The customer’s experience interacting with the support line might determine whether the insurance company will be seen as a trusted partner in times of crisis or an adversary. Investing in a positive customer experience is crucial for long-term success. The market value of AI in insurance is expected to reach $36B by 2026 from $4.59B in 2022 [1 & 2]. Almost half of that growth is explained by conversational AI in insurance customer support and claims adjusting. There is further evidence of the coming industry transformation in the increasing demand for gen AI consulting and ML experts among insurance firms. According to Deloitte [3], 74% of surveyed insurance companies plan to increase their budgets for AI investment, with the highest priority being given to AI-powered chatbots.
Conversational AI systems are more than just chatbots with a language interface. They can understand context, handle complex situations, and adapt their responses to meet user needs.
Let's explore how conversational AI in insurance is used to save time for both customers and insurers and foster trust between them.
Use cases of conversational AI in insurance
The goal of conversational AI in insurance is not to completely replace human communication but to enhance it. Many customers contact their insurance provider during a stressful situation, which limits their patience for frustrating chatbot interactions. Receiving compassionate and efficient treatment, whether from a human agent or an AI, is particularly important. A report by Deloitte projects that empathy will become the key value in the insurance industry with the rise of automation. This is especially true in health insurance –83% of healthcare organizations have already implemented an Artificial Intelligence strategy, and more are developing one [4].
1. Claims handling
Settlement speed is one of the biggest reasons for customer dissatisfaction and churn. Conversational models assist customers in filing claims, staying informed, and receiving real-time updates on the claims process. Automating routine tasks expedites claims processing, reduces paperwork, and enhances the overall claims experience for customers.
AI language models can also utilize their conversational abilities to automatically read, interpret, and process relevant documents and even photographs. This includes tasks such as extracting necessary information from medical records or identity cards, recognizing vehicle types, and assessing damage.
Customers can receive all the necessary information about their particular claim when they need it. Conversational AI can mitigate the inconsistencies often associated with human claim assessments, which can result in significant disparities between customer expectations and actual evaluations.
2. Automated after-call work
Conversational AI can generate reports of its conversations, saving hours of after-call work. It can also be used to create a report for a phone call made by an employee. It automatically transcribes the call and logs detailed information about customer interaction, such as the call duration, issues discussed, resolutions provided, and any follow-up actions required.
By radically optimizing administrative tasks, agents can prioritize delivering quality service to customers without the need to simultaneously complete reports. This increased efficiency not only enhances the overall customer experience but also improves the ability to handle more inquiries.
3. Customer query resolution and personalization
Conversational AI-powered chatbots excel at resolving customer queries and providing immediate assistance. They can answer common questions about policies, premiums, coverage details, and claims processes. Some models can also analyze the tone of speech to determine whether a customer requires a brief answer or a detailed explanation.
Beyond that conversational AI can analyze customer data and provide personalized policy recommendations based on individual needs and preferences. Chatbots can offer tailored recommendations to customers factoring, among other variables, age, income group, risk profile, and job stability, improving cross-selling and upselling opportunities.
For example, in healthcare, McKinsey suggests that Medicare Advantage plans are missing out on $2.9B around Stars-related payments*, due to suboptimal member experiences. Personalization in AI-powered chatbots can help address this issue by tailoring interactions to individual members, ensuring better engagement, and adherence to health programs, and ultimately improving the overall Stars rating, which can result in higher payments and revenue for health plans.
4. Fraud detection
Conversational AI can play an essential role in fraud detection and prevention. Through analyzing customer interactions, chatbots can identify patterns and anomalies indicative of potential fraudulent activity. Insurers can leverage this data to flag suspicious claims, investigate further, and mitigate risks associated with fraudulent activities.
A benefit of using conversational AI for fraud detection is its ability to monitor customer interactions in real-time. For example, during a live chat session with a customer, the AI can assess the responses and flag any signs of potential fraud. Conversational AI can be integrated with other data sources within an insurance company's ecosystem. This includes policyholder data, historical claims records, and external databases with fraud-related information. By cross-referencing customer interactions with this data, chatbots can provide a more comprehensive fraud analysis.
5. Distribution and sales
Insurers can provide instant quotes and policy issuance with conversational AI, simplifying the customer acquisition process. A quick automated conversation eliminates the need for lengthy application forms and manual underwriting processes, making insurance more accessible and convenient for customers. Instant quotes and policy issuance also enable insurers to capitalize on customer interest and convert leads into customers more efficiently.
The same AI-powered assistants can also provide agents with real-time access to customer data, policy information, and product recommendations. This enables agents to serve customers more efficiently. These assistants can also help agents manage tasks like scheduling appointments, generating quotes, and processing policy changes.
Developing an AI implementation strategy
AI is undoubtedly going to revolutionize many insurance businesses in the next decade. By following best practices, insurance companies can avoid making hasty decisions to implement trendy technology and instead maximize the competitive advantage created by AI. Here are some important points to consider when developing a conversational AI implementation strategy.
Managing both customer and employee expectations
Clear communication and education regarding the capabilities and limitations of AI-powered systems are crucial to avoiding customer frustration and employee malpractice. Insurers should clearly outline policies that explain the role of AI in various company processes, like underwriting, claims handling, and customer service.
For example, the customer should be made aware of whether the conversational AI is simply predicting the conditions and the amount of compensation for their claim, or if the AI is making a decision regarding it when it communicates this information. Failing to set realistic expectations for what conversational AI entails can lead to cases of deceit and violation of regulations.
From the agent’s perspective, understanding what an AI system can and cannot do leads to a more effective use of it. For example, AI assistants are not capable of operating in uncharted territory like rare medical conditions or highly nuanced situations. Using AI to assess such claims might lead to mistaking AI hallucinations for real answers. However, AI saves hours on routine tasks and common cases.
Reworking the existing systems
Insurance companies typically have a complex IT infrastructure, comprising legacy systems and a mix of modern applications. Ensuring seamless integration of conversational AI with these diverse systems is essential for its functionality. The integration needs to enable real-time data exchange and provide access to the necessary databases for claim processing, customer relationship management (CRM), and policy management. Working with a Conversational AI consulting company for implementing conversational AI in insurance might open an opportunity for streamlining and enhancing all the company’s systems.
Read more: AI strategy consulting: An in-depth guide
Regulatory compliance and transparency
To meet the increased demand for data created by conversation AI, insurers have to create and implement a data strategy policy. This policy should cover the collection, storage, processing, and use of customer data, ensuring compliance with regulations such as HIPAA in the United States.
The chosen data infrastructure provider should have strong data encryption measures, clear data access and breach notification policies, and compliance with international standards such as ISO 27001 for information security management.
Providing high-quality data
The effectiveness of a conversational AI system relies on the quality and relevance of its training data. Insurance companies deal with vast amounts of data, but this data can be unstructured, outdated, or inconsistent. Poor data quality can lead to inaccurate responses from the AI, potentially damaging customer trust and satisfaction.
Additionally, there is inequality in modern societies that is reflected in the data used to train the models. When interacting with minority clients, certain conversational AI models may suggest discriminatory pricing or provide less accurate health information. Insurers must conduct thorough audits of their data to identify and correct biases and implement strategies for equitable data collection and AI model training. This involves engaging with diverse teams and external experts, regularly testing for fairness, and providing ongoing employee training in recognizing and mitigating bias.
Conclusion
It is an increasingly realistic scenario for a homeowner to swiftly manage a burst pipe incident by taking a few photographs of the damage and sending them to an AI assistant. The AI would then assess the extent of the damage and, within the same brief text exchange, schedule the earliest available appointment with repair services.
EBOOK
Get your ultimate guide on Generative AI use cases and applications
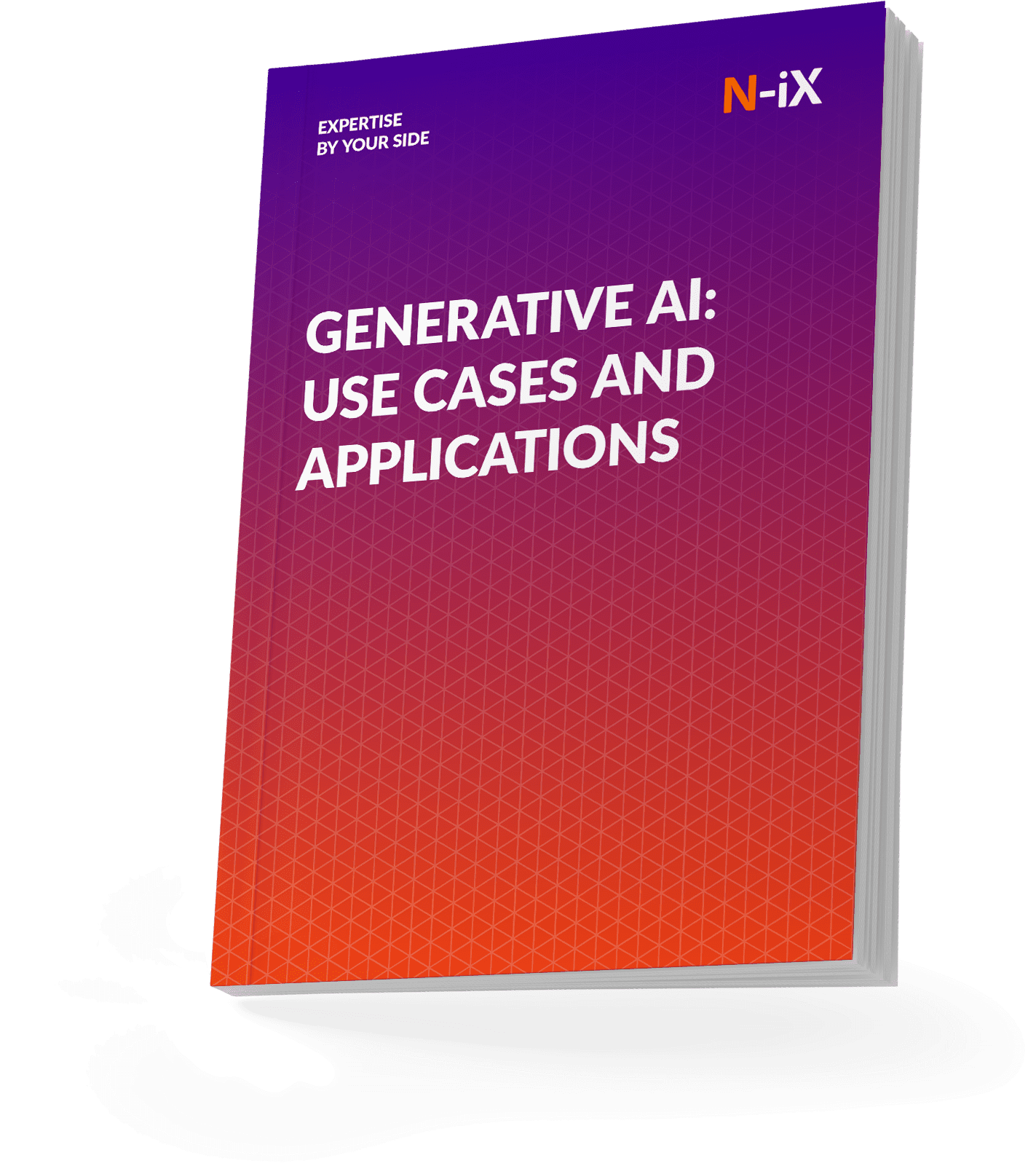
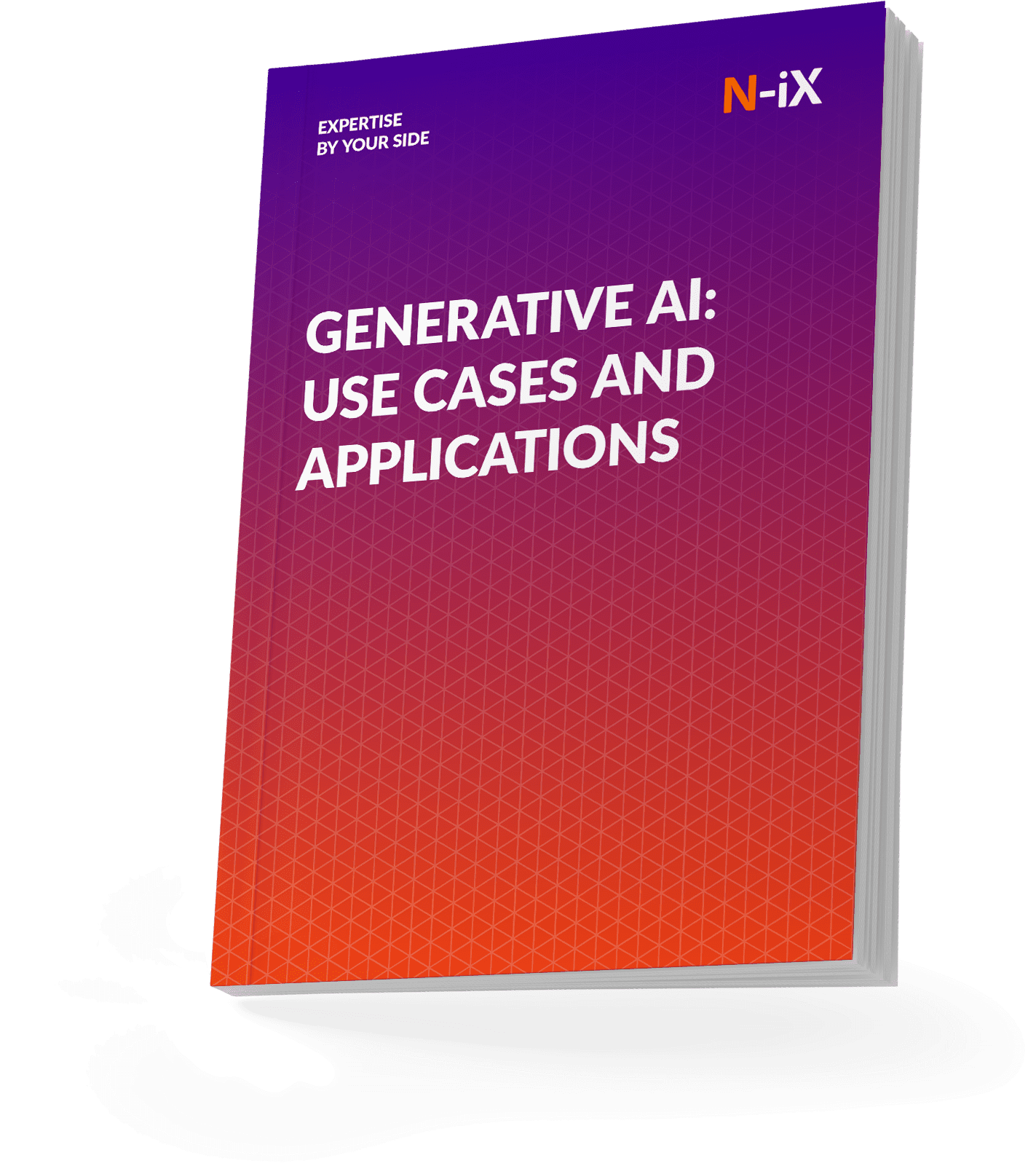
Success!
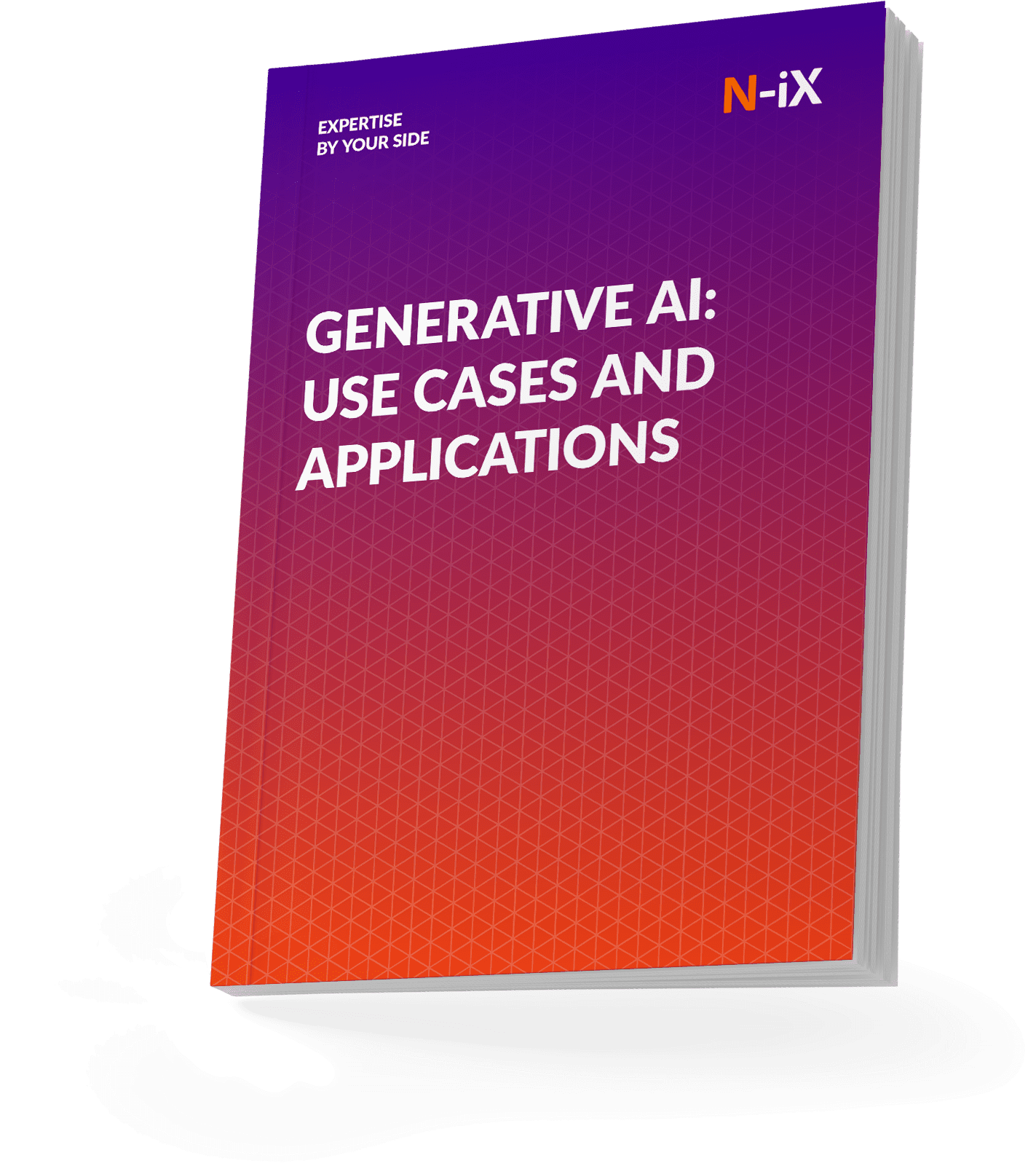
Why talk to N-iX experts about your conversational AI implementation
- N-iX houses a team of over 200 Data, AI, and ML experts.
- We have delivered over 160 data science and AI projects for industry pioneers and Fortune 500 companies.
- Our engineers have a wide range of data expertise, including data migration strategy, Machine learning and AI implementation, big data strategy design, and more.
- We keep data protected by complying with established security standards such as ISO 27001:2013, ISO 9001:2015, GDPR, and PCI DSS.
References:
* Stars-related payments in Medicare are financial incentives or penalties provided to healthcare providers and Medicare Advantage plans based on their performance in delivering care to beneficiaries.
1. Mantra research: AI chatbots in insurance report, 2020
2. Artificial Intelligence (AI) In Insurance Market Size, Report 2023-2032
3. 2024 global insurance outlook, Deloitte Insights
4. “Third Annual Optum Survey on AI in Health Care,” Optum, October 2020.
Have a question?
Speak to an expert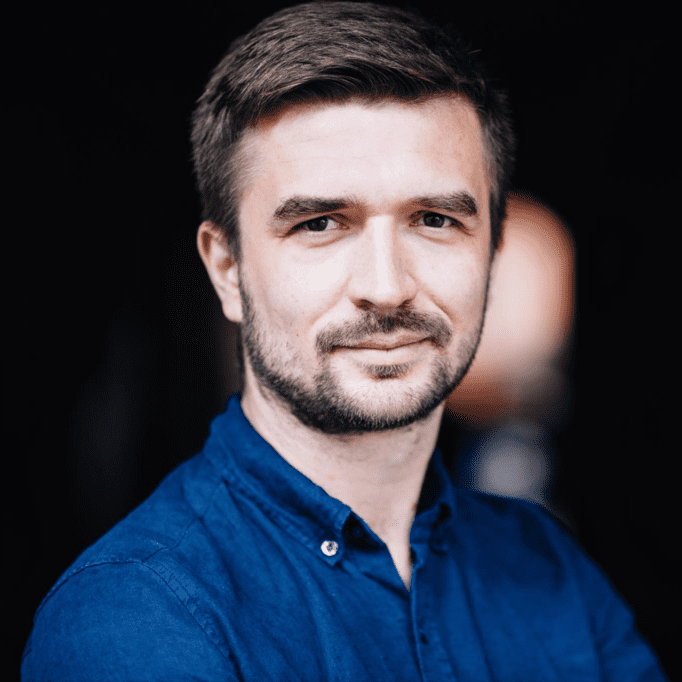