Despite significant investments, many organizations struggle to scale AI effectively. This isn't due to a lack of technology-modern AI tools are more advanced than ever. The core problem lies elsewhere: too many enterprises jump into AI without thinking through its purpose and asking the critical questions: What problems are we solving? How does AI fit into our business operations? How can it scale across the organization? These questions go unanswered when enterprises treat AI as a quick-fix solution rather than a foundational part of their operations.
The problem isn't the technology itself-it's the approach. Businesses often implement solutions without defining use cases, misalign projects with business goals, and overlook the human and operational factors needed for success. AI implemented without a clear roadmap becomes a patchwork of experiments, failing to integrate into core processes or deliver the enterprise-wide impact it promises. Let's explore how enterprises with AI consulting services can move beyond disconnected efforts and develop an AI strategy that works.
Why AI adoption without strategy fails
Approaching AI without a well-defined strategy often leads to wasted resources, misaligned objectives, and missed opportunities. To better understand the challenges, let's address some key questions that highlight the common risks enterprises encounter:
- Why do siloed AI projects often fail? Siloed AI projects lack enterprise integration, impeding scalability and preventing cohesive data and workflow interactions across departments.
- What happens when success metrics aren't defined? Undefined success metrics lead to unmeasurable outcomes, making it impossible to assess ROI or align AI initiatives with strategic objectives.
- How does poor data quality impact AI outcomes? Poor data quality disrupts model accuracy, creating unreliable insights and limiting the effectiveness of AI-driven decision-making.
- What are the risks of ignoring biases and privacy concerns? Ignoring biases and privacy issues damages trust, risks regulatory penalties, and erodes the credibility of AI systems in enterprise environments.
- What happens when organizations neglect change management? Neglecting change management fosters employee resistance, undermining enterprise AI adoption and reducing the effectiveness of implemented solutions.
Now that we understand the difficulties that hinder AI adoption, it becomes clear that success relies on a comprehensive strategy to align AI initiatives with enterprise objectives. Without that strategy, AI may become just another technology that doesn't live up to its promise.
Defining an AI strategy for enterprises
An enterprise AI strategy is a structured, holistic approach to embedding AI into every facet of an organization. The plan requires alignment across several business dimensions-data, technology, processes, and human resources-ensuring that all components work harmoniously to achieve the desired outcomes.
AI systems rely on high-quality data to make informed decisions and need the proper technological infrastructure to process and analyze that data efficiently. Furthermore, the workforce must be ready to adopt and work with AI technologies, which requires adequate training, change management, and an understanding of how AI can augment their roles.
Organizations that take a holistic approach-combining technical, operational, and cultural changes-are better positioned to maximize the value of their AI initiatives. To build an effective AI strategy, enterprises must focus on the following critical factors:
- Scalability: Enterprises operate in dynamic environments with evolving demands. AI systems must scale effortlessly to process expanding datasets, support additional users, or accommodate increased complexity without compromising performance.
- Reliability: AI systems underpin mission-critical operations, where any inconsistency or failure can disrupt workflows and erode trust. For instance, in industries like finance or healthcare, inaccuracies in AI-driven recommendations can have significant consequences.
- Security: AI systems must safeguard this data against internal and external threats. A single breach can compromise trust and lead to regulatory penalties.
- Integration: An AI solution is only as effective as its ability to function within the broader organizational ecosystem. Siloed systems create inefficiencies and duplicate work.
- Governance: AI governance goes beyond setting ethical standards. It is about accountability and control over the impact of AI. For enterprises, this includes setting clear policies on how AI models are trained, deployed, and monitored.
- Technological and organizational readiness: AI success relies not only on advanced technology but also on an organization's culture and readiness to embrace change. Without proper preparation, even the best technologies can fail.
Read more: Generative AI for enterprise: challenges and integration solutions
Key components of a robust enterprise AI strategy
Building a robust AI strategy for enterprises requires integrating technical capabilities, organizational readiness, and ethical considerations into a cohesive framework. At N-iX, we emphasize a comprehensive approach. Below are the key components that define this strategy:
Business-driven approach
For enterprise AI to deliver meaningful results, it must directly address the organization's strategic objectives. At N-iX, our approach focuses on tightly connecting AI initiatives to measurable business outcomes, such as enhancing operational efficiency, driving revenue growth, improving customer experiences, or enabling innovation. Without this connection, AI risks becoming a fragmented tool rather than a driver of enterprise transformation.
To establish alignment, organizations must answer critical, outcome-driven questions:
- Which core business challenges or inefficiencies can AI resolve?
- How will success be defined and tracked through specific KPIs?
- What departments or processes will experience the most immediate impact from enterprise AI adoption?
Accountability is a key component of alignment in enterprise AI strategy. N-iX helps organizations clearly define responsibilities, fostering collaboration among IT, operations, and business stakeholders. This alignment transforms AI into a central part of enterprise strategy rather than an isolated initiative.
Defined use cases
Well-defined and prioritized use cases form the foundation of a successful AI strategy. We specialize in helping enterprises identify specific, actionable problems AI can address, ensuring these initiatives are impactful and achievable. Use cases should meet three essential criteria:
1. Feasibility: Evaluating whether the organization has the data, tools, and processes required for the AI solution to function effectively. Questions to address include:
- Is the necessary data available, high-quality, and accessible?
- Can the existing IT infrastructure support the computational needs of the AI system?
- Are the operational workflows adaptable for AI integration?
2. Business value: Focusing on use cases that deliver tangible benefits, whether in cost savings, operational efficiency, or competitive advantage. Key considerations involve:
- How does this use case align with enterprise objectives and long-term strategy?
- What measurable outcomes or ROI can be expected?
- Which KPIs will track the success of this initiative?
3. Scalability: Ensuring that the solution can be expanded across departments or replicated for similar organizational challenges. Important questions include:
- Can this AI solution handle growing datasets or increasing complexity?
- Is the architecture adaptable for broader deployment across multiple teams or use cases?
Specific examples of high-impact use cases include AI-driven predictive maintenance in manufacturing, where real-time data models minimize downtime, or fraud detection in financial services, which leverages ML to identify and prevent transaction anomalies. These examples highlight the diverse potential of AI across industries.
For instance, N-iX collaborated with a leading ecommerce platform provider to transform their marketing capabilities through ML-driven churn prediction. Using AWS-based serverless infrastructure and MLOps best practices, we enabled subscription churn analysis, customer segmentation, and personalized email campaigns.
Explore more details: Enhancing ecommerce services with ML-powered churn prediction calculation
We emphasize a comprehensive approach to prioritization. Our teams work with organizations to analyze the feasibility of proposed AI projects, focusing on available resources and operational readiness. This process involves mapping data quality, technological capacity, and team expertise to the requirements of each use case.
Critical questions to guide the stage of defining use cases include:
- What specific business problem is the AI solution addressing?
- Who are the stakeholders, and how will they collaborate effectively?
- How will the solution integrate into existing processes without significant disruption?
- What mechanisms are in place to monitor and refine the system post-deployment?
Data strategy
We recognize that the foundation of any enterprise AI strategy lies in a robust and forward-looking data strategy. Data serves as the fuel for AI systems, and its management directly impacts the performance, accuracy, and scalability of AI initiatives. We collaborate closely with enterprises to design data strategies prioritizing current requirements and long-term scalability. Our data strategy framework includes:
- Data centralization and integration: Breaking down silos to establish a unified data ecosystem where all relevant information is accessible and interconnected. This includes designing centralized data lakes or warehouses tailored to AI workloads.
- Data governance and compliance: Implementing stringent governance policies to maintain data quality, lineage, and integrity while adhering to industry-specific regulations.
- Data preparation and labeling: Developing processes for cleaning, enriching, and labeling data. High-quality datasets are critical for effectively training AI models, especially in ML and deep learning applications.
- Real-time data pipelines: Building real-time or near-real-time pipelines to handle large volumes of streaming data, enabling AI systems to react to changes as they occur.
N-iX focuses on creating enterprise data strategy roadmap that addresses both immediate operational demands and the long-term objectives of our clients. For instance, our collaboration with a global ecommerce platform provider highlights how a well-structured data strategy can drive measurable results.
By designing and implementing a modern data platform leveraging AWS and Snowflake, we enabled seamless integration of data sources and established a three-tier data processing model (bronze, silver, and gold layers). This framework powered visual reports through Power BI, offering insights into subscription metrics like revenue and customer growth.
Discover more: Driving growth in ecommerce with a comprehensive data analytics solution
Technology services
Selecting the right AI solutions requires a strategic approach that aligns with your organization's goals and operational needs. At N-iX, we focus on delivering tailored AI services that address your unique challenges, ensuring measurable impact and seamless integration into your existing workflows.
Key AI and ML services we specialize in include:
- Machine Learning services: We develop predictive analytics and optimization solutions that enhance decision-making and drive operational efficiency. From dynamic forecasting to real-time data insights, our ML services enable enterprises to adapt to market changes swiftly.
- Deep Learning solutions: Leveraging advanced techniques, we create systems capable of processing complex data, enabling applications such as visual recognition, autonomous operations, and advanced analytics tailored to specific industry needs.
- Natural Language Processing services: Our NLP services transform how organizations manage communication workflows, automate document processing, and enhance customer engagement through intelligent assistants and chatbots.
- Computer Vision expertise: From real-time object detection to defect inspection and video analytics, our computer vision services enable organizations to drive efficiency in manufacturing, healthcare, retail, and more.
- Generative AI services: We craft creative AI-driven solutions, such as content generation, innovative design tools, and advanced simulations, to explore new possibilities and accelerate innovation.
- Conversational AI solutions: We design and implement intelligent chatbots and virtual assistants that deliver seamless, human-like customer interactions, improving user experiences and operational efficiency.
We bring deep expertise across various AI disciplines. For instance, by leveraging Machine Learning and Natural Language Processing, we helped a leading P2P software review platform enhance its user experience. Using GPT-4 and innovative clustering techniques, we developed a Pros and Cons feature that analyzes user feedback, improving decision-making for over 80 million annual users. This showcases how the right technology choices drive meaningful outcomes at scale.
Keep reading the success story: Improving user experience of a P2P review platform with Machine Learning and NLP
Scalable infrastructure
For enterprise-level clients, we focus on building AI-ready systems designed to handle large-scale workloads, support diverse use cases, and adapt to the evolving demands of AI technologies.
For enterprise AI, cloud and hybrid environments are often central to achieving flexibility and scalability. Cloud platforms provide the elasticity to accommodate growing data volumes and computational requirements. In addition, high-performance computing capabilities are equally critical, particularly for resource-intensive AI tasks. These computationally demanding applications require infrastructure capable of rapid model training and real-time analytics.
We've seen firsthand how companies can overcome the limitations of legacy systems by implementing enterprise AI strategy. One example is our collaboration with a Global Fortune 100 company, where we transformed their logistics operations through a robust, AI-powered infrastructure. By migrating to a cloud-native microservices architecture and integrating advanced technologies like ML and CV, we enabled efficient inventory management for over 400 warehouses across more than 60 countries. The modernized platform automates manual processes, enhances package tracking, and predicts warehouse loads.
Read more: Driving logistics efficiency with industrial Machine Learning
Training and governance
Enterprise AI adoption often introduces fears of disruption or job displacement, which can hinder its success. To address this, N-iX develops tailored training programs for employees across all levels, from technical teams like data scientists and engineers to business managers and non-technical staff. These programs demystify AI, illustrating its role as a collaborative tool that enhances rather than replaces human expertise.
We actively involve employees in the AI adoption process, from pilot projects to feedback loops, creating a sense of ownership. By aligning teams around shared goals and demonstrating the direct benefits of AI in their workflows, we help build trust and reduce resistance to change.
Responsible AI implementation requires clear governance frameworks to ensure ethical, secure, and effective deployment. At N-iX, we integrate governance principles at every stage of AI development and operations. Our approach includes:
- Bias mitigation: Monitoring datasets and algorithms to minimize bias and deliver fair, transparent results.
- Data security: Implementing rigorous controls such as encryption and access management to safeguard sensitive information.
- Regulatory compliance: Staying ahead of global regulations to align AI solutions with legal standards, including GDPR and other industry-specific policies.
- Accountability structures: Defining roles and responsibilities for managing and monitoring AI models, ensuring continuous oversight and improvement.
- Risk mitigation: Conducting thorough testing and simulations to identify and address potential operational or ethical risks before full-scale deployment.
WHITE PAPER
Mitigate AI risks and enhance compliance – get the guide to enterprise AI governance!
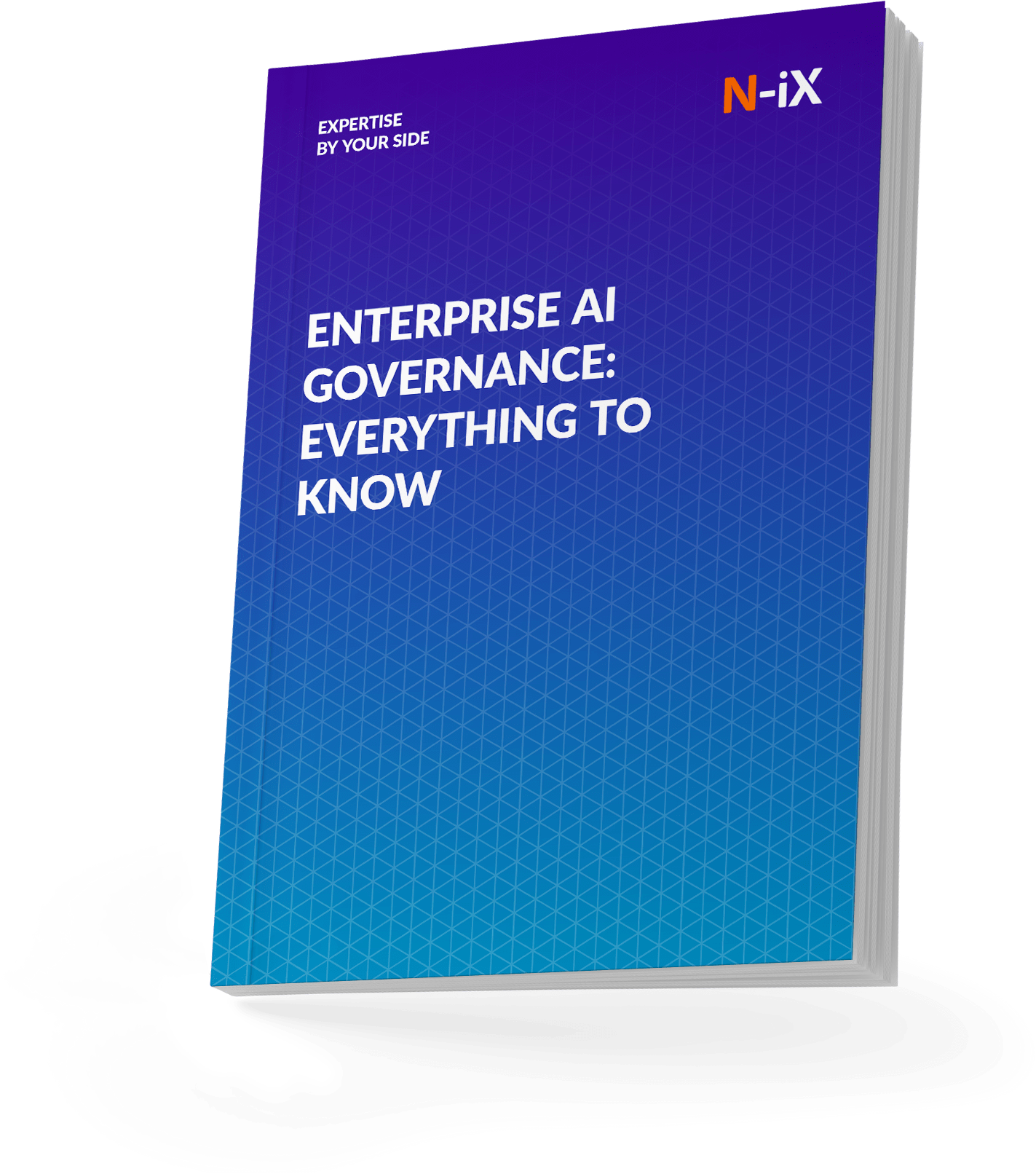
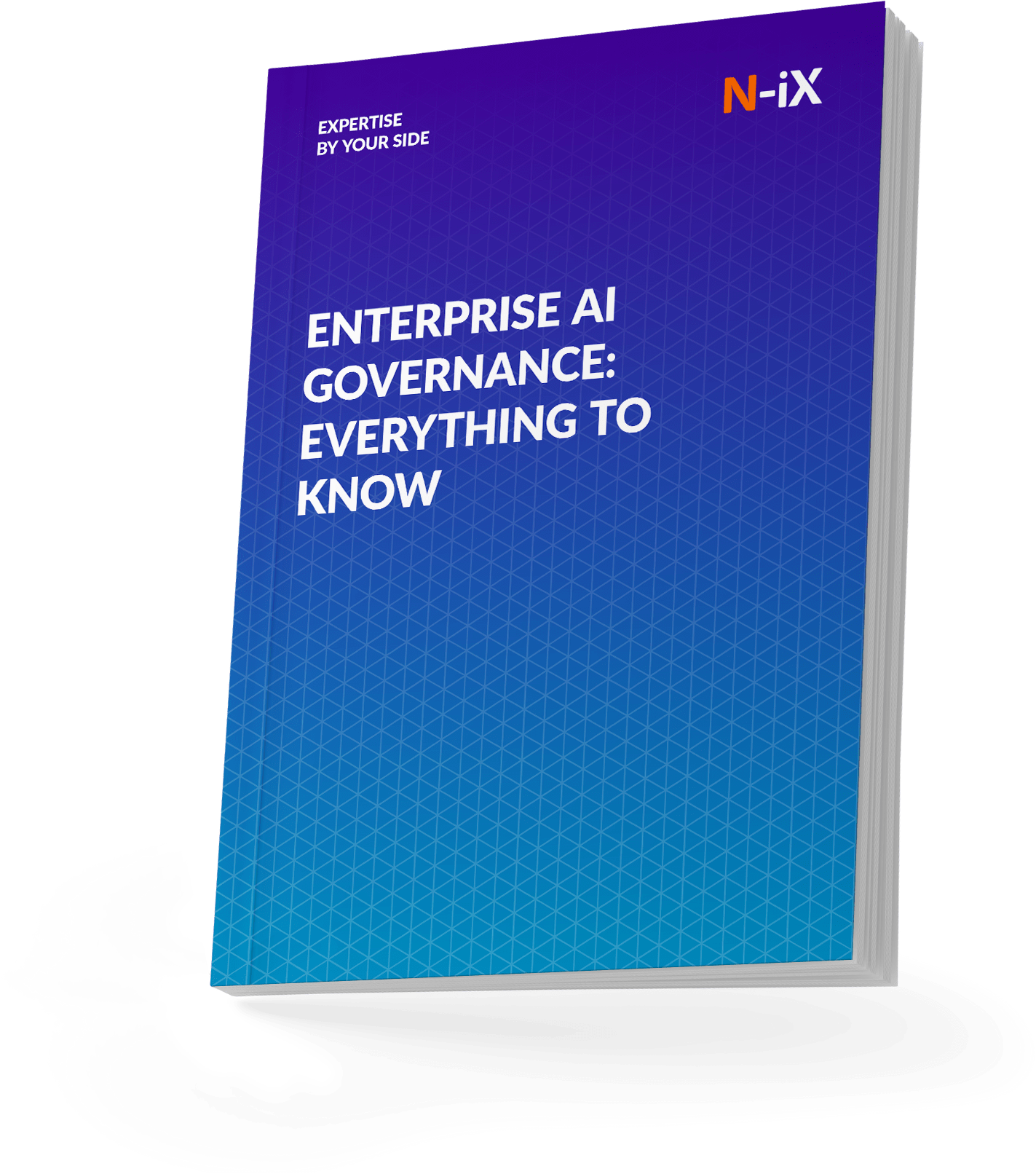
Success!
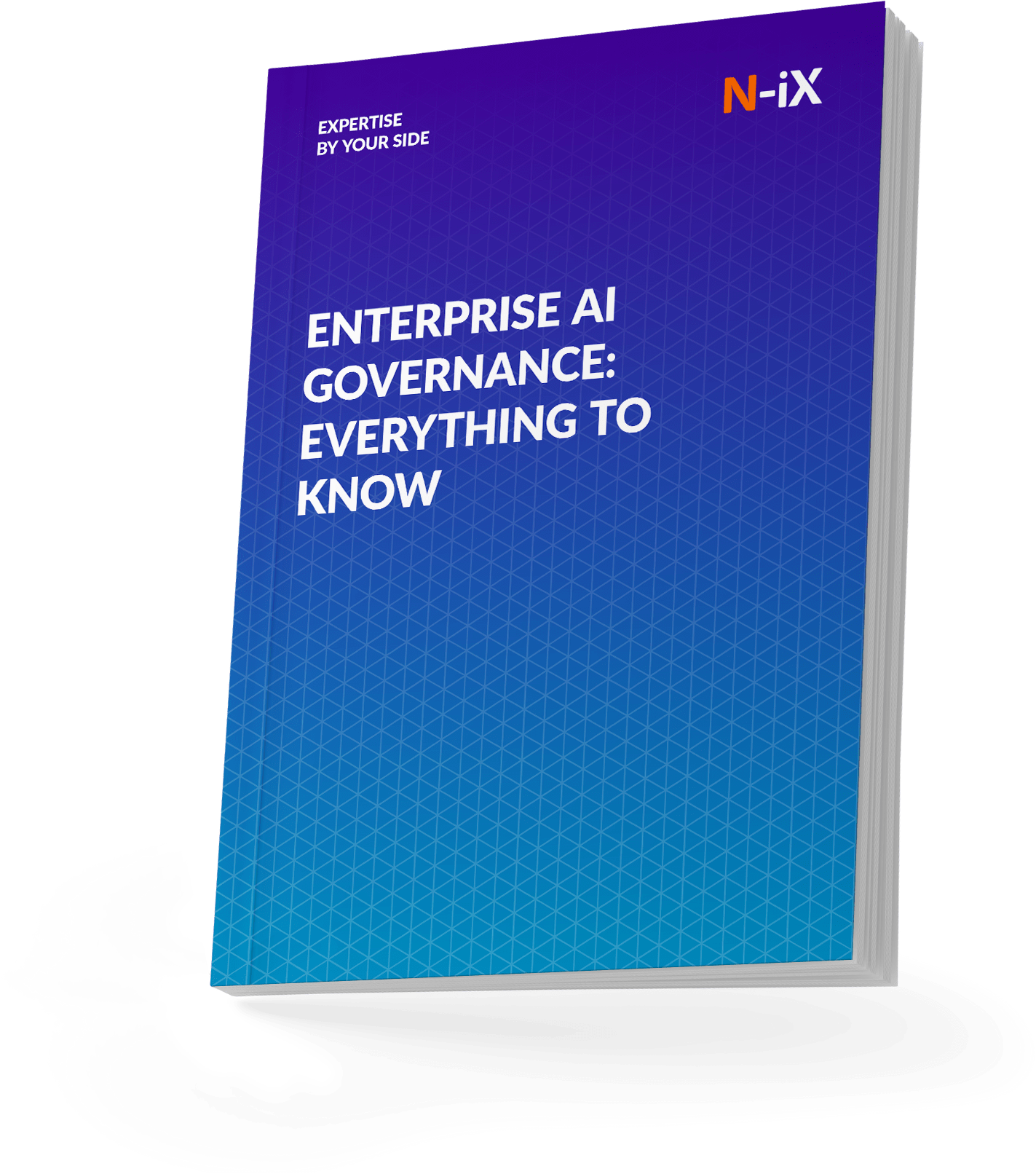
How to implement enterprise AI strategy smoothly
Drawing on N-iX's expertise in AI-driven enterprise transformation, here are the best practices to ensure a smooth and impactful implementation of enterprise AI strategy:
- At N-iX, we embed security protocols into every stage of the AI lifecycle-from data input to model deployment. This includes using algorithmic filters to prevent data poisoning, implementing privacy controls, and deploying observability tools to monitor model behavior.
- N-iX emphasizes the importance of bias mitigation, transparency, and regulatory compliance in every AI project
- Our AI engineering teams upskill employees through tailored training programs and change management strategies. Our mission is to enable teams to embrace AI as a tool that augments, rather than replaces, their expertise.
- We design modular AI architectures that allow organizations to expand their AI capabilities incrementally. Our clients may start small, add functionality as needs grow, and maintain flexibility for future advancements in AI technologies.
- N-iX integrates MLOps practices into AI systems, automating processes like model retraining, performance tracking, and version control.
Bottom line
Without a clear roadmap, AI efforts often end up fragmented-used in isolated pockets of the business with little impact on broader operations. Enterprises need a clear roadmap, one that aligns AI with business goals, integrates it seamlessly into operations, and prepares employees to embrace it as a tool for growth.
N-iX has seen the capabilities of AI unfold when implemented strategically, and we understand the complexities that come with enterprise-scale adoption. Our meticulous enterprise AI strategy is about building a system that scales, adapts, and creates measurable value over time. It's not about chasing trends; it's about crafting tailored solutions that drive real, sustainable impact.
If your organization is ready to take AI from concept to competitive advantage, the path begins with a strategy-not just technology. It's time to transform potential into progress.
Have a question?
Speak to an expert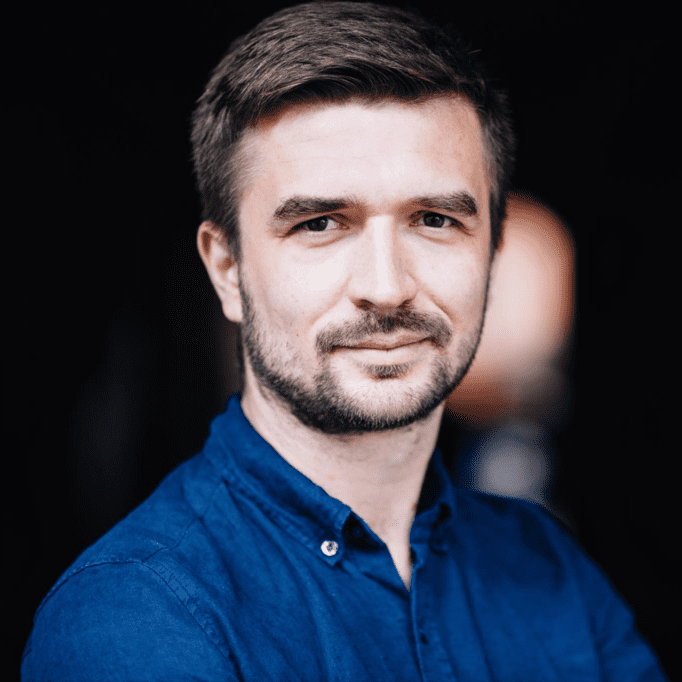