Generative AI, one of the most exciting advancements in Artificial Intelligence (AI), has the potential to revolutionize the way modern enterprises operate, innovate, and grow. However, the concept is still developing in the business world, and many enterprise leaders are yet to fully understand the potential benefits, challenges, and implications of adopting generative AI.
Previously, we’ve covered generative AI use cases and applications, and it’s time to explore the technology from the enterprise angle. What are the specific benefits of generative AI for enterprises? And what factors should you consider before implementing it? We’ll also spotlight two success stories from our generative AI consulting portfolio to showcase the transformative capacities of generative AI for enterprises. Explore how generative AI can help enterprises convert challenges into opportunities and foster lasting business value, operational efficiency, and revenue growth.
Generative AI for enterprises: Outlook
According to the recent McKinsey survey, one-third of the global respondents said their organizations regularly use generative AI in at least one function. On top of that, 40 percent of respondents say their businesses will increase their AI investments because of the recent technological advancements. That means that the capabilities of generative AI are already transforming the industry landscape, delivering tangible business outcomes.
On the disruption side, the same study highlights that three-quarters of respondents expect generative AI to cause significant or even disruptive change in the competitive landscape in the following years. This data is also followed by the Gartner survey, which specifies cloud concentration, mass viability, and financial planning uncertainty as the most commonly cited emerging risks of generative AI in 2023.
Implementing generative AI into enterprise operations requires substantial computational resources and robust data infrastructure. Furthermore, these models might need high-quality, diverse data for training. Therefore, enterprises must develop data collection, management, and governance strategies.
The critical point for enterprises is to develop a comprehensive strategy for generative AI, ensuring it aligns and harmonizes with your existing enterprise AI initiatives. The core principles that govern an AI-driven organization are also essential for successfully implementing generative AI. These include access to curated enterprise data, robust AI governance, and transformation of processes to leverage cognitive workers, among others.
As technology in this area rapidly evolves, it is vital not to navigate this complex landscape alone. Seek collaboration, support, and insights from industry partners well-versed in this field, like N-iX. Our expertise can provide guidance, minimizing risks and maximizing the potential benefits of generative AI integration within your enterprise.
Generative AI for enterprise: Implementation challenges
Adopting generative AI in an enterprise setting is not without its challenges. As companies strive to leverage the power of AI, they often face obstacles that can hinder progress. Integrating AI into existing processes and systems requires a delicate balance between technological advancement and organizational alignment. One important distinction is that consumers and enterprises have different business needs. In particular, enterprises looking to adopt generative AI solutions should ask themselves the following how-to’s:
- How to control data?
- How to manage and mitigate implementation-related risks?
- How to integrate the existing data and applications?
- How to achieve the maximum possible rate of accuracy and explainability?
- How to mitigate fraud and other security considerations?
What’s more, except for the mentioned questions, there are five key challenges that businesses often encounter:
1. Data privacy. Generative AI models require large amounts of data for training. Data that includes personal or sensitive information raises privacy concerns. Even if data is anonymized, there's a risk that individuals could be re-identified based on their profiles. This challenge requires stringent governance policies, compliance with data protection regulations, and potentially, techniques such as differential privacy that add noise to data to prevent re-identification.
2. Contextual limitations of language models. Large language models (LLMs) can generate human-like text and code but struggle with context in complex or specific scenarios. For example, they might need help understanding nuanced prompts or be able to produce consistent answers over a prolonged conversation. Human supervision, fine-tuning the model on specific tasks, and using techniques like reinforcement learning from human feedback can help mitigate these limitations.
3. Output quality and accuracy. Generative AI models can produce outputs that may be nonsensical, inappropriate, or unsuitable for the context in question. This poses a challenge, mainly when the AI generates content for customers or assists in making crucial business decisions. Regular human review and feedback, implementing quality control mechanisms, and fine-tuning the models according to specific guidelines can significantly improve the quality and accuracy of the outputs.
4. Biases in AI models. AI models can inadvertently learn and replicate biases in the training data, leading to unfair or discriminatory outputs. Given the potential for harm, this is a critical challenge, significantly when scaled on the enterprise scale. Using diverse and representative datasets, regularly auditing models for bias, and employing techniques for bias correction can help mitigate these biases.
5. Scalability and integration with existing systems. Implementing generative AI within an enterprise environment often demands significant resources, including processing power, storage, and expertise. Scalability can become a challenge as models grow in complexity and data requirements. Additionally, integrating generative AI models into existing workflows and systems may require substantial customization and technical know-how. Solutions include leveraging cloud-based platforms and modular architectures and partnering with a trusted Big Data vendor to ensure seamless integration and scalability.
Enterprise readiness should be the core of any approach to generative AI adoption. Enterprises must consider the key factors, including data governance, security and compliance, and reliability and sustainability. By understanding and proactively addressing these challenges, enterprises can better leverage the power of generative AI while minimizing potential pitfalls.
Generative AI integration for enterprises: Checklist
When considering the application of generative AI for enterprises, the following hand-picked questions are highly relevant to be reviewed. Before engaging with integration, it’s essential to go through each step of this checklist and develop a well-rounded strategic plan.
Implementation
- What specific problems is your enterprise aiming to solve?
- What infrastructure is in place, and what will be needed to implement AI solutions?
- How will the integration with existing systems work?
Data
- Are there any privacy or security concerns with the data being used?
- How will data be maintained and updated?
Ethics and compliance
- What are the ethical considerations to keep in mind?
- How does the use of generative AI align with legal regulations and industry standards?
Costs and ROI
- What will be the total cost of implementing and maintaining generative AI solutions?
- How will the Return on Investment (ROI) be measured?
Vendor selection
- Are there preferred vendors or partners with expertise in generative AI?
- What criteria will be used to select tools and platforms?
Skills and training
- Does your team have the necessary skills to utilize generative AI?
- What training or hiring will be required?
Risk management
- What risks are associated with implementing generative AI, and how will they be mitigated?
- What contingency plans will be put in place?
Performance metrics
- How will the performance and effectiveness of implemented solutions be monitored and evaluated?
- What KPIs will be used, including but not limited to faster ideation, drastically reduced time-to-market, or unprecedented speeds of identifying new market trends?
Future adaptation and scalability
- How will the solutions be adapted to future technological advancements or changes in business needs?
- How scalable are the proposed solutions to meet growing or changing demands?
These questions can guide a comprehensive analysis and planning process for implementing generative AI in an enterprise, ensuring alignment with business goals, compliance with legal standards, and effective utilization of resources.
Generative AI for enterprise integration: Success stories
Reviewing the success stories is vital to empirically reviewing how AI can impact enterprise growth. At N-iX, we delivered custom generative AI solutions to our partners and would like to highlight the cooperation results.
Automating marketing interventions and ensuring personalized experiences
Based in Germany, Cleverbridge is our esteemed client specializing in comprehensive e-commerce and subscription management. They are experts in seamlessly monetizing digital products, online services, and SaaS across various industries. Their cloud-powered platform addresses recurring billing and customer experience enhancement challenges and is a consolidated solution for global compliance and payment requirements.
Challenge: Cleverbridge sought to reduce its customer attrition rate and boost Customer Lifetime Value (CLTV) by implementing Machine Learning (ML) for predicting subscription cancellation and fine-tuning communication strategies. The challenge also involved utilizing generative AI for the tailored creation of marketing content.
Value: N-iX engineered an ML system tailored to Cleverbridge’s needs, facilitating effortless integration of ML models and continuous support using MLOps techniques. We crafted a specialized multi-tenant ML solution that predicts subscription cancellations and augments it with AI algorithms to recommend impactful communication strategies.
By assisting Cleverbridge in automating marketing responses for segments defined by churn propensity, N-iX enhanced retention through a data-driven methodology, expedited content creation, and minimized manual dependencies. This contributed to more individualized and efficient customer interactions, reflecting the core values and capabilities of both Cleverbridge and N-iX.
Scaling review generation and reducing costs for a P2P review platform
Our client, a leading player in the international technology arena, offers a specialized platform for software comparison, boasting an impressive 60M annual user base. This platform allows users to submit reviews across a multitude of software categories.
Challenge: The client sought to implement aggregate product reviews to enhance their SEO performance. The primary obstacle was identifying the most effective summarization algorithm to provide coherent summaries without sacrificing critical information. The objective was to extract a consistent and structured output from a large language model (LLM), all while constraining the word processing count.
Value: N-iX engineered a solution grounded in OpenAI technology that generated structured aggregate reviews, encompassing general information, pros, and cons for each product. Rigorous testing was conducted on 50 product reviews across 30 distinct categories from the prior six months, and results were meticulously refined based on client input.
Our solution delivered our clients the autonomy from third-party review services they desired and enabled automated review generation, all while ensuring scalability to handle larger data quantities. Utilizing the GPT LLM led to a higher output caliber than smaller NLP models and offered a more rapid implementation timeline than bespoke language models. This efficiency not only cuts down the cost of testing the client's hypothesis but also holds the potential to elevate their SEO should the hypothesis prove accurate.
Bottom line
Generative AI revolutionizes enterprises by offering solutions to learn from data, generate ideas, and drive significant value across sectors. It's redefining boundaries and enabling innovation, creativity, and efficiency that was unimaginable just a few years ago. However, adopting this technology also requires careful planning and consideration of various factors, such as technical requirements, ethical implications, and potential risks.
Embracing generative AI is not just about staying competitive–it's about seizing the opportunity to lead and innovate. As a global company that provides software solutions and engineering services, N-iX can support your business on this journey. With our deep expertise in AI, Machine Learning, and other tech domains, we can help you leverage generative AI to transform challenges into lasting business value, operational efficiency, and revenue growth.
Have a question?
Speak to an expert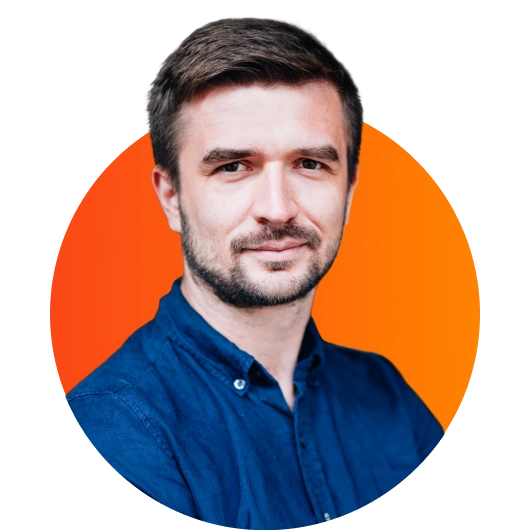