Building and retaining a base of loyal customers is crucial for any business. They not only cost significantly less to retain than acquiring new ones but also tend to spend substantially more, boosting overall profitability. Customer satisfaction is the number one predictor for return purchases. 60% of customers will only return if service is satisfactory, and 94% say that a good experience will likely lead to another purchase [1&2]. Data analytics tools in ecommerce software development allow retailers to benefit from the vast user data they collect. Customer service analytics is a set of tools to track, process, and interpret data throughout the customer experience. Meaningful interpretation of this data enables businesses to make informed improvements in customer satisfaction and, ultimately, brand loyalty.
Let’s examine how customer service analytics help identify improvement opportunities across seven key customer experience metrics.
Find improvement opportunities across KPIs of customer service
Only a third of customer support managers review KPIs at least once a week [3]. 21% of organizations review their KPIs yearly. This means that if a problem arises, leading to a drop in metrics, these organizations could lose customers for up to 12 months before discovering the issue. Collecting, processing, and analyzing feedback through traditional methods can take time, delaying the ability to respond to issues or capitalize on positive trends. In contrast, modern customer service analytics systems update data hourly or even in real-time, enabling the quick detection of trends and anomalies. Thus, management teams can swiftly address any problems, thereby minimizing potential customer loss and improving overall service quality.
Another aspect of customer service analytics is the diversity of the types of input data: text messages, emails, phone conversations, social media communication, and surveys. Traditional tools rely on customers voluntarily providing feedback, which can lead to biased input as only the most satisfied or dissatisfied customers tend to respond. Big data analytics can make sense of all the generated data, regardless of the format. This creates a more complete and accurate image of the customer experience.
Here is how analyzing customer service KPIs can help diagnose problems and identify areas for improvement.
Customer satisfaction
Asking customers to rate their interactions can help businesses understand how well their customer service is performing. A simple feedback form can help identify which issues are being resolved effectively and which are not. Analytics software will identify trends in these scores and explore the causes of any changes.
NLP tools are a more sophisticated way to approach customer satisfaction tracking. Based on textual input, models can estimate the customer's mood throughout the conversation. For example, if the tone of the messages changes from happy to frustrated, it indicates low customer satisfaction and vice versa. After conducting this analysis, it is possible to identify parts of the interaction that are likely to cause a change in mood. For example, customers might be upset about waiting times or the lack of return options. This suggests that the business underestimated the importance of these aspects for customer satisfaction, and change would be beneficial.
Read more: NLP in ecommerce use cases
Analyzing what customers frequently inquire about can provide valuable insights beyond customer service, impacting overall business management. Frequently asked questions often highlight recurring issues or areas of confusion. This information can guide product development teams to address these pain points through design improvements, additional features, or better user instructions. Frequent questions about certain processes, such as returns or billing, can highlight areas where internal processes may be complex or confusing, prompting a review and optimization to enhance customer satisfaction and operational efficiency.
Total time to resolution
Creating a model of the query resolution process helps visualize the workflow, identify inefficiencies, and determine where improvements can be made. Analyzing operators’ actions at each step can highlight bottlenecks and streamline workflows. For instance, a high average time to assign a query to an operator might indicate an understaffing issue or disproportionate operator specialization.
Deloitte’s study of customer service performance finds that employee satisfaction is highly correlated with end customer satisfaction [4]. Essentially, the happier and more satisfied the employees are in their roles, the better the service they provide to customers. Optimizing work schedules can help employees work appropriate hours and maintain a healthy work-life balance, which translates into a more positive and productive workforce. Analyzing the times when the most requests come in will help create a schedule to minimize the waiting time and alleviate the need for overworking.
Efficient and user-friendly data retrieval systems enable employees to perform their tasks effectively. When employees have quick and easy access to necessary information, they can resolve customer queries faster and more accurately. Streamlined processes enable employees to focus more on customer interactions and less on navigating bureaucratic hurdles, enhancing the overall quality of service.
Read more: Retail business intelligence: step by step implementation
WHITE PAPER
Explore how to leverage Business Intelligence in ecommerce for sales growth – get the guide now!
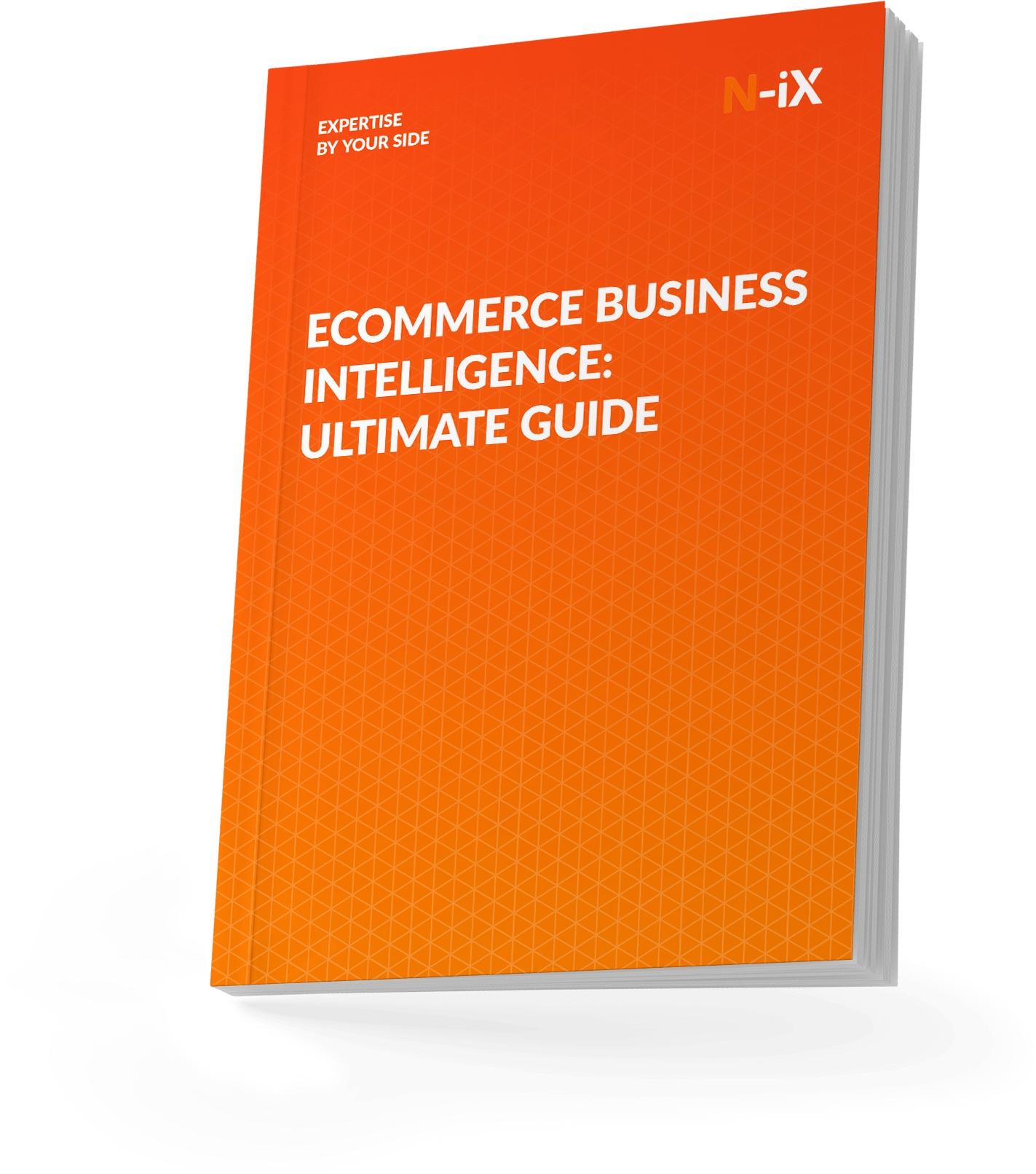
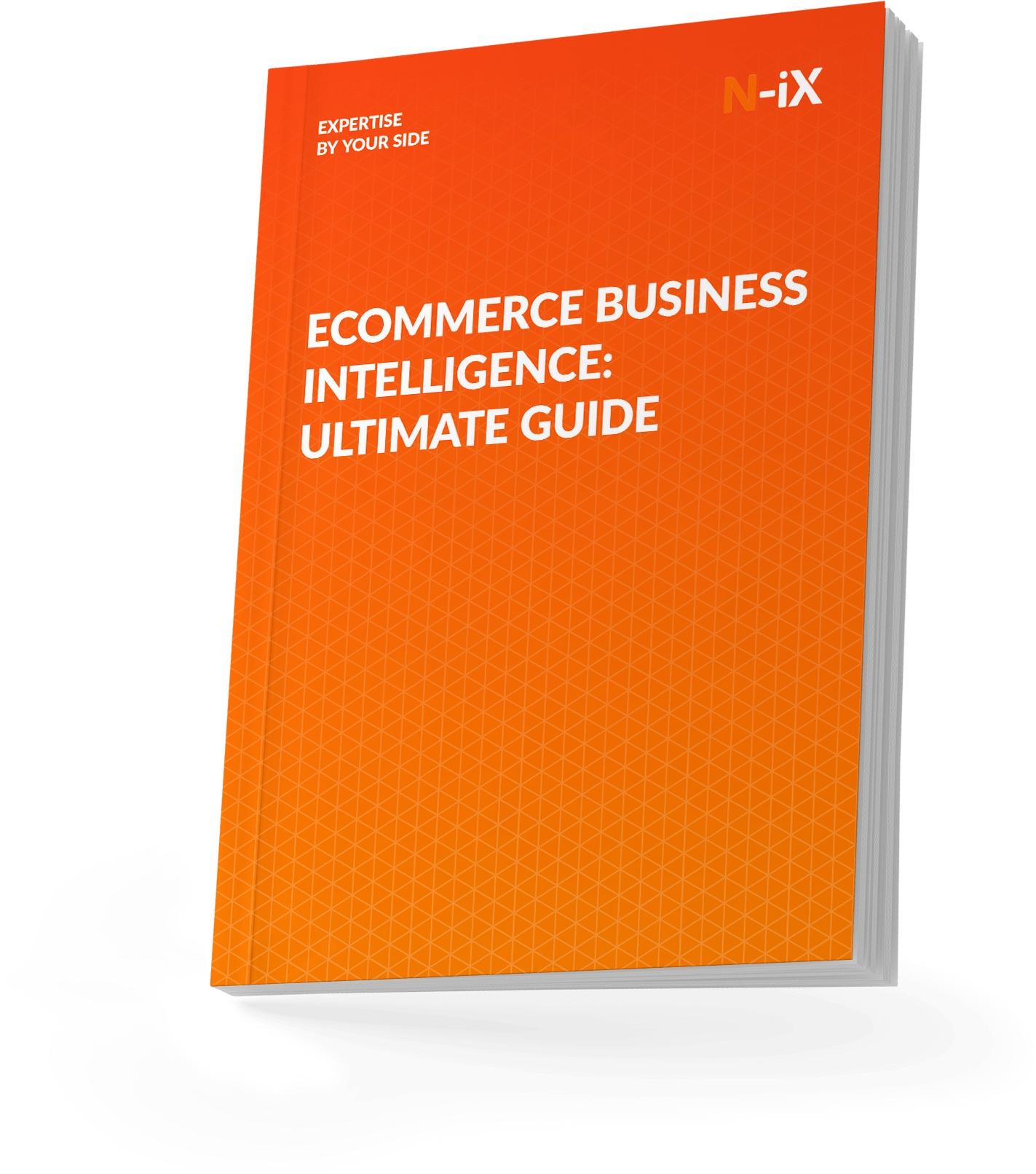
Success!
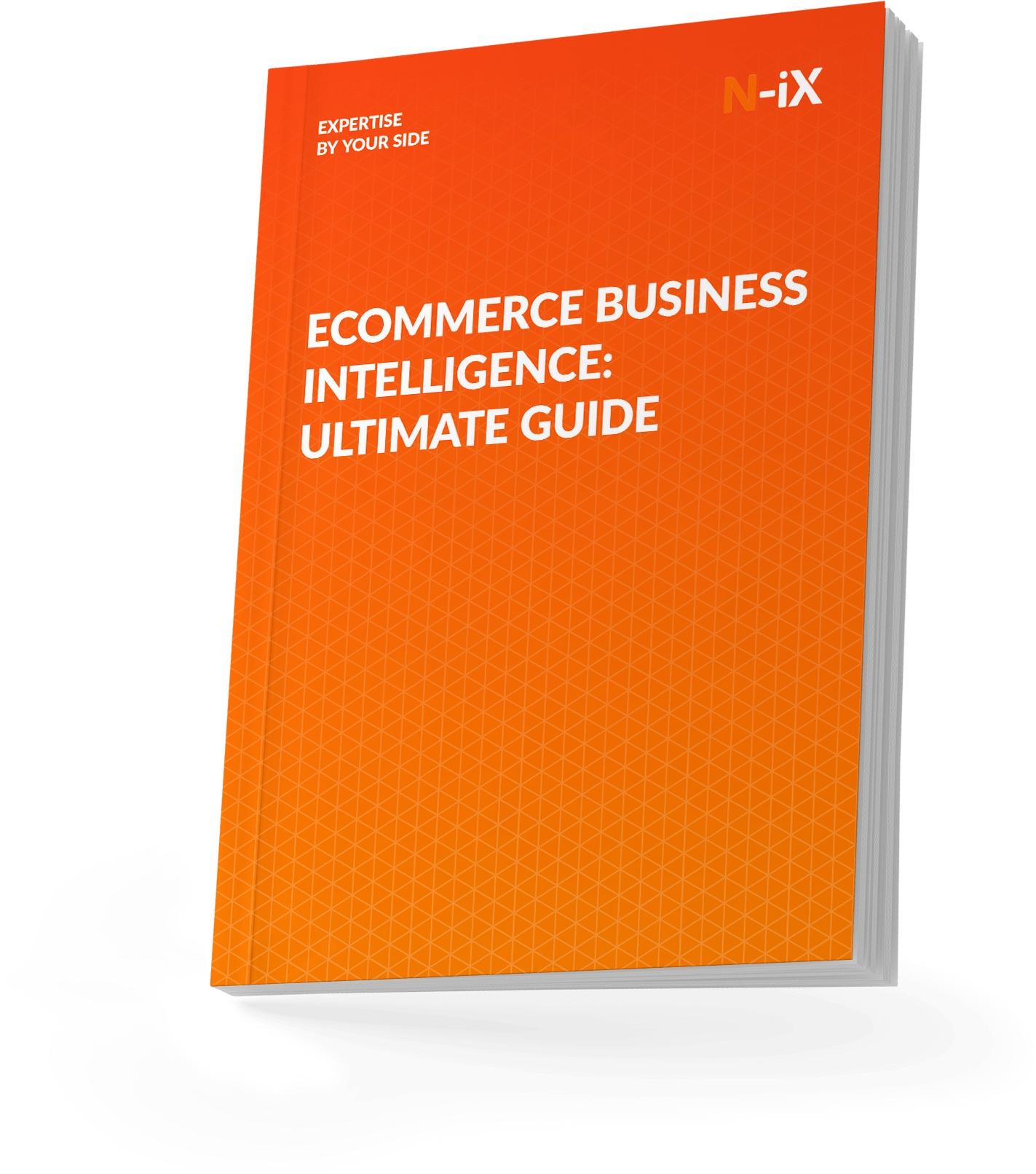
First response time
Time-to-resolution analysis can also determine which steps involve repetitive or low-complexity tasks that can be automated. There are several ways the first-response time can be improved depending on the origin of the delay:
- More comprehensive self-service: Providing enough instructions for the customers to handle the most common issues themselves can significantly lessen the load on operators. Creating tutorials, how-to guides, and webinars based on frequent questions can enhance customer knowledge and usage of the product, leading to better customer experiences and reduced support costs.
- Data flow integration: When customer service agents have immediate access to comprehensive customer data, including previous interactions, purchase history, and preferences, they can quickly understand the context of the inquiry and respond more efficiently.
- Delegate initial states to an AI bot: It is not always true that customers prefer to speak to a human; 74% of online shoppers say that they would feel more comfortable asking simple questions of a robot. AI bots are incredibly proficient in analyzing relevant customer data and handling the majority of queries. More advanced issues that do not fit within a typical pattern can then be addressed by a human operator. Automating this initial step of query resolution with an AI chatbot can decrease first-response time to seconds.
Customer effort score
For a smooth customer experience, operators should have all the necessary customer information at hand so customers don't have to repeat themselves or search for it. The Customer Effort Score represents how many actions a customer must take to resolve an issue while speaking to an operator. The smaller the score, the better. The score can be determined by asking customers how easy it was to resolve their issues in a form or by using NLP tools to analyze conversations for action points.
Identifying channels with the highest Customer Effort Scores can pinpoint areas that are negatively impacting customer satisfaction. For instance, if a high effort score is linked to agents lacking information from a particular channel, integrating this channel's data into the systems used by agents can streamline the process and reduce customer effort.
In conclusion, leveraging modern customer service analytics and optimizing KPI review frequencies can significantly enhance the ability to detect and address issues promptly. This proactive approach not only minimizes potential customer loss but also improves service quality, operational efficiency, and overall customer satisfaction.
N-iX’s take on customer service analytics
Customer service analytics fits in the context of the shift from intuitive to data-driven decision-making. Implementing big data tools will highlight the critical aspects of customer service that need attention. They reveal the most common customer requests and issues with the product, enabling the business to make informed and targeted enhancements. Retailers leveraging customer service analytics as part of their digital transformation strategy gain a competitive edge. They can offer superior customer experiences, make informed decisions quickly, and adapt to market changes with more agility compared to competitors.
At N-iX, our 200 data experts are experienced in helping businesses build effective data management systems to make the most out of the wealth of data they collect. We excel at each step of the data management systems: designing a data strategy, building a data warehouse, creating data platforms, and setting up data analytics.
Read more: Driving growth in ecommerce with a comprehensive data analytics solution
References:
- Customer Service Trends for 2022, Stella Connect
- State of the Connected Customer Report, Salesforce 2023
- Customer Service Excellence Survey 2023, Deloitte 2023
- Ibid.