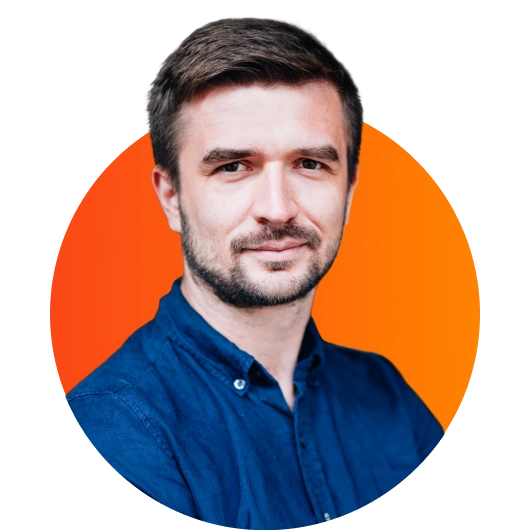