Data science has become a revolution across many industries, and the financial sector is no exception. Finance is the domain where massive volumes of information are generated daily. And, for sure, properly harnessing this data's power can make a big difference. That’s where data science emerges, enabling the transformation of arrays of disorganized information into valuable insights.
The implication of this data-driven approach is felt across every facet of the financial world. Whether we’re talking about seasoned investors, financial analysts, or institutions, they’ve all harnessed the predictive capabilities of data science services to their advantage. It enables them to anticipate market trends, manage risks, optimize portfolios, and analyze complex legal regulations with unimaginable precision and efficiency.
The data revolution in finance
Every day, the financial sector is flooded with an astronomical volume of data. This data comes in various forms – market data, transaction records, customer profiles, and economic indicators at an astonishing pace. Such an amount of data challenges traditional methods of manual analysis and processing, which become insufficient to cope with the scale and complexity of the data.
However, data science in finance is not merely a matter of collecting and storing data; it’s about insights hidden within this information. Data scientists employ various techniques, including advanced analytics, machine learning, and data mining, to extract meaningful patterns and trends from the data. For this reason, let’s explore the primary use cases in more detail to unveil the applications of data science for the financial sector.
Data science in finance: use cases
The intersection of data science and finance has created a dynamic environment where traditional financial models are supplemented with advanced analytics. In this section, we delve deep into various use cases of data science in finance, from risk management to customer service, and discuss the key considerations organizations must be aware of for effective implementation.
1. Risk prediction and management
The transformative impact of data science in the financial sector is perhaps most evident in risk assessment and mitigation. Sophisticated predictive algorithms use diverse data sets to gauge risks with increased accuracy. For insurance companies, these enhanced models inform the underwriting process, enabling more tailored premium assignments based on individual risk metrics. Investment firms also benefit, as predictive analytics provide deeper insights into asset-related risks, thereby optimizing portfolio management strategies.
2. Real-time fraud prevention
Data science algorithms are pivotal in enabling real-time fraud detection. These high-frequency monitoring systems scrutinize every transaction in real-time, applying complex machine learning models to identify aberrations or suspicious activities. These algorithms can instantly flag potentially fraudulent activities like unauthorized credit card use or anomalous transfer amounts by evaluating many variables – from past transaction history and geolocation data to transaction frequency and size. This real-time analytical capability minimizes financial losses and reinforces the integrity and security of financial transactions, serving both institutions and their clients.
3. Regulatory compliance automation
Financial institutions face complex legal regulations at every stage of their work. Data science plays a critical role in automating and streamlining regulatory compliance. Utilizing machine learning models, these automated systems continuously monitor and validate transactions against legal criteria, flagging any discrepancies in real-time. They also integrate anomaly detection algorithms that alert compliance officers of potential regulatory breaches. Beyond real-time monitoring, these data science-driven platforms generate comprehensive, audit-ready reports, thereby easing the burden of regulatory scrutiny and possible investigations.
4. Enhanced credit scoring
Advanced credit scoring models that incorporate diverse data sources to assess an individual’s creditworthiness are where data science is widely used. Besides the traditional methods of credit scoring, primarily based on the credit/debit history and available financial assets (also automated nowadays), data science allows analyzing non-traditional data such as social media behaviors, online shopping habits, and mobile phone usage. By incorporating this diverse data, lenders can establish a more accurate picture of credit risk, resulting in fairer leading decisions based on data overlooked by conventional scoring models.
5. Personalized customer services
Financial institutions leverage data science to go beyond one-size-fits-all solutions, offering highly personalized services and products based on a thorough analysis of each customer's behavior, transaction history, demographics, and preferences. This personalization extends across various sectors, including retail banking, wealth management, and insurance. For example, a data science-driven wealth management platform can construct individualized investment portfolios by analyzing a client's financial goals and risk tolerance, optimizing asset allocation and investment strategies in real-time.
6. Algorithmic trading optimization
Algorithmic trading represents a critical application of data science finance, elevating automated trading strategies to new levels of precision and efficiency. While algorithmic trading systems automatically execute trades based on set criteria and real-time market conditions, data science enhances these processes by continually refining and adapting the underlying algorithms. Through machine learning and advanced analytics, data science allows for developing more nuanced and effective trading strategies, ensuring that trading algorithms can adapt to market changes swiftly and accurately.
7. Wealth management and financial planning
Financial advisors and wealth managers leverage data science to formulate highly personalized financial plans, fine-tuned to each client's specific financial goals, risk appetite, and current financial standing. These data-driven models are robust frameworks for delivering precise recommendations on asset allocation, investment strategies, and retirement planning. The application of data science heightens the accuracy of financial planning and dramatically reduces the manual labor involved, allowing for a more streamlined and efficient advisory process.
8. Market sentiment analysis
In the financial sector, data science is employed to sift through enormous unstructured data from social media, news platforms, and other online resources, providing a nuanced understanding of public sentiment towards specific financial assets or market conditions. This sentiment analysis equips market players with insights into how assets or conditions are perceived, enabling a more informed anticipation of potential market shifts. The process harnesses machine learning algorithms and natural language processing to distill complex emotions and opinions into actionable investment intelligence.
Data science in finance: challenges and considerations
As organizations in the financial sector increasingly adopt data science to gain a competitive edge, several critical aspects must be considered for successful implementation. These range from ethical concerns to skill requirements and financial investments. Here, we delve into these considerations in greater detail, shedding light on the multifaceted journey toward integrating data science in financial operations.
-
Ethical use of data
The ethical implications of using data science in the financial sector are significant and require vigilant oversight. Financial institutions must be transparent in collecting, storing, and utilizing customer data. It involves clear communication about data usage policies and obtaining informed consent from customers. Such ethical practices form the bedrock of a trusting relationship between institutions and their clients.
-
Skill gap
Integrating data science into the financial industry is not just a matter of applying algorithms; it also demands specialized finance and data science expertise. Institutions face a distinct skill gap and must decide between upskilling their existing workforce through targeted training programs or contacting an external vendor.
-
Technology investment
Deploying data science solutions in finance requires a substantial technological investment. Whether modernizing existing infrastructure or introducing new analytical tools, these efforts come with a price tag. Therefore, a rigorous cost-benefit analysis is crucial to ensure that the investment aligns with the anticipated ROI and contributes positively to the long-term business strategy.
In summary, these considerations are not just hurdles but essential elements defining the roadmap for effectively implementing data science in the financial sector. Now, consider how partnering with N-iX can reshape your operations with data science integration services.
Read more: Data science consulting: Process, visualize, and monetize your data
Unlocking transformation: How N-iX drives data science excellence in finance
Coping with the challenging landscape of data science in finance requires a trusted partner with the know-how to integrate sophisticated technologies seamlessly. N-iX emerges as that crucial ally, backed by over 21 of cross-industry experience and a global footprint that spans 25 countries. Here's why you should consider N-iX as your go-to partner for data science in finance:
- Tech mastery meets domain knowledge: Our expertise isn't confined to technology. N-iX offers a wide range of services, from cloud computing and data analytics to machine learning, designed to meet the challenging needs of the financial sector. Our tech expertise and profound industry insight position us uniquely to solve your most challenging problems.
- Building credibility through strategic alliances: Our portfolio isn’t just robust; it's proven. N-iX's history of partnerships with industry pioneers and Fortune 500 companies attests to the dependability, quality, and high trust clients place in us.
- Driving business value, not just building solutions: At N-iX, we go beyond delivering technical solutions. Our approach is centered around generating enduring business value. We aim to elevate your operational efficiency, carve out new revenue opportunities, and align our offerings with your overarching business objectives.
- Cross-sector experience: Our extensive experience is wider than the financial sector. We've delivered impactful solutions across diverse manufacturing, retail, and supply chain industries. This multidisciplinary knowledge empowers us to offer more innovative solutions for your financial challenges.
Choosing N-iX means entrusting your data science needs to a partner with a comprehensive understanding of technology and the financial landscape. Let us help you navigate the complex journey of integrating data science into your financial operations, turning challenges into lasting business value.
Have a question?
Speak to an expert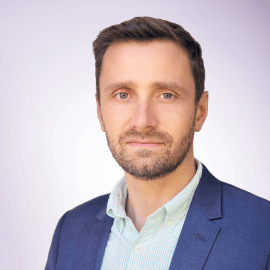