By 2023, 40% of enterprise applications will have built-in conversational AI capabilities. However, many businesses struggle to implement these solutions correctly and often do so without a practical strategy. This leads to scalability issues and inconsistent support quality, ultimately leading to low customer satisfaction.
To overcome these challenges, enterprises need a well-defined conversational AI strategy. Such a strategy guides the integration and optimization of AI solutions to meet business and customer needs.
At N-iX, we understand the complexities involved in designing conversational AI solutions effectively due to our 21 years AI, ML and Data Science expertise. In this blog post, we'll delve into the essential components of a conversational AI roadmap, drawing on our AI experience.
What is conversational AI strategy?
A conversational AI strategy is a comprehensive plan that outlines how an organization will deploy conversational AI technologies, such as chatbots, virtual assistants, and voice-activated systems. This plan involves knowing all the possible uses and the technical, operational, and ethical aspects of conversational AI.
When defining goals, organizations should consider several key questions:
- If the primary goal is to offer better customer service, how can conversational AI provide faster and more accurate responses to customer inquiries?
- If the objective is to reduce operational costs, how can conversational AI automate repetitive tasks and free human resources for more complex issues?
- If the aim is to increase user engagement, what strategies can be implemented to ensure conversational AI interactions are engaging and meet user needs?
- If the focus is generating leads and increasing sales, how can conversational AI nurture leads through personalized interactions and drive sales conversions?
- If the goal is to drive innovation, how can conversational AI be integrated into developing new products or services to offer unique value propositions?
Conversational AI roadmap strategy
At N-iX, our long-term experience designing and implementing conversational AI strategies for various clients has allowed us to develop a robust and comprehensive roadmap.
Understand current AI readiness
Our process begins by evaluating your organization's current capabilities and preparedness for adopting and implementing conversational AI technologies. Key focus areas include reviewing the existing IT infrastructure, assessing data quality and management practices, and evaluating staff skill levels. This thorough assessment helps identify gaps and areas for improvement, ensuring a solid foundation for your AI journey.
Define a vision for AI adoption
Next, we work closely with stakeholders to outline specific technologies and their proposed applications within your organization. Each use case outlines conversational AI's application and the expected outcomes. Our strategy includes the necessary resources, team composition, financial budget, and infrastructure for successful implementation. Additionally, we provide a clear roadmap for deployment and a framework for assessing the impact of AI-powered initiatives.
Conduct Proof of Concept (PoC)
Implementing initial pilot projects is crucial for validating the feasibility and effectiveness of conversational AI solutions. These projects help identify potential challenges and refine the approach based on real-world feedback. PoC involves deploying AI solutions on a small scale to test their functionality and impact, identify potential challenges, and demonstrate the value of AI to stakeholders. Pilot projects extend this testing to a broader scope, gathering more data and insights.
Implement сonversational AI solutions
Our implementation phase involves selecting the appropriate technologies, designing and developing AI solutions, integrating them into existing systems, and thoroughly testing their effectiveness. Our conversational AI consulting specialists offer guidance throughout this process to ensure the technical implementation aligns with the strategic vision. We also provide project management support to oversee the implementation, ensuring it adheres to planned timelines and budgets and promptly addresses any challenges.
Manage risks and ethical concerns
We imply measures to manage risks and address ethical concerns associated with AI. Establishing data governance policies, ensuring compliance with regulations, and maintaining fairness and transparency in AI operations are critical. Addressing these concerns proactively builds trust and mitigates potential legal and reputational risks, setting a strong ethical foundation for AI initiatives. We will look closer at the data strategy below.
Ongoing support and optimization
The AI journey does not end with the initial implementation. We provide ongoing assistance, helping companies monitor the performance of their AI solutions, troubleshoot technical issues, and adapt to changes in the business ecosystem or advancements in AI technology. Our team helps refine the strategy and its implementation based on performance feedback and evolving business goals, ensuring continuous improvement and long-term success.
Read more about: Conversational AI use cases to watch and adopt
How data strategy impacts conversational AI
Last but not least, the performance and accuracy of conversational AI systems heavily depend on the quality of the data used to train them. Effective data management is critical to enhancing the performance of conversational AI. It involves systematically collecting, storing, processing, and governance of data. Here's how it impacts AI performance:
- Data collection: Effective data management starts with collecting the right data. This includes gathering diverse datasets from various sources, such as customer interactions, transaction records, and social media. Ensuring that data is relevant and representative is essential for training robust AI models.
- Data storage: Storing and managing data efficiently is crucial for accessibility and scalability. Utilizing cloud solutions and big data technologies allows organizations to handle large volumes of data seamlessly. Structured and well-organized data storage enables quick retrieval and processing, vital for real-time AI applications.
- Data processing and analysis: Raw data must be cleaned, structured, and analyzed to generate valuable insights. Techniques like ML and statistical analysis are employed to discover patterns, tendencies, and correlations within the data. This processed data is then used to train AI models, enhancing their accuracy and effectiveness.
- Data governance: Implementing robust data governance policies ensures that data is used ethically and legally. This includes maintaining data privacy, ensuring data security, and complying with regulations such as GDPR and CCPA. Good data governance builds trust with users and stakeholders and prevents potential legal and ethical issues.
Keep reading: Use cases of conversational AI in healthcare
Best practices to follow in building the practical conversational AI strategy
Leverage feedback
To ensure your conversational AI remains relevant and highly functional, use feedback from employees and customers. For instance, if support managers notice agents frequently answer a specific question, such as tracking shipments, the AI can be updated to address this issue if it is suitably flexible. This minimizes backend coding and makes improvements practical. Additionally, ensure your AI can collect and analyze feedback from various channels to continuously refine its performance.
Incorporate multi-channel integration
Build on your existing strengths by maintaining familiar interfaces and communication channels. Choose a conversational AI solution that integrates seamlessly with your current tools and third-party sources like CRMs. Multi-channel integration allows users to switch platforms without losing context, providing a cohesive and flexible user experience. Use APIs and integration tools to facilitate this interoperability.
Implement continuous learning mechanisms
Ensure your conversational AI system is capable of continuous learning. This involves setting up mechanisms for the AI to collect data from each interaction, improving its responses over time. Use reinforcement learning and active learning techniques to refine the AI's performance. Continuous learning helps the AI adapt to new queries and maintain high accuracy and relevance.
Prioritize ethical AI practices
Adopting ethical AI practices is crucial for building trust and ensuring regulation compliance. Develop a framework to address bias, transparency, and accountability issues. Implement measures to audit AI decisions and outputs for fairness and inclusivity regularly. This not only ensures ethical compliance but also enhances user trust and acceptance.
Design for scalability
Considering the rapid advancements in AI technology, an AI strategy should prioritize scalability and adaptability. Organizations must proactively plan how to expand their AI initiatives as capabilities and needs grow. Additionally, they should remain agile, ready to integrate new developments in AI technology or adjust to shifts in the business environment. This flexibility ensures that AI solutions stay relevant and effective over time, enabling businesses to continuously benefit from AI advancements.
Read more: Current use cases of conversational AI in insurance
WHITE PAPER
Explore how to use Conversational AI in eCommerce to boost engagement and drive sales – get the guide now!
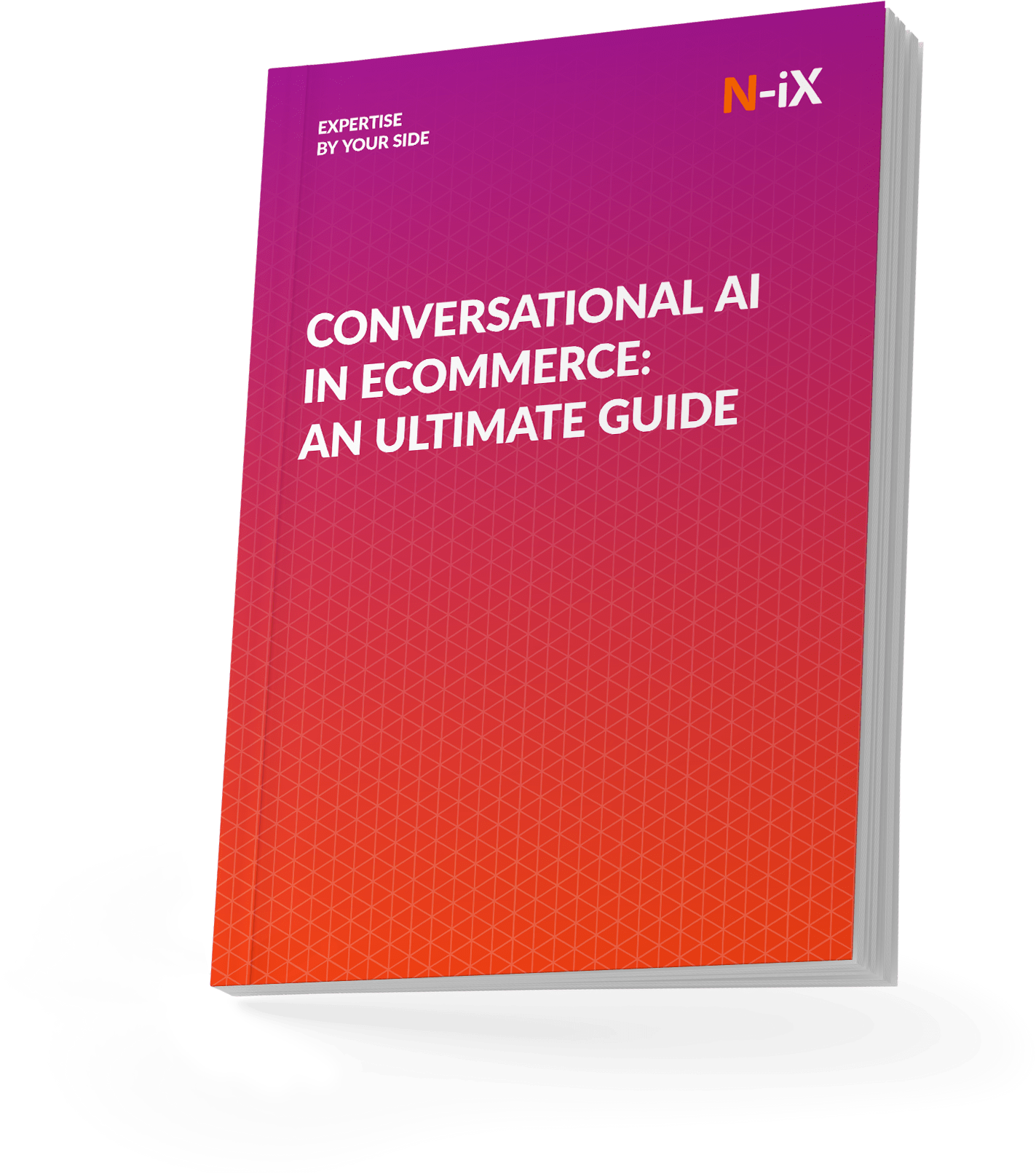
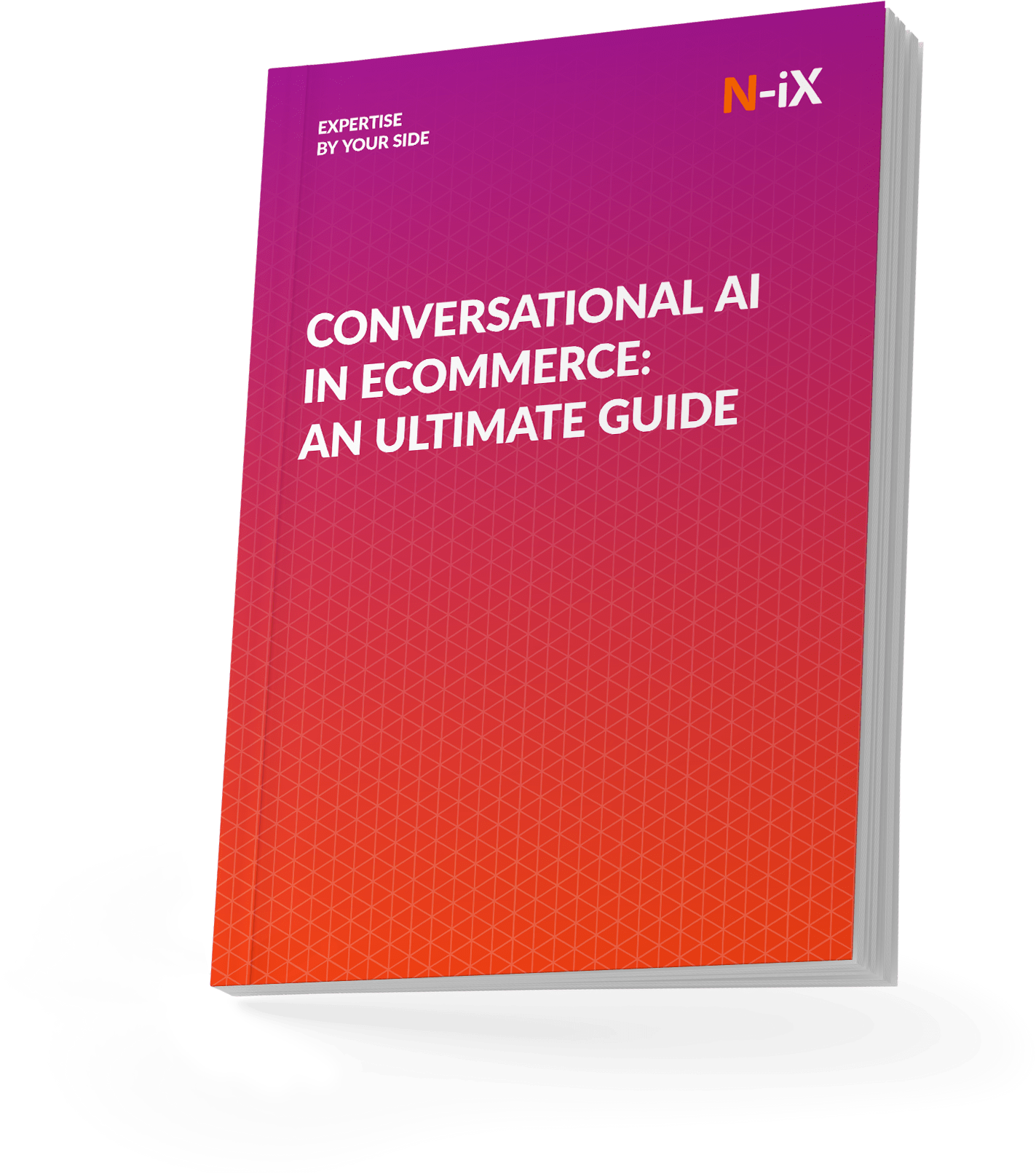
Success!
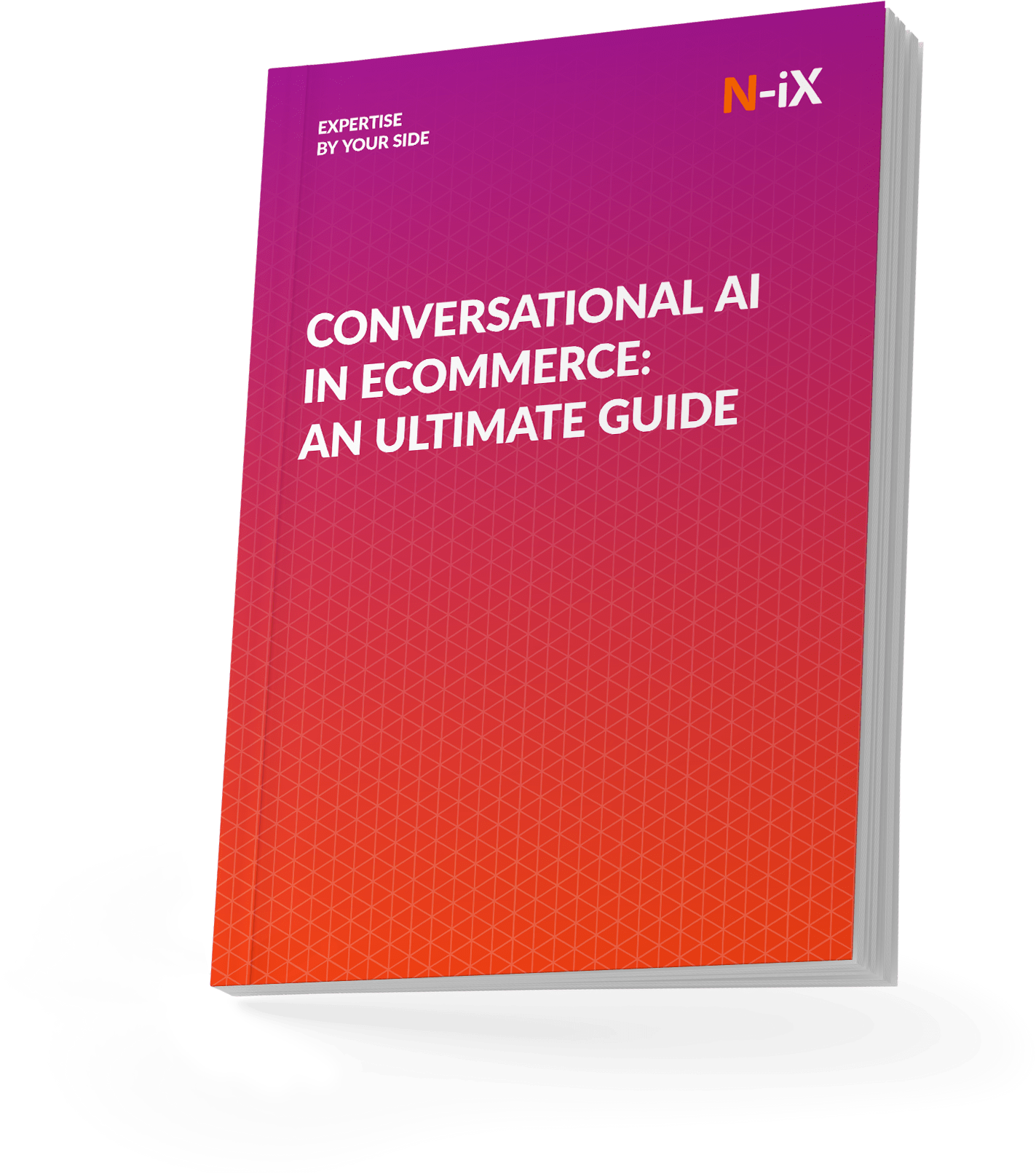
N-iX success story: Designing a conversational AI solution
Nixie, an advanced AI chatbot, exemplifies how a well-crafted conversational AI roadmap can transform customer engagement and sales processes. Powered by OpenAI and LlamaIndex Library, Nixie is designed to provide real-time, accurate information.
We delivered key features that enhance its functionality and user experience:
- Seamless integration into websites or applications
- Customized design to match brand aesthetics
- Multilingual and voice-responsive capabilities
- User-friendly, human-like conversation interface
- Integration with CRM systems for streamlined lead management
- Tailored monitoring system for gathering valuable insights
Provided the following value:
- Nixie provides AI-driven, personalized responses to customer inquiries, improving interaction quality and satisfaction.
- Features like AI-driven suggestions, dynamic content adaptation, sentiment detection, and smart escalation ensure that customers receive timely and relevant support.
- Automated data entry into CRM, automated lead scoring, behavioral insights, and instant profile updates ensure businesses can optimize strategies based on real-time data.
Final thoughts
Adopting a strategic approach to conversational AI is essential for businesses aiming to leverage this technology effectively. A well-defined strategy ensures conversational AI initiatives align with broader business objectives, optimize resource utilization, and maintain flexibility for future scalability.
Contact N-iX for a consultation on conversational AI strategy. Our experts will guide you through the process, from assessing your current capabilities to implementing robust AI solutions that drive business success.
- We have a dedicated team of over 200 data and AI experts with extensive experience in Big Data, AI/ML, legacy migration, Data Science, and Data Analytics.
- Our expertise spans AI and ML, Cloud Solutions, DevOps, Intelligent automation, IoT and embedded development, and more.
- We have a proven track record of building and implementing successful AI-powered solutions for Fortune 500 companies. Our clients include Gogo, Lebara, Cleverbridge, and others who have praised our professionalism and skill set.
- N-iX is committed to maintaining high industry standards and adheres to PCI DSS, ISO 9001, ISO 27001, and GDPR.
Have a question?
Speak to an expert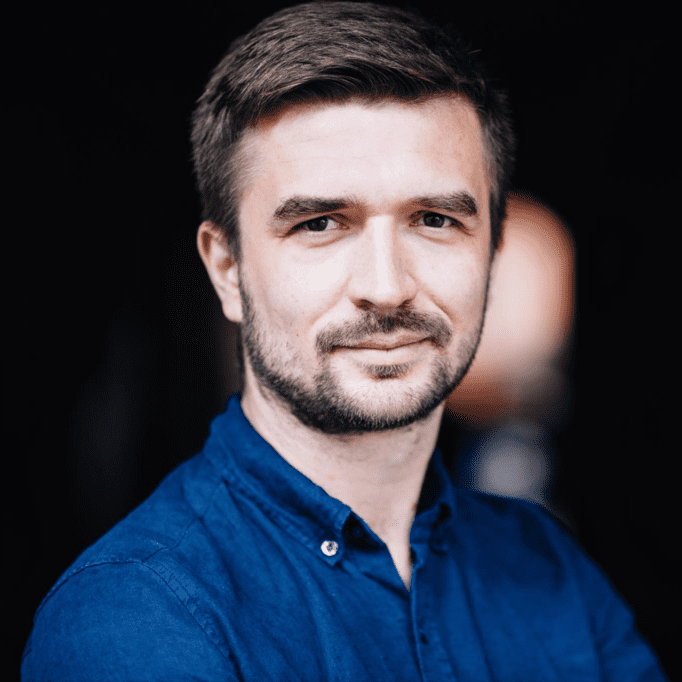