According to a McKinsey report, 63% of businesses increased their AI investments in 2022, with generative AI emerging as a critical focus area. Despite this rise, only 23% of companies successfully deploy AI at scale.
The problem isn't the technology itself-it's the approach. Many enterprises jump into generative AI implementation without clearly understanding how to tailor it to their specific needs. As a result, they face issues like poor scalability, misaligned outcomes, and incompatibility with existing processes. Generic AI tools often fall short, leading to underutilization and wasted resources because each business challenge-whether it's optimizing supply chains, enhancing customer engagement, or driving product innovation-requires a customized generative AI solution.
Rather than relying on a general-purpose tool, enterprises must focus on a well-thought-out plan covering everything from initial discovery and data assessment to full-scale deployment and ongoing optimization. Let's take a closer look at the process of implementing generative AI consulting solutions in a structured way. In this guide, we will walk through key strategies, best practices, and actionable steps to help you navigate generative AI's complexities.
Before implementation: key considerations
Generative AI implementation involves integrating advanced AI models into business workflows. The biggest strength is the ability to drive innovation, optimize operations, and provide personalized experiences at scale.
Transitioning from strategy to operational success involves overcoming challenges and aligning AI initiatives with broader business objectives. Below are the core needs and pain points enterprises face when planning and deploying generative AI solutions:
- How do we ensure generative AI initiatives align with strategic priorities and not merely technology-driven experiments? Defining clear business objectives and ensuring that each AI project is designed to support these goals rather than adopting AI for its novelty is required for enterprises.
- What are the key areas where generative AI can deliver tangible business value, and how do we prioritize them to ensure measurable ROI? Enterprises should evaluate use cases based on potential revenue impact, cost reduction, or efficiency improvements and prioritize those with the highest ROI potential.
- How can generative AI seamlessly integrate into existing IT infrastructure without disrupting current operations or creating technical debt? Proper planning and alignment between IT and business teams are essential to design integration strategies that complement, rather than conflict with, current systems.
- What are the hidden costs and complexities associated with generative AI implementation, and how can we ensure the project remains on budget and within scope? Hidden costs can include data preparation, model maintenance, and compliance, so it's vital to have a comprehensive budgeting plan that accounts for these variables.
- What KPIs should be defined upfront to evaluate generative AI's impact on business outcomes, and how do we quantify the value created through these initiatives? KPIs should directly link to business goals, such as increased sales, reduced churn, or improved customer satisfaction, and track these against baseline metrics.
- Who are the primary stakeholders and decision-makers for the project? Identify critical stakeholders early, such as C-level executives, department heads, and technical leads, to ensure alignment throughout the project.
Addressing key challenges from the start is crucial to take advantage of generative AI for long-term business impact. The path to successful implementation involves two main focus areas:
- Establishing a solid data foundation to provide reliable inputs and support AI infrastructure.
- Executing generative AI implementation framework in alignment with real-world business needs.
We'll detail these two essential components below, covering what's needed and how to approach them effectively.
The role of data strategy in generative AI implementation
Data is the core foundation that determines AI models' effectiveness, reliability, and scalability. A flawed data strategy can lead to poor performance, compliance issues, and significant operational bottlenecks. Without a single, cohesive data strategy, enterprises may face critical risks throughout the AI lifecycle:
- Low-quality or unstructured data can lead to inaccurate predictions and flawed AI outputs. If the underlying data is inconsistent or biased, even the most advanced models will produce skewed results that undermine business value.
- When data is siloed across multiple systems or departments, achieving the unified view required for generative AI to perform optimally becomes challenging. A lack of real-time data integration means that models are trained on outdated or incomplete data, reducing their effectiveness in dynamic business environments.
- If data architecture and governance are not designed to scale, adding new use cases or expanding AI capabilities can become a logistical nightmare. As a result, it limits the AI's potential and causes exponential increases in costs and time for maintenance.
- Generative AI often involves sensitive data, and a weak data strategy can expose the organization to compliance violations and security breaches. Without strong data governance policies, companies risk mishandling personal or regulated data.
- Managing data quality, ensuring version control, and maintaining data consistency are just a few operational challenges that arise without a solid data management strategy. This complexity makes it harder to monitor model performance, track issues, and ensure reliable outputs over time.
To address these challenges, a comprehensive data strategy for generative AI should include the following key components:
- Data governance framework that includes clear policies for data usage, access controls, and compliance with regulations such as GDPR or CCPA.
- Data quality and validation, automated tools, and manual processes that clean and standardize data, ensuring that it meets the required standards for model training.
- Scalable data architecture that supports real-time data ingestion, processing, and storage to ensure that AI models are equipped with up-to-date and accurate information.
- Metadata management that provides contextual information, ensuring that data sources, version histories, and processing methods are transparent and well-documented.
These elements form the basis of a reliable generative AI implementation strategy, supporting every phase, from training to real-world deployment.
Generative AI implementation framework: a step-by-step guide
Implementing generative AI in an enterprise setting demands a clear strategy, meticulous planning, and precise execution to translate AI capabilities into tangible business outcomes. At N-iX, we have developed a structured implementation framework that guides organizations through every step of the generative AI journey, from initial planning to ongoing optimization. Below, we outline our comprehensive roadmap.
1. Setting specific AI goals
The first phase focuses on understanding the unique requirements and pain points that generative AI can significantly impact. Through a series of up to six targeted workshops, our team collaborates with business leaders, technical stakeholders, and domain experts to dive deeply into the enterprise's current state and future aspirations. We identify high-value use cases that align with the organization's strategic goals and ensure the company's readiness for generative AI adoption.
During these sessions, we define the overarching vision for generative AI and prioritize which initiatives to pursue based on potential ROI, complexity, and feasibility. For example, generative AI could streamline customer support operations by automating routine inquiries, enhancing marketing through personalized content, or accelerating product development with AI-assisted designs.
This phase establishes a shared understanding across departments, setting clear expectations and aligning stakeholders around success criteria. We conduct generative AI readiness assessments and education sessions to confirm that technical teams and business units are prepared for what's next.
2. Testing key use cases
Once the initial groundwork is laid, we move into the rapid prototyping phase. The focus here is on validating the identified use cases through real-world experimentation. This stage typically lasts 2 to 8 weeks and involves building quick prototypes and conducting iterative testing to gather feedback and refine the solution. The goal is to test the feasibility of the generative AI model in a controlled environment and fine-tune it based on live input before scaling up.
Rapid prototyping minimizes risks and helps identify potential pitfalls early on. Our generative AI engineers, project managers, software engineers, and UX/UI designers work closely with clients to ensure the prototypes function correctly and deliver meaningful insights that guide further development. We focus on validating core functionalities, providing ease of integration with existing systems, and evaluating the overall impact on business processes. This iterative approach helps make the solution robust, scalable, and aligned with business needs.
Businesses can identify which AI use cases are most feasible and impactful by addressing these considerations. Here are some key questions to evaluate the suitability of each use case:
- Does the use case support core business objectives, such as enhancing customer experiences, increasing operational efficiency, or enabling product innovation?
- Does the organization have the necessary data to support this use case? If not, what data acquisition strategies are needed?
- What are the technical and operational challenges involved? Does the company have the necessary resources, or will significant upskilling and investments be required?
- What is the potential business value regarding revenue growth, cost savings, or market differentiation?
The success story of generative AI implementation from N-iX: We helped a leading brokerage firm streamline employee operations by developing a custom generative AI-powered web portal. This solution automated routine tasks such as writing emails, generating JIRA tickets, and querying internal policies, significantly improving efficiency. By integrating robust multi-tenant data storage and LLMs for rapid information retrieval, employees are able to access company knowledge seamlessly. Our solution also ensured data security through encrypted requests and network protection, reducing infrastructure expenses by optimizing GPU usage. Explore more details on integrating custom generative AI in the case study.
3. Designing strategic solution
With validated prototypes in place, the next step is to design a comprehensive solution that can be scaled across the organization. This phase, which usually takes 2 to 4 weeks, involves defining the architecture vision, establishing a strategic roadmap, and ensuring that all technology choices align with long-term business objectives. At this stage, we consider factors like infrastructure scalability, integration requirements, and the need for ongoing support.
Our solution architects, business analysts, and generative AI engineers collaborate to create a detailed blueprint that outlines how the generative AI solution will be implemented, scaled, and maintained. The process includes choosing the right technology stack-whether to use pre-trained models or custom-built models tailored to niche business needs. For text-based use cases, models like GPT-4, Claude, Mistral and LLaMA may be suitable, while DALL·E and StyleGAN are preferred for visual content generation. For tasks requiring deeper natural language comprehension, BERT or RoBERTa are highly effective. In cases where more domain-specific or highly customized functionalities are needed, building specialized models from scratch or fine-tuning existing ones can lead to better results and greater alignment with business goals.
The success story of generative AI implementation from N-iX: We partnered with a leading P2P software review platform to implement an AI-powered Pros and Cons feature. Using GPT-4 and topic modeling techniques, we helped categorize and summarize user reviews, highlighting key insights. Our solution optimized prompt structures to reduce API costs and improve SEO rankings by generating precise review keywords. Continue reading this case study: Improving user experience of a P2P review platform with Machine Learning and NLP
To ensure seamless adoption, we assess options based on the industry-specific regulatory landscape, such as HIPAA compliance in healthcare, GDPR for data privacy, and PCI DSS for payment systems. We also evaluate the company's technical capabilities, identify gaps, and propose solutions. The outcome is a well-defined architecture and a phased implementation plan.
4. Implementing generative AI into business operations
At N-iX, the implementation of generative AI solutions is a comprehensive process designed to translate advanced AI models into practical business tools that deliver value from day one.
The process typically begins with a solution development phase, where the initial validated prototype is transformed into a fully functional AI system. This stage, which can span from 2 to 4 months, depending on the complexity of the solution, is focused on ensuring that the generative AI models are fully operational, reliable, and ready to deliver business value from day one.
This phase involves refining the generative models and ensuring they integrate seamlessly into the organization's infrastructure. During this stage, N-iX engineers and solution architects work meticulously to build robust data pipelines, define architecture specifications, and create a resilient structure capable of supporting complex AI functionalities. Each element is crafted with long-term goals in mind, guaranteeing flexibility and scalability for enterprises.
Integration and testing are the next critical steps. Here, the focus shifts to ensuring that the AI system operates harmoniously with the organization's existing tools, workflows, and databases. Next, we connect APIs, configure data flows, and align the model outputs with the company's operational standards. The N-iX team performs comprehensive testing, including stress and performance evaluations, to confirm that the solution meets all requirements. During this time, any potential bottlenecks or incompatibilities are addressed proactively to minimize future disruptions.
An equally vital component of N-iX's implementation strategy is team enablement and training. The successful adoption of generative AI hinges not just on technical execution but also on empowering the people who will interact with it. N-iX conducts in-depth training sessions for both technical and non-technical stakeholders, explaining the AI's capabilities, usage scenarios, and best practices. This training and extensive documentation ensure that internal teams can effectively leverage the new solution, reducing friction during the handover phase.
Beyond the initial deployment, quality assurance and ongoing support are integral to the N-iX approach. A dedicated team of QA specialists continuously monitors the solution, fine-tuning components and providing swift responses to emerging issues. This support framework helps maintain high-performance levels, addresses unforeseen complications, and adapts solutions to evolving business needs.
5. Refining and enhancing AI performance
The journey doesn't end once the generative AI solution is deployed. Continuous optimization is a crucial part of N-iX's generative AI implementation service. This phase involves monitoring the system's performance, gathering user feedback, and improving the solution's effectiveness. We implement robust monitoring and reporting systems to track key metrics, such as accuracy, processing speed, and user engagement, which are essential for fine-tuning the models. As business needs evolve and new insights are gained, we adapt the AI models to maintain relevance and maximize their contribution to the organization's goals.
Our optimization team, which includes generative AI engineers, support engineers, and project managers, controls that the AI solution continues to deliver value by proactively addressing any issues and implementing enhancements based on real-world data. We employ prompt engineering, fine-tuning, and retraining models to refine outputs and keep the technology ahead of market shifts. The ultimate goal is to maintain a high level of performance and the solution's scalability as the organization grows. This phase often involves:
- Adapting data pipelines to handle larger volumes of information;
- Extending integration to other business systems and processes;
- Adding new functionalities based on user needs and feedback;
- Enhancing security protocols to protect sensitive data;
- Implementing tools for continuous performance tracking and optimization.
Addressing the challenges of generative AI implementation
Success in implementing requires a deep understanding of potential roadblocks and a strategic approach to mitigate risks while maximizing business value. At N-iX, we help enterprises overcome these obstacles.
Use case identification
One of the key challenges in adopting generative AI is accurately identifying which use cases will drive the most impact and align with strategic business objectives. Many organizations rush into deploying AI without thoroughly evaluating its long-term benefits, or they gravitate toward trendy applications like chatbots while missing out on potentially transformative but less obvious use cases. Another area for improvement is limiting the scope to fit off-the-shelf tools instead of considering custom-built solutions that address specific business needs better.
N-iX solution: We conduct in-depth workshops and stakeholder interviews to uncover areas where generative AI can provide tangible value, focusing on high-impact use cases that may take time to be apparent. Our process involves a rigorous evaluation to avoid common pitfalls, such as selecting use cases based solely on perceived visibility rather than potential business outcomes. We assess each use case against a balanced criteria scorecard-including scalability, complexity, and alignment with enterprise goals-so that AI investments are rooted in a clear understanding of risk and reward.
The success story of generative AI implementation from N-iX: We collaborated with a global software engineering firm to implement a generative AI-powered sales assistant to address inefficiencies in responding to customer inquiries. The solution was designed using OpenAI's API and a custom-built corporate knowledge base capable of parsing and indexing a vast repository of internal documents, case studies, and services. Each customer question is matched against this knowledge base using advanced Natural Language Processing models. Our approach reduced response times, improved user engagement, and increased lead conversion.
Read more highlights of this success story: Improving user experience and client engagement in IT with generative AI
Organizational readiness
Many enterprises need to pay more attention to the groundwork required to implement generative AI successfully. Data preparation, infrastructure readiness, and engaging end-users early are often overlooked, resulting in stalled projects or poor adoption rates. If staff are not adequately trained and the current IT ecosystem cannot support AI models, the chances of success decrease rapidly. Moreover, failing to engage end-users during the initial stages leads to solutions that may be technically sound but lack practical usability.
N-iX solution: We ensure organizational readiness through a multi-step approach that addresses technical, operational, and cultural factors. We start by assessing the enterprise's data landscape to identify quality, availability, and governance gaps. Next, we involve cross-functional teams-from business units to IT and compliance-early in the planning stage to foster a culture of collaboration and shared ownership. We also provide comprehensive training programs to upskill teams to prepare them for new workflows and technologies.
Our readiness assessment goes beyond technology. During generative AI implementation service, we focus on aligning internal capabilities with AI requirements, ensuring that the people and the infrastructure are equipped to handle the demands of generative AI. It requires evaluating cloud readiness, data processing capabilities, and compliance frameworks to create a robust foundation for AI implementation.
Lean AI development
Overambitious projects, rigid requirements, and unclear success criteria frequently contribute to failed AI implementations. Too often, enterprises aim for fully matured AI solutions from the outset, leading to extended timelines, escalating costs, and eventual project abandonment. Another pitfall is the inability to adapt as technology advances, leaving AI initiatives disconnected from market realities.
N-iX solution: We prioritize an iterative development approach that allows for quick validation and flexibility. Starting with small, focused PoCs, we gather continuous feedback to refine requirements as the project evolves. Our agile development practices focus on delivering immediate value while adapting to new insights and technological advances, ensuring that the solution remains relevant and impactful.
Scaling AI
One of the biggest challenges enterprises face is transitioning from small-scale pilots to full-scale AI implementations. Many organizations mistakenly treat PoCs as production-ready solutions without proper validation, leading to failures during scaling. Additionally, a lack of cohesive strategy and adequate resource allocation can help scalability.
N-iX solution: We approach scaling with a well-defined roadmap that transitions AI models from pilot phases to enterprise-wide adoption. Our team conducts detailed scalability assessments to identify potential bottlenecks in infrastructure, computational resources, and data pipelines. We thoroughly validate service quotas, system limits, and integration points before expanding AI deployment.
Our scaling strategy involves building modular AI solutions that adapt to varying business needs across different departments and regions. This flexibility reduces dependency on a one-size-fits-all model and allows customization based on localized needs. We also set up monitoring frameworks and automated scaling mechanisms to ensure that AI performance remains optimal as demand increases.
Responsible generative AI
Using generative AI brings many ethical considerations, including potential misuse, data privacy issues, and biases in AI models. Irresponsible data handling can lead to breaches and compliance risks, while poorly governed AI models may produce outputs that conflict with human values. Moreover, generative models are vulnerable to adversarial attacks without robust security measures, which can undermine trust and lead to reputational damage.
N-iX solution: We prioritize building responsible AI systems by embedding ethical guidelines, security protocols, and bias mitigation strategies from the start. Our process includes regular audits, fairness assessments, and explainability features to ensure that AI models operate within ethical boundaries. We also implement data governance frameworks to protect sensitive information and comply with global standards.
Best practices for adopting generative AI smoothly
Deploying generative AI solutions in an enterprise requires a thoughtful, phased approach to ensure alignment with business goals and smooth integration into existing workflows. Based on our extensive experience, we recommend the following best practices to streamline adoption and maximize the potential of generative AI:
- Setting a holistic generative AI strategy: Our approach begins with deeply understanding your business goals and creating a comprehensive roadmap that aligns technology choices with strategic priorities. This strategy should consider long-term scalability, governance, and potential ROI.
- Ensuring robust data protection and compliance: We suggest prioritizing data security and adhering to industry-specific compliance standards. Implementing robust data governance frameworks is essential to build trust, mitigate risks, and maintain the integrity of your AI solutions. Our experts guide organizations through these complexities to ensure regulatory alignment.
- Adopting a gradual rollout approach: Avoid the urge to go all-in from the start. We recommend a gradual, phased rollout to monitor outcomes closely, gather feedback, and make necessary adjustments. This approach reduces the risk of large-scale disruptions and ensures smooth technology adoption across teams.
- Managing costs: AI projects can quickly escalate costs without proper oversight. We advocate for rigorous cost management practices like conducting detailed ROI analysis and scenario planning to control budget allocation and resource utilization throughout the implementation.
- Focus on prior use cases and customer-facing functions: Our experience shows that starting with customer-facing functions-such as marketing, customer service, and sales-outputs the highest returns. These areas benefit significantly from generative AI's ability to automate repetitive tasks, personalize interactions, and optimize content creation, driving immediate business value.
WHITE PAPER
Choose the best AI approach for your business – explore the guide on Generative vs Predictive AI!
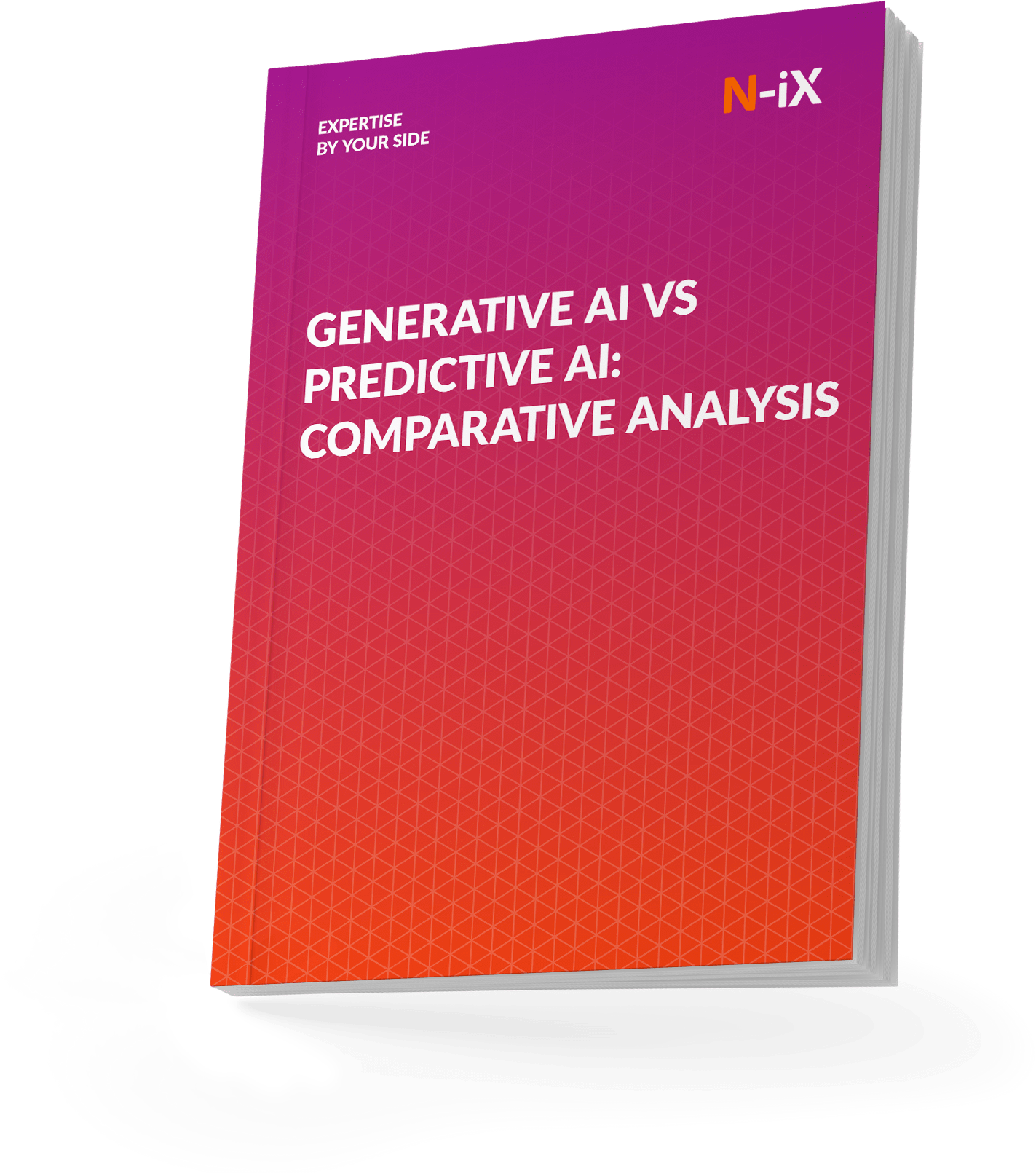
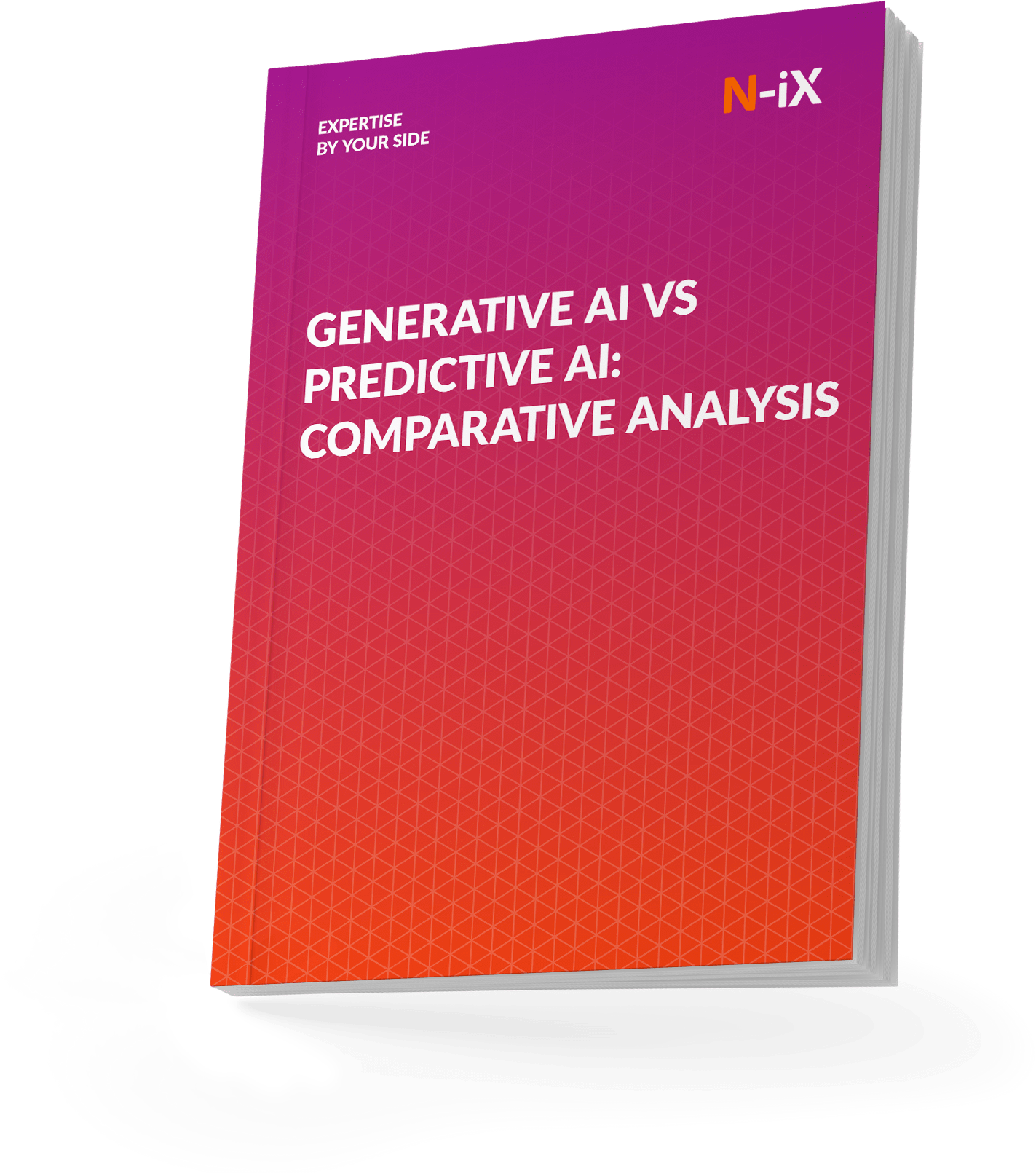
Success!
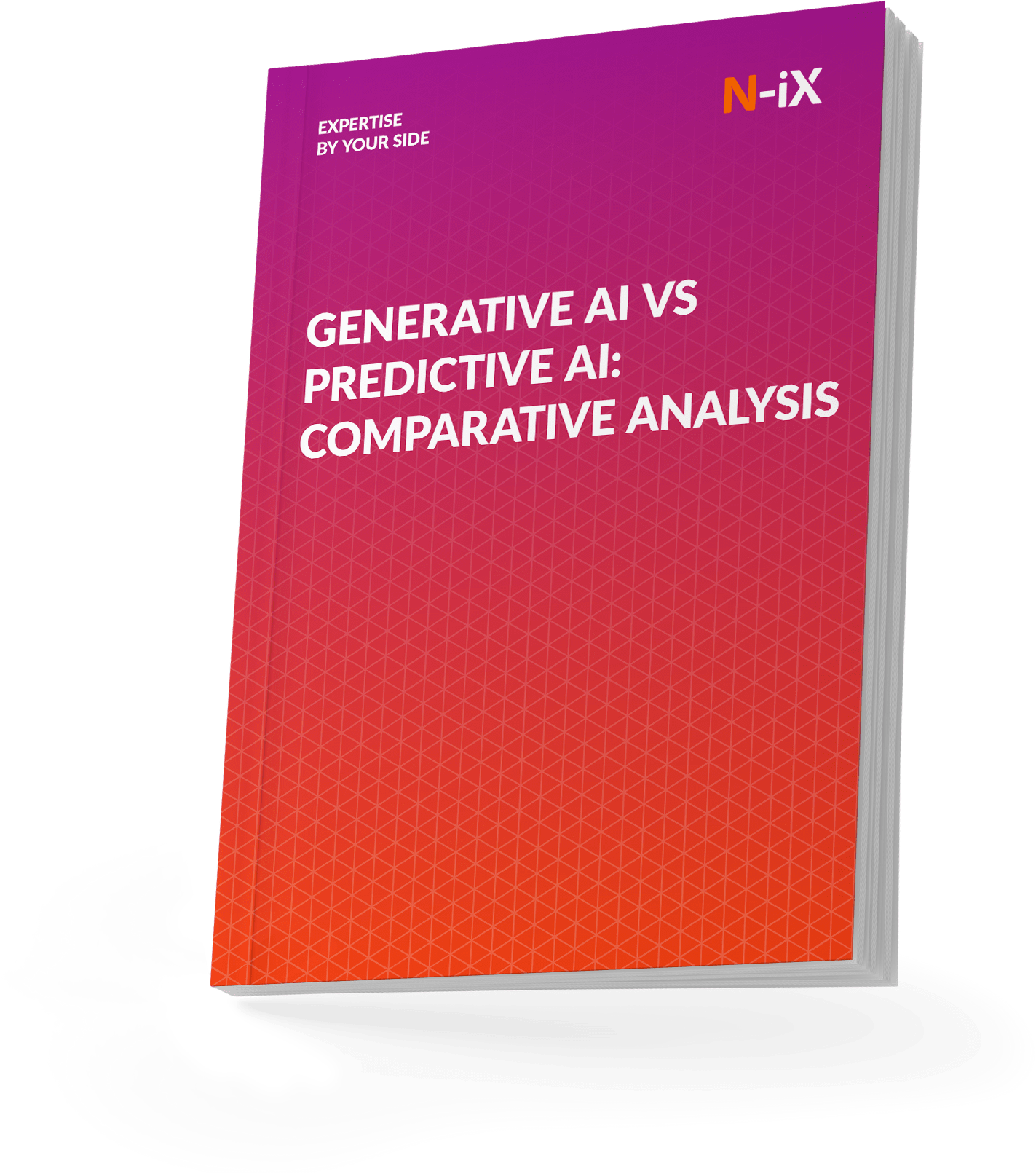
Wrapping up
It's essential to emphasize that successful generative AI implementation is not about jumping into the latest technology trends but about taking a thoughtful, structured approach.
At N-iX, we don't just deliver AI solutions-we partner with businesses strategically, ensuring each initiative aligns with their goals and operational needs. We achieve sustainable growth, measurable results, and long-term success by working with clients. Our approach is systematic, not chaotic, focusing on delivering real value with clear outcomes, trusted by Fortune 500 companies and industry leaders worldwide.
- We have delivered over 60 data science and AI projects, and have a team of 200 data, AI, and ML experts.
- Our certified partnership with Google, AWS, and Microsoft provides our AI developers with state-of-the-art tools to create and deliver secure solutions for highly regulated industries.
- N-iX has been recognized by ISG as a rising star in data engineering, highlighting its excellence and innovation in this domain.
If you want to leverage AI to drive your business forward, N-iX is the partner who can help you turn vision into reality.
Have a question?
Speak to an expert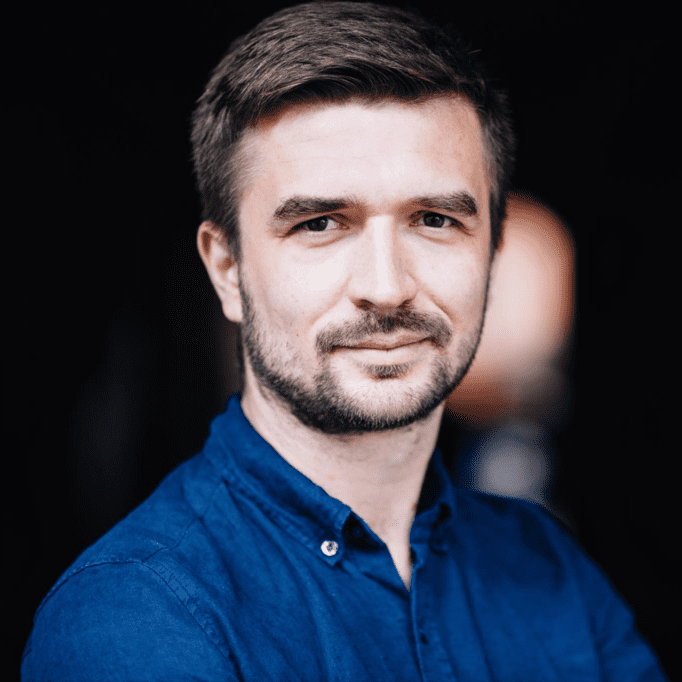