As enterprises manage vast amounts of structured and unstructured data, choosing the right data management architecture is crucial. Data lakes help organizations overcome challenges like data silos and inconsistent formats by providing a centralized repository for diverse data types. They also enable real-time analytics, empowering businesses to make data-driven decisions efficiently.
However, navigating the broad spectrum of data lake use cases and aligning them with your business needs requires expertise and strategic planning. Partnering with a reliable provider offering data lake consulting services can ensure you select and implement the right solutions effectively. From designing the architecture to optimizing performance, an experienced partner helps maximize the value of your data assets while minimizing risks.
What is a data lake? What are the 10 best data lake use cases? Which practices help to face the challenges associated with data lakes? Let’s find out.
What is a data lake, and how does it work?
A data lake is a modern data management solution that provides a single repository for diverse datasets, from structured transactional records to unstructured images and videos. Its primary advantage is its ability to ingest data without predefined schemas, making it a key tool for businesses managing large and varied datasets. While traditional systems like data warehouses are optimized for structured data, a data lake’s architecture supports the storage, processing, and analysis of all data types in one place.
Key stages in the data lake process:
- Data ingestion: Information is collected from multiple sources such as IoT devices, APIs, databases, and streaming platforms. Both batch and real-time ingestion methods ensure seamless integration and constant data flow.
- Storage: Data is stored in scalable solutions like AWS S3 and Azure Data Lake Storage, preserving its native structure for future analysis or transformations.
- Processing and analytics: Distributed processing frameworks, such as Apache Spark, prepare and analyze data, enabling advanced applications such as machine learning, AI development, and predictive analytics.
- Data governance and management: Robust governance mechanisms, supported by metadata catalogs and compliance with regulatory standards, ensure that the data lake remains a valuable resource over time.
Ready to scale up? Discover more about the enterprise data lake
Data lake use cases
Data lakes are highly adaptable, supporting a broad spectrum of use cases that drive innovation and efficiency across industries. Their strength lies in their ability to store, process, and analyze diverse datasets, enabling businesses to uncover insights that were previously inaccessible. Below are detailed descriptions of key use cases:
Advanced analytics and business intelligence
Data lakes enable organizations to consolidate and analyze structured and unstructured data, providing the foundation for advanced analytics and business intelligence (BI). Retail enhances demand forecasting by integrating historical sales data, real-time inventory updates, and external factors like weather or seasonal trends. Supply chain companies use data lakes to optimize inventory management and improve transportation efficiency, leading to cost savings and better decision-making. Businesses can uncover trends, forecast demand, and develop strategies to drive revenue growth by connecting BI tools like Power BI or Tableau to data lakes.
Powering ML and AI applications
One of the most impactful data lake use cases is made possible by supporting the data-intensive processes required for Machine Learning and AI applications. Financial institutions use them to train fraud detection models by combining transactional data with behavioral analytics. Healthcare organizations leverage data lakes to aggregate patient records, medical imaging, and genomic data for personalized treatments and disease prediction. Data lakes accelerate the development and deployment of ML and AI solutions, driving innovation across industries.
IoT data management
IoT devices generate continuous streams of data from sensors and machines, which data lakes efficiently capture and store. In logistics, companies use data lakes to monitor fleet efficiency by analyzing vehicle performance and optimizing routes. Manufacturers leverage them for predictive maintenance to reduce downtime by identifying potential equipment failures before they occur. The scalability of data lakes ensures that they can handle the vast amount of data IoT devices produce, making them an essential component of IoT data management.
Customer experience enhancement
By aggregating customer interaction data from various touchpoints, data lakes create a comprehensive view of customer behavior. Ecommerce platforms use them to deliver personalized product recommendations, combining browsing history, purchase data, and demographic information. Streaming platforms utilize data lakes to enhance user engagement by offering tailored content suggestions. These insights enable businesses to refine their offerings, improve customer satisfaction, and drive loyalty.
Supply chain optimization
Data lakes provide supply chain managers real-time visibility into operations, integrating data from suppliers, transportation networks, and warehouses. This enables companies to analyze delivery schedules, predict disruptions, and improve vendor relationships. For instance, food distributors use data lakes to optimize delivery routes by analyzing weather conditions and traffic patterns. Bringing together and analyzing diverse data streams enables businesses to streamline operations, eliminate inefficiencies, and improve supply chain performance.
Risk management
Risk management relies on analyzing large datasets to identify potential threats and vulnerabilities. Insurance companies use data lakes to evaluate claims, detect fraud, and predict high-risk customer segments. In the energy sector, data lakes monitor equipment performance and flag potential failures to prevent costly outages. By offering a centralized platform for risk-related data, data lakes help organizations take proactive measures to mitigate risks and maintain compliance.
Real-time and streaming data processing
Many industries, such as telecommunications and ecommerce, rely on real-time insights to improve operational efficiency. Data lakes support real-time data ingestion and analysis, enabling applications like dynamic pricing, fraud detection, and real-time performance monitoring. For example, telecom companies use data lakes to reduce reporting delays, providing near real-time updates that empower teams to act faster. The integration of streaming data ensures businesses can derive immediate value from live insights.
Data archiving and compliance
Data lakes securely store historical and compliance-related data, ensuring it remains accessible for audits and long-term analysis. Industries such as healthcare, finance, and government rely on data lakes to meet regulatory requirements, including GDPR, while optimizing storage costs through tiered storage solutions. This approach simplifies compliance management while maintaining data accessibility for strategic decision-making.
Data-driven application development
The scalable and flexible infrastructure provided by data lakes supports the development of data-driven applications, such as recommendation engines, financial forecasting tools, and patient management platforms. These applications benefit from the ability to process large datasets in real time, enabling businesses to deliver innovative solutions that adapt to changing requirements. By integrating modern processing frameworks like Apache Spark, data lakes streamline the development of applications that drive competitive advantage.
Enhanced cross-departmental collaboration
Data lakes break down data silos by consolidating information from across an organization, enabling seamless collaboration between departments. For example, marketing teams can access shared datasets to align their strategies, while product development teams can analyze customer feedback and market trends stored in the data lake. This centralized approach fosters a data-driven culture and ensures all teams work with the same reliable insights.
Data lake use cases applied by N-iX
Predictive maintenance through a data lake for in-flight connectivity
Our client faced significant challenges with maintaining their satellite antennas. Frequent failures resulted in costly maintenance and customer dissatisfaction, while no-fault-found cases further exacerbated inefficiencies.
N-iX collaborated with them to build a robust data lake and predictive analytics platform. The platform consolidated data from IoT devices and operational logs, enabling ML models to predict antenna failures with high accuracy. By analyzing patterns in the data, N-iX developed algorithms to identify failure risks and recommend proactive maintenance schedules.
The solution significantly reduced no-fault-found rates by 75%, improved the accuracy of failure predictions to over 90%, and optimized maintenance processes. This enhanced customer satisfaction and minimized operational costs.
Azure-based data lake for real-time reporting in telecom
A leading telecom company faced challenges with delayed reporting caused by the limited scalability and performance of its legacy systems. Reports would often come with delays of 3-4 hours, impacting decision-making and operational efficiency.
To address this issue, N-iX implemented an Azure-based data lake that enabled almost real-time data streaming and reporting. The solution consolidated data from multiple sources into a unified repository, leveraging modern data processing tools to ensure the timely availability of insights for multiple departments.
The implementation reduced reporting delays significantly, providing near real-time updates that empowered teams to make faster, more informed decisions. This improved operational agility and enhanced cross-departmental collaboration.
Benefits of data lakes
Data lakes offer several advantages that make them a critical part of modern data management strategies:
- Breaking down data silos: Data lakes consolidate data from multiple sources into a unified platform, eliminating silos. This enables seamless collaboration across departments and ensures that decision-makers have access to comprehensive datasets.
- Scalability and flexibility: Data lakes are designed to handle massive amounts of structured, semi-structured, and unstructured data, making them highly scalable for growing data needs. Their flexibility supports a wide variety of analytics and applications.
- Cost-effective storage: Data lakes, which leverage cloud-based solutions, provide cost-efficient storage for vast datasets, including archival and historical data.
- Facilitation of advanced analytics: Data lakes empower organizations to perform sophisticated analytics by providing raw data for AI, machine learning, and predictive modeling.
- Enabling real-time insights: With support for real-time and streaming data, data lakes allow organizations to derive immediate insights critical for operations and decision-making.
Read about the difference and learn how to choose between a data lake vs data warehouse
Challenges and best practices to overcome them
Avoiding the data swamp
Unmanaged and unorganized data in a data lake can lead to a "data swamp," where information becomes difficult to locate, interpret, or utilize effectively. This often results from a lack of governance, metadata management, and defined structure for data ingestion. When data lakes turn into swamps, they fail to deliver value and become a costly burden.
To mitigate this risk, N-iX implements structured data lake architectures that define clear layers. Automated metadata tagging and cataloging tools, such as AWS Glue or Apache Atlas, provide us with transparency into data origin and transformations. Additionally, processes for regular audits, quality checks, and user access monitoring are applied to ensure the integrity and organization of the data lake over time.
Ensuring data quality and accessibility
Inconsistent data formats and a lack of standards across various sources often lead to challenges in maintaining quality and accessibility. If data cannot be trusted or easily accessed, it undermines the entire value of the data lake for analytics and decision-making.
To address this, N-iX employs automated data pipelines that cleanse, standardize, and validate information as it is ingested into the data lake. Tools such as Apache Spark or Databricks transform and harmonize data across sources. For accessibility, role-based access control (RBAC) ensures that datasets are securely available to the right users while maintaining usability for analytics teams. This approach enables organizations to deliver reliable, high-quality data to all stakeholders while maintaining governance.
Managing scalability and costs
Handling the exponential growth of data without incurring excessive storage and processing costs presents a significant challenge. Storing vast amounts of both raw and processed data can lead to inefficiencies if not carefully managed.
N-iX designs cost-efficient data lake solutions by leveraging cloud storage platforms like AWS S3 or Azure Data Lake Storage. These platforms offer tiered storage that balances performance and cost by allocating high-frequency data to performance-optimized tiers and archiving less critical data in cold storage. Scalable processing frameworks, such as serverless configurations of Apache Spark or Databricks, allow resources to scale up or down based on workload demands. Cost monitoring tools further ensure resource efficiency while meeting business requirements.
Complying with regulatory requirements
Meeting compliance requirements such as GDPR or PCI DSS is a challenge when managing sensitive data in a large-scale data lake. Failure to adhere to these regulations can result in legal penalties and loss of trust.
To address compliance, N-iX integrates encryption at rest and in transit, using tools such as AWS Key Management Service or Azure Key Vault. Role-based access policies are implemented to ensure that sensitive data is only accessible to authorized users. Comprehensive audit logs are established to maintain a clear record of data access and usage, helping clients adhere to regulatory standards while safeguarding their data assets.
Integrating real-time data streams
Processing real-time data streams alongside batch data can be technically complex, especially for use cases like dynamic pricing or fraud detection. Traditional data lakes often struggle to handle low-latency requirements effectively.
To overcome this challenge, N-iX incorporates real-time processing tools like Apache Kafka, AWS Kinesis, or Azure Event Hubs into the data lake pipeline. These solutions enable seamless streaming data integration with minimal latency, ensuring that time-sensitive applications can deliver actionable insights. The system is designed for flexibility and scalability, supporting fluctuating data volumes without compromising performance.
Conclusion
The extensive range of data lake use cases underscores their effectiveness in tackling complex business challenges across various industries. Implementing a well-architected data lake enables organizations to navigate through their data, extract valuable insights, and improve decision-making. However, to fully harness the benefits of these data lake use cases, partnering with an experienced provider is essential.
With over two decades of experience, N-iX has a proven track record in delivering software and data solutions across various industries. Our team of 2,200 experts offers comprehensive data lake consulting, implementation, and optimization services. By collaborating with N-iX, organizations can ensure their data lake infrastructure is robust and efficient, which maximizes the value derived from their data assets.
Have a question?
Speak to an expert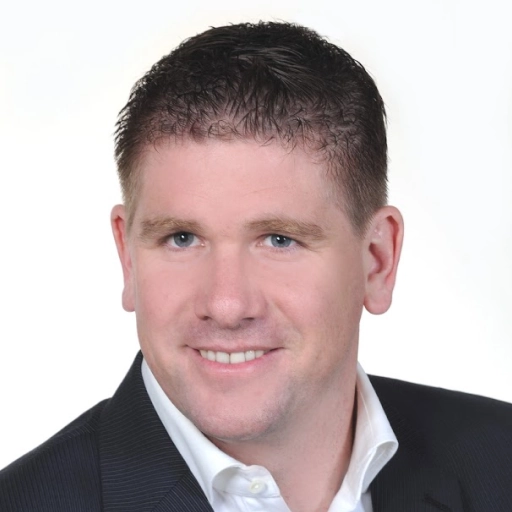