The digital twins technology market has experienced significant growth, with a projected compound annual growth rate (CAGR) of 50% from 2020 to 2030, reaching a valuation of $184.5B. The main driver behind this expansion is the substantial return on investment, with studies indicating that well-executed digital twin projects can yield a 6:1 return, reports the Institute of Digital Engineering. Companies that seek to tap into this growth partner with an experienced software engineering services provider. What is digital twins technology?
Digital twins are virtual representations of physical products with a continuous data flow between the real and digital counterparts. Integrating modern technologies like IoT, Big Data, AI, and ML has expanded DTs beyond products to encompass processes, services, and living entities. A key feature distinguishing DTs from digital models or shadows is their bidirectional data flow, enabling dynamic updates and real-time interactions between physical and digital spaces.
The main characteristics of digital twins are seamless, continuous data exchange with the physical world and real-time updates that ensure accuracy. DTs require dynamic data on the asset and its environment, integrating input from various sources such as IoT sensors and external conditions. This data supports predictive algorithms and decision-making processes. Unlike static models, DTs are equipped with AI that evolves and adapts, allowing simulations, preventive maintenance, and optimization of operations. Their utility spans industries, ensuring that digital twins are dynamic, informed, and responsive tools essential for efficient, data-driven decision-making.
Digital twin model components
A complete digital twin model comprises three main components: data, the model, and software. Data is the foundational element, as digital twins rely heavily on historical and real-time data collected from physical systems, including details about the system's operations and controllers. The model is the core of digital twins, comprising theory-based and data-driven models. Theory models are built on the principles of physical systems, while data-driven models are trained using historical and real-time data through techniques such as AI and data processing. This iterative training process enables continuous self-learning and updating of the model for accuracy.
The software is the essential carrier that bridges physical systems and their digital representations. It incorporates the algorithms and code needed to create the digital twin models and ensures they function as intended. The software runs key functionalities such as representation, diagnosis, prediction, and decision-making. This enables the digital twin to provide optimized commands to the physical controller, enhancing the overall performance of the physical system.
Benefits of digital twins technology
Digital twins technology offers significant advantages across industries by providing holistic and real-time data integration, enabling comprehensive insights and informed decision-making. One key benefit is the integration of data silos, ensuring that all operational and environmental data are consolidated within a shared platform. This allows systems and decision-makers to access a complete picture for better decision-making. Additionally, digital twins enhance predictive maintenance by using AI and ML to analyze data and anticipate problems before they occur, minimizing downtime and optimizing maintenance schedules. They also enable what-if scenario simulations to assess the impact of various decisions without disrupting real-world operations, which can be valuable in fields such as healthcare and smart city management.
Moreover, digital twins improve efficiency and safety by automating repetitive tasks and allowing remote control in hazardous environments, freeing humans to focus on creative tasks. The data-driven insights generated by digital twins, powered by IoT and advanced analytics, support precise and proactive decision-making. This capability enhances process optimization, resource utilization, and significant cost savings. Overall, digital twins' integration, predictive, and simulation capabilities drive higher efficiency and operational safety across various sectors.
Applications of digital twins technology across industries
Digital twins technology has evolved into a vital tool across industries, driving efficiency and informed decision-making. Digital twins enable data integration, scenario simulations, and performance optimization by creating real-time digital replicas of physical assets and systems. Let's explore how industries such as manufacturing, healthcare, and construction leverage digital twins to transform processes and boost productivity.
Manufacturing
Digital twins technology originated in the manufacturing industry and has become a cornerstone of smart manufacturing and Industry 4.0. They are especially impactful in product life cycle management, addressing challenges like data isolation and duplication across design, manufacturing, and service stages. By enabling the testing and validation of models during the design phase and integrating real-time data on the shop floor, digital twins help streamline processes, optimize resource management, and improve manufacturing control. Companies like Siemens have recognized digital twins' significant role in unifying data across all stages of a product's life cycle, enhancing efficiency and predictive capabilities.
Practical applications of digital twins include General Electric's use for predictive maintenance across various sectors, such as power generation and oil and gas, yielding reported customer savings of $1.5B. In the automotive industry, Tesla employs digital twins for every car manufactured, collecting real-time data to enable automatic adjustments and reconfiguration, which boosts operational accuracy and product performance. These examples show digital twins' transformative power in enhancing productivity, reducing costs, and optimizing manufacturing processes.
Read more: Digital twins in manufacturing: benefits, technologies and use cases
Healthcare
Digital twins in technology healthcare began with applications in predictive maintenance and device performance optimization but have since expanded significantly. GE Healthcare's 'Hospital of the Future Simulation Suite' exemplifies this evolution, using digital twins to model patient and staff behaviors, optimize patient flow, and improve resource allocation through data-driven what-if scenario testing. This approach has enhanced bed usage, surgical scheduling, and staff planning, supporting overall operational efficiency. Similarly, Siemens Healthineers, in partnership with Mater Private Hospital in Dublin, developed a digital twin for the hospital's radiology department to manage patient demand and resource use without expanding physical space, resulting in reduced waiting times, faster patient turnaround, and lower staffing costs.
Further, Siemens is advancing healthcare by creating digital models of human organs, aiming for a comprehensive digital twin of the entire human body. Starting with a heart model enhanced with deep learning for simulating physiological responses, such advancements support personalized and precision medicine. These digital twins are utilized for testing drug efficacy, personalized nutrition plans, and tailored treatment systems, offering healthcare practitioners a platform for trial-error experiments without risk to patients. This capability enables precise treatment planning and optimizing medical outcomes and healthcare processes.
Read more: Digital twins in healthcare: transforming patient care and operations
Supply chain
Supply Chain Digital Twins (SCDTs) address key challenges like disruption management and recovery by allowing real-time monitoring and proactive risk mitigation. Using data from IoT sensors, RFID systems, and third-party risk information, SCDTs feed AI algorithms to predict disruptions and simulate responses, aiding decision-makers in optimizing recovery strategies and maintaining network stability. This approach ensures that potential interruptions are anticipated and managed effectively.
Digital twins technology also tackles information asymmetry within remanufacturing supply chains, where data inconsistencies impede decision-making. Continuous, bidirectional data flow between the physical and digital realms helps synchronize and share real-time data across all enterprises in the chain. While adoption depends on enterprise readiness and data security concerns, advancements in IoT, Big Data, and cloud technology support seamless integration and implementation, enhancing overall supply chain transparency and efficiency.
Construction
Historically among the least digitized sectors, the construction industry now leverages digital twins technology to enable real-time, data-driven decision-making and process optimization. A notable example includes a digital twin-based decision support system for managing bulk silo dispatch and replenishment processes, addressing inefficiencies such as delayed material replenishment and lack of usage monitoring. Implementing digital twins in such scenarios has demonstrated potential cost savings and environmental benefits, including a 25% reduction in transportation costs and CO2 emissions through smarter logistics.
Additionally, digital twins are applied in Structural Health Monitoring (SHM) to enhance infrastructure management, such as bridges. Digital twins improve data interoperability and lifecycle monitoring by integrating diverse data for analysis and interpretation. For example, the Netherlands' Rijkswaterstaat has pioneered using a digital twin for a tunnel project to simulate operations, anticipate incidents, and streamline stakeholder coordination, showcasing its practical benefits in large-scale construction projects.
Education
Digital twins serve as effective educational tools by providing high-fidelity virtual representations of physical processes, enabling students to engage in practical laboratory exercises that mirror real-world operations. This approach enhances learning by allowing students to apply theoretical knowledge through simulations without impacting actual systems. Professors supervise these sessions, ensuring students only transition to physical applications after demonstrating proficiency with the virtual models.
The concept of "cognitive digital twins" further elevates education by assisting individuals in managing their learning processes. These digital twins can identify knowledge gaps and suggest tailored learning paths by tracking educational activities such as courses, readings, and publications. This capability supports continuous skill development, helping learners stay current in an era of rapid information growth and knowledge obsolescence.
Smart cities
The advent of advanced sensing and communication technologies has paved the way for smart cities, where real-time data from IoT sensors and other sources monitor urban infrastructure and optimize city services. Digital twins technology plays a crucial role by enabling cities to simulate and manage activities, aiding decision-makers in urban planning, mobility, energy use, and risk management. For instance, Herrenberg, Germany, uses a digital twin combining 3D models, urban mobility simulations, and collaborative data to test solutions for traffic and pollution, fostering public involvement in problem-solving.
Other notable examples include H2Porto in Portugal, a platform that manages the urban water cycle, using predictive analytics to improve service reliability and response times. Virtual Singapore creates a comprehensive 3D model incorporating data on infrastructure, traffic, and environmental conditions for planning and emergency management. Amsterdam's digital twin optimizes traffic flow near the ArenA stadium, leveraging 5G technology for real-time monitoring and improved visitor guidance. These examples illustrate how digital twins enhance urban efficiency, resilience, and citizen engagement.
Challenges of digital twins implementation
Implementing digital twins technology comes with notable challenges that align with the complexities of enabling technologies like AI and IoT. The most significant barriers include high costs due to computational demands, particularly for advanced AI algorithms running on expensive GPUs, and the integration of legacy systems with modern IoT infrastructure. Concerns over data privacy and security, compliance with regulations like GDPR, and maintaining trust in AI processes are pivotal issues. Federated learning is one potential solution for locally safeguarding data by training models, ensuring compliance while avoiding third-party data sharing. Real-time, bidirectional data exchange is critical for Digital Twins, necessitating robust connectivity and data strategies to avoid latency and ensure accurate, timely insights.
The implementation also faces obstacles in data quality, sensor placement, and handling the extensive data generated by IoT devices. Successful digital twin projects require strategic data collection, effective communication infrastructure, and comprehensive planning. Standardization remains lacking, with efforts like the ISO/DIS 23247-1 and the Digital Twin Consortium offering some guidance, mainly on manufacturing. The financial and technical burdens can be daunting for small to medium enterprises (SMEs). However, initiatives like TNO and DIGITbrain provide tailored support for adopting digital twins and promoting accessibility through AI-driven tools and guidance.
WHITE PAPER
Learn how to scale AI initiatives across your enterprise effectively – get the guide now!
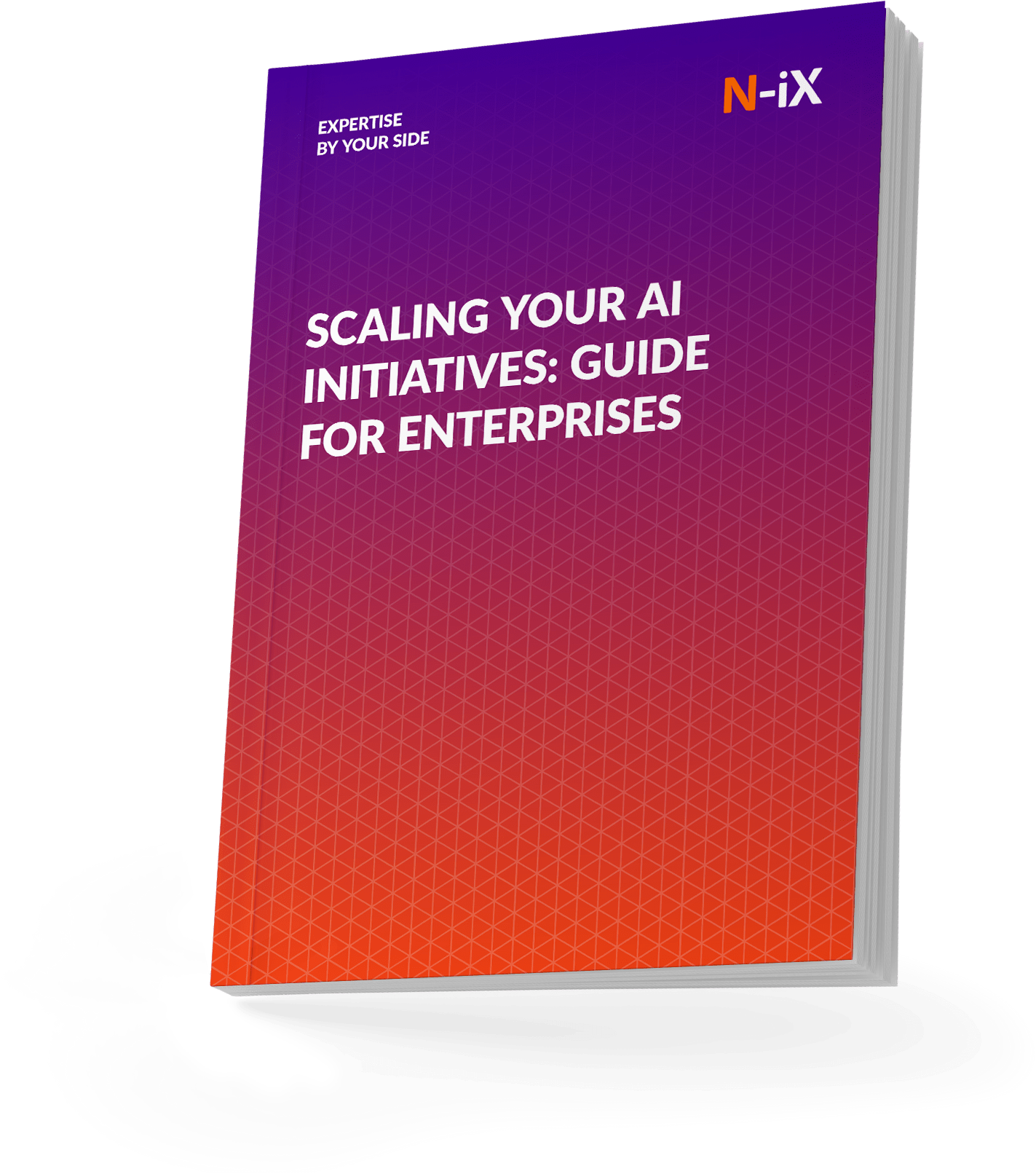
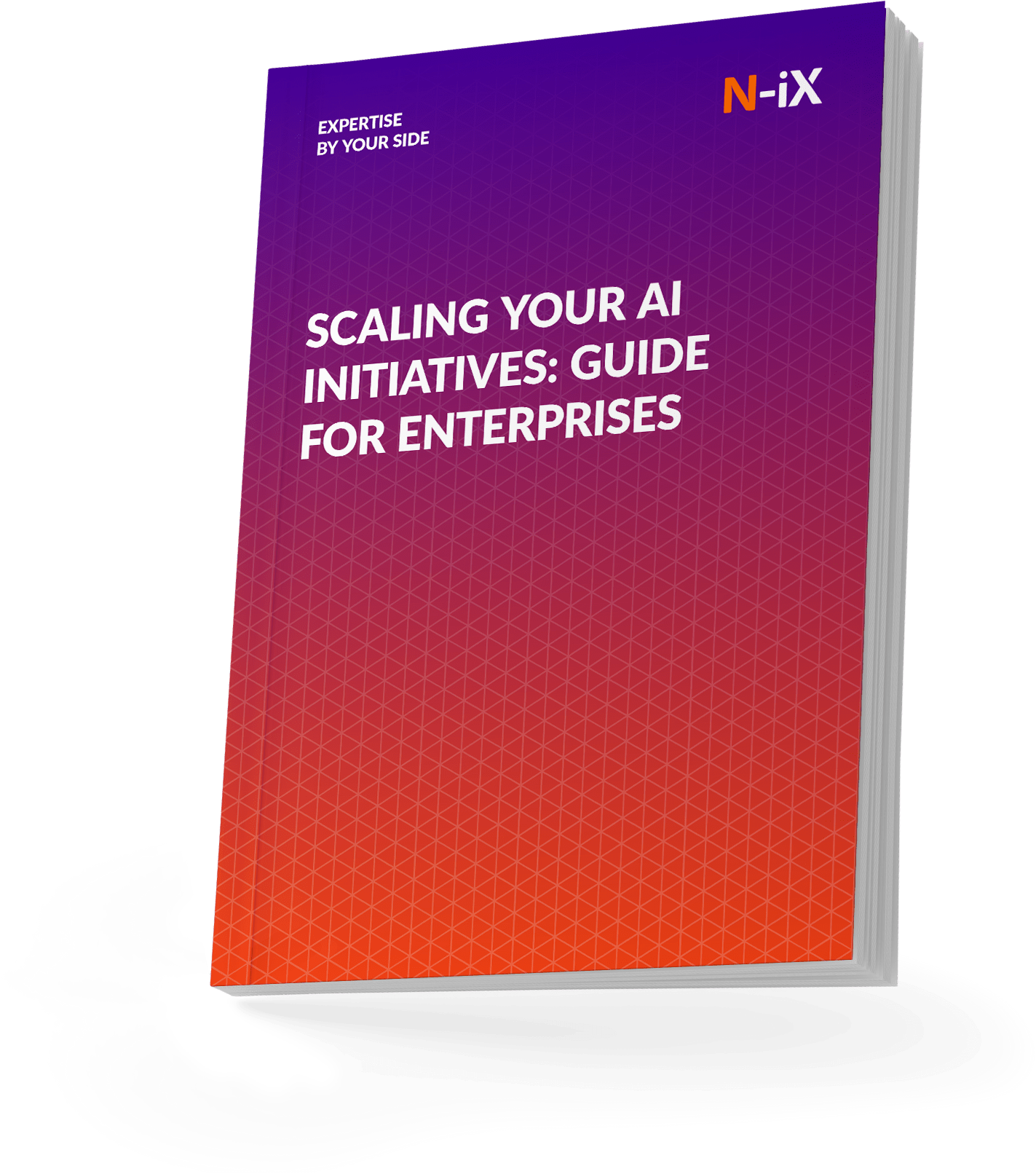
Success!
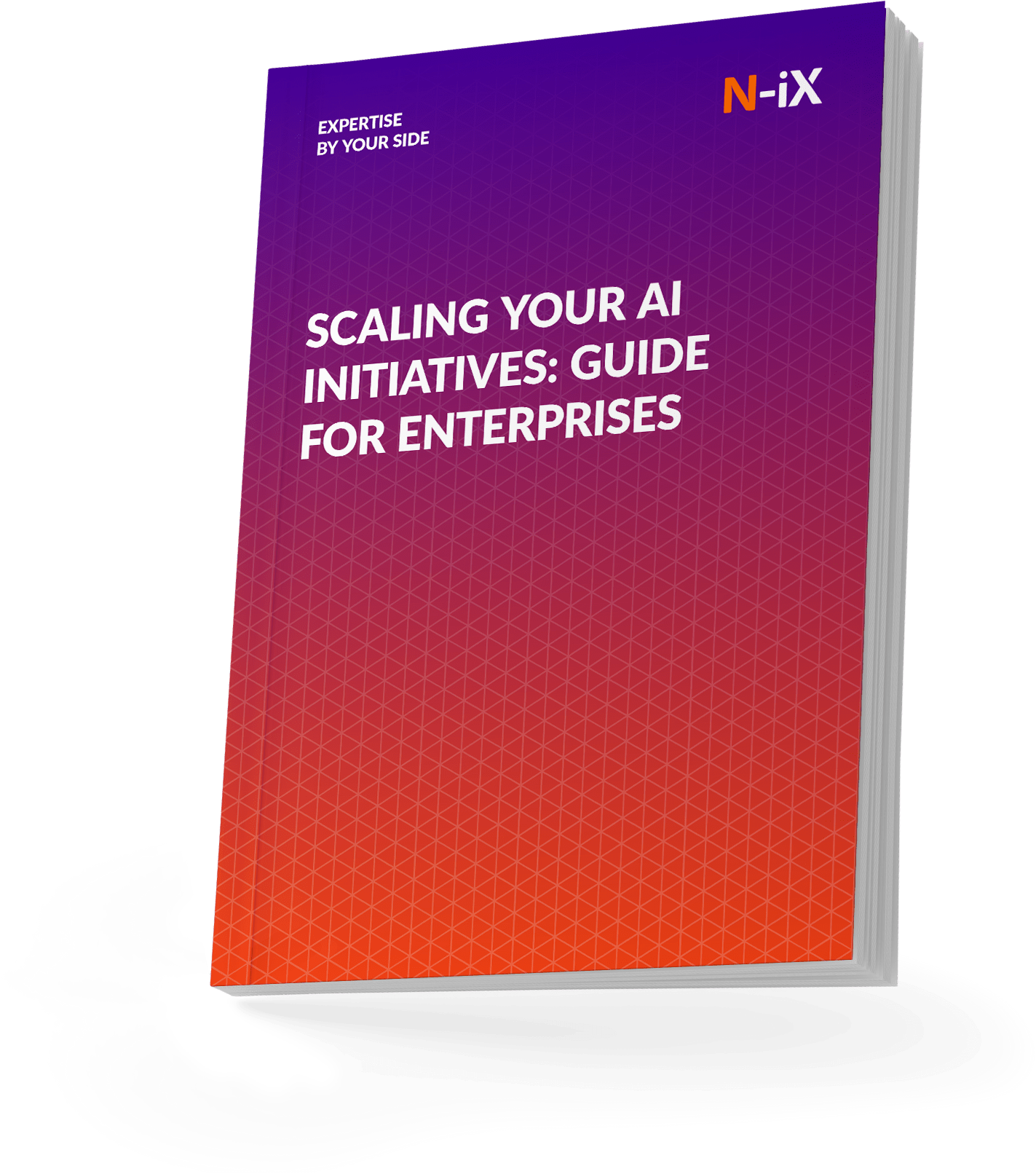
Wrap-up
Digital twins technology has transformed from a concept resembling science fiction into essential digital representations of valuable organizational assets-ranging from devices to full-scale processes and services. DT are pivotal for digital transformation and are integral to Industry 4.0, altering how businesses across various sectors like manufacturing, aerospace, and healthcare operate. Digital twins support users from design and monitoring to analysis and prediction, enabling informed decision-making through real-time data integration.
The implementation of digital twins is closely tied to technological advancements such as IoT and AI, which provide the necessary infrastructure and analytical capabilities. However, as these technologies come with their challenges, organizations must understand both their benefits and potential hurdles to harness the potential of digital twins fully. With technological integration becoming more pervasive, adopting digital twins is increasingly seen as a current and critical step for future-oriented businesses. Partnering with an experienced digitalization partner will be essential for organizations looking to adopt digital twins and effectively drive sustainable innovation and growth.
Have a question?
Speak to an expert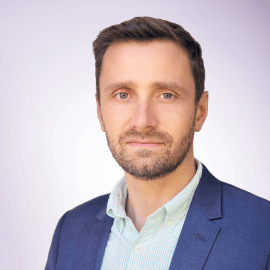