The nature of ecommerce businesses allows them to collect a wealth of data and analyze using big data tools to understand many aspects of the business. One such aspect big data analytics shed light on is the sales funnel. Previously just a marketing concept, advanced ecommerce software development can transform the sales funnel into a tangible process that retailers can measure, understand, and optimize.
Monitoring metrics such as the number and position of clicks per page, shopping carts, and the typical duration of a page visit, plus demographic details, can put together a realistic picture of each step in the funnel. Understanding each drop-off point shows the areas that could benefit from targeted improvements, optimize marketing strategies, enhance user experience, and ultimately increase conversion rates and revenue. Let’s look at each step in the sales funnel and see how big data analytics in ecommerce can explain lost customers.
Learn more about our ecommerce consulting services
How big data analytics can explain lost leads
Understanding why customers abandon their journey down the sales funnel is the first critical step toward making effective changes to retain them. Tailoring and personalizing marketing, enhancing CRM processes, and implementing loyalty programs, discounts for returning customers, and subscription services are all potential solutions for customer retention.
However, their effectiveness can vary significantly based on whether they are applied to the correct issue. For example, while implementing a loyalty program might boost long-term engagement among frequent shoppers, it could be less effective if the primary issue is poor customer service, which needs to be addressed first. Identifying the problem through data analysis is crucial to selecting and applying the most appropriate strategy for retaining customers.
Aware, but not clicking
Big data analytics in ecommerce arms marketers with the insights needed to run more personalized, efficient, and responsive campaigns. Demographic, behavioral, transactional, and engagement data can be pulled together to create accurate and detailed customer profiles. For example, suppose the data reveals that a particular segment of customers frequently purchases a specific type of product and responds well to email marketing. In that case, the campaign automation tools can be programmed to send targeted offers or content related to that product via email to this group.
A more detailed approach to targeting and segmentation, enabled by big data, provides a particular advantage for online retailers. In this highly competitive environment, customers look for businesses that reflect their values and interests. Catering to these diverse sentiments can increase the effectiveness of the marketing campaigns and build positive awareness. Big data also enhances the efficiency of marketing budget allocation. Understanding which channels and tactics yield the best ROI allows businesses to allocate their budgets more effectively, investing more in high-performing strategies and cutting back on those that underperform.
When a user visits the website, big data analytics can analyze behavioral data to understand what stops the user from viewing products. There may be various reasons for the user leaving the website prematurely:
- Loading time: If users leave within seconds of opening the website, it could be because the landing page takes too long to load. Therefore, improving the loading speed can help retain more users in the funnel.
- Ambiguous advertisement or naming: Big data analytics in ecommerce can help identify users who didn't find what they were looking for on the website. For instance, if a significant number of UK users search for "jumper," get results for sleeveless dresses (the US meaning of "jumper"), then leave, this pattern suggests a discrepancy between what users search for and what they see. This is an exaggerated example, but how often are you trying to figure out what the item you are searching for is properly called? The solution is to adjust marketing campaigns based on location or other determining factors to reduce ambiguity.
- Insufficient search engine accuracy: Most customers, about 69%, skip browsing entirely and head straight to the search bar on an ecommerce website [1]. They will leave the website if they don’t find what they were looking for on the first try. Implementing tools like semantic search can help connect more customers to their desired items, even if their queries aren’t precise.
Read more: NLP in ecommerce use cases
- Difficult user path: If the user journey looks like they are traversing a maze until they give up and close the tab, this indicates big problems with the UI. Simplifying the user journey from landing to items can boost customer retention and improve satisfaction.
Considering, but not converting
Big data analytics tools can find patterns in the groups of customers who make a purchase vs. those who don’t. These explanatory variables can be related to location, age, socioeconomic status, interests indicated from previous search history, and more. The groups that aren’t converting might require a different version of the product. On the other hand, this group might be falsely targeted by marketing campaigns, and changes should be made to avoid advertising to this group.
Additionally, comparing product offerings, pricing, and customer experiences with those of competitors can highlight gaps in value or user experience. Big data tools can aggregate and analyze competitor data, providing insights into why customers prefer other options. Running experiments with different versions of product pages can yield data on which design, layout, or messaging elements are more effective. Analyzing these results helps businesses implement changes that increase the likelihood of purchase, ultimately enhancing customer satisfaction and boosting sales.
70% of shoppers abandon their carts without finishing the purchase [2]. Big data analytics can help determine why; there are a few possible reasons:
- Unexpected costs: Additional charges like shipping fees, taxes, or other hidden costs can surprise and deter customers at the final stage of their purchase. If many customers leave at the stage of seeing these costs, this indicates that it is the likely reason for abandonment. Segmentation can also reveal groups that are more sensitive to the extra costs. For example, out-of-state customers may face higher delivery fees than expected.
- Account creation requirement: Redirecting customers to create an account before completing a purchase can be a major deterrent, especially for those looking for a quick checkout.
- Payment issues: Limited payment options or technical difficulties during the payment process can cause customers to give up on their purchases. If the customers leave at this stage, they will most likely face problems with the payment.
- Lack of trust: Concerns about a website's security or doubts about the business's legitimacy can lead to cart abandonment. Sentiment analysis can uncover negative sentiments related to specific issues by analyzing customer reviews and social media mentions.
- Unclear return policy: If customers are unsure how to return products or if the return policy is unfavorable, they might decide against completing the purchase. Pages in the user path can show that many customers read the policies before leaving the website.
Conducting A/B tests on different elements of the checkout process can help identify pain points. For example, testing different checkout processes can reveal which method leads to higher completion rates.
Converted, but not returning
According to Business.com, attracting new customers is 5 to 10 times more expensive than selling to existing ones [3]. Furthermore, loyal customers spend up to 67% more than new customers. Big data analytics in ecommerce can process data on engagement after purchase to understand how many customers are lost at this final stage of the funnel and what exactly happened. For example, with products that offer subscription services, it is straightforward to track the duration of customer usage and make conclusions based on this data. Here are some of the potential reasons customers don’t return and how big data tools can diagnose them:
- Unsatisfactory customer service experience. Positive interactions and effective problem resolution create trust and a sense of mutual understanding, making customers more likely to return and recommend the business to others. 60% of customers state that they can only be loyal to a brand if their experience with customer service is good [4]. Tools to estimate satisfaction can range from simple feedback forms to NLP algorithms analyzing the tone and flow of conversation. Advanced models may even identify points in conversations that trigger a change in attitude, such as asking the customer for information the business should already possess.
- Need for better customer segmentation. If there is a variable that can predict customer loyalty, it often means that the product or service resonates more strongly with a specific demographic or psychographic segment. This insight allows businesses to tailor their marketing strategies and product offerings better to meet the preferences and needs of these target groups, thereby enhancing customer satisfaction and increasing the likelihood of repeat purchases and long-term loyalty.
Read more: Big Data in retail: Use cases that bring the most value
Explore how to leverage Business Intelligence in ecommerce for sales growth – get the guide now!
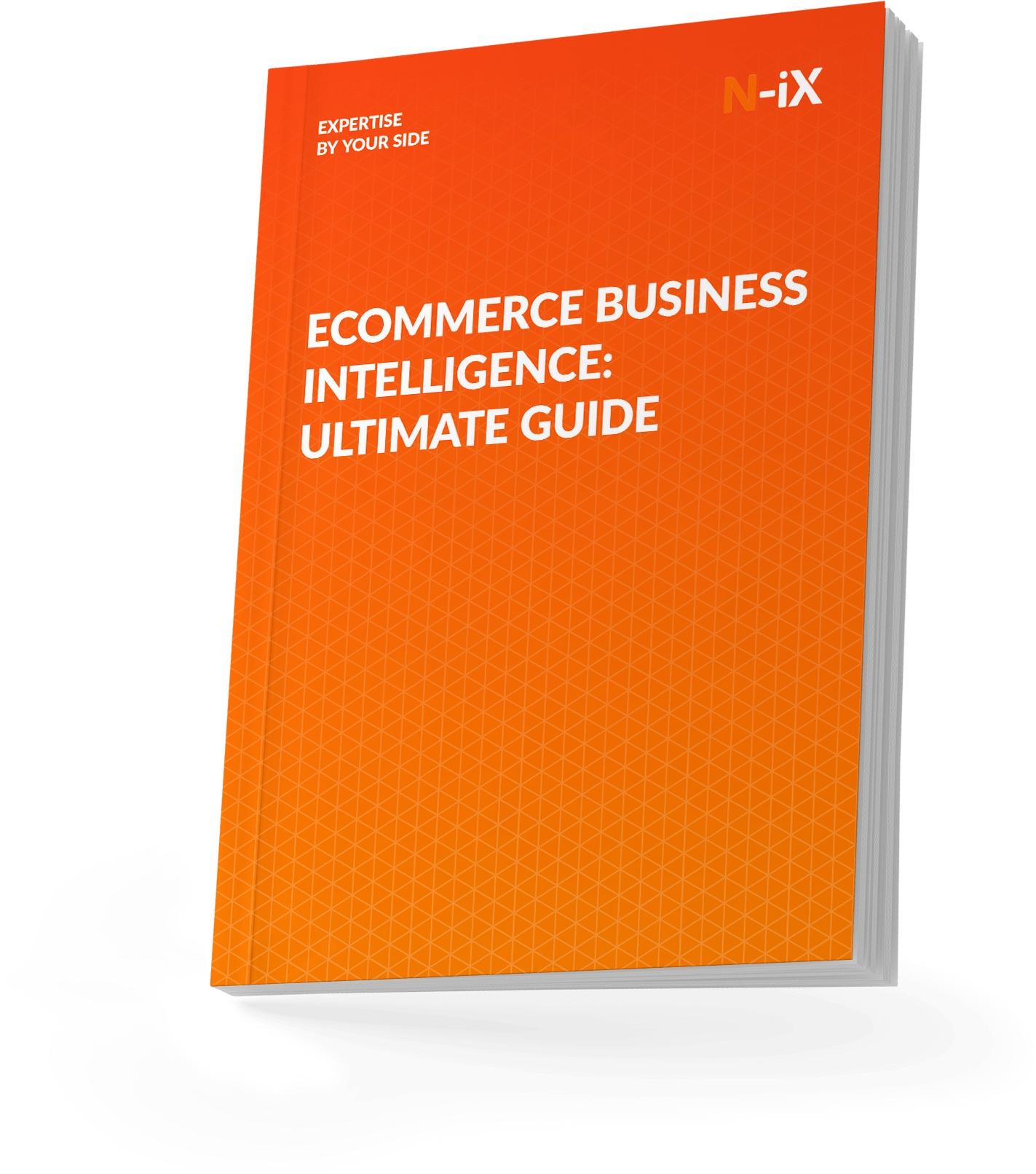
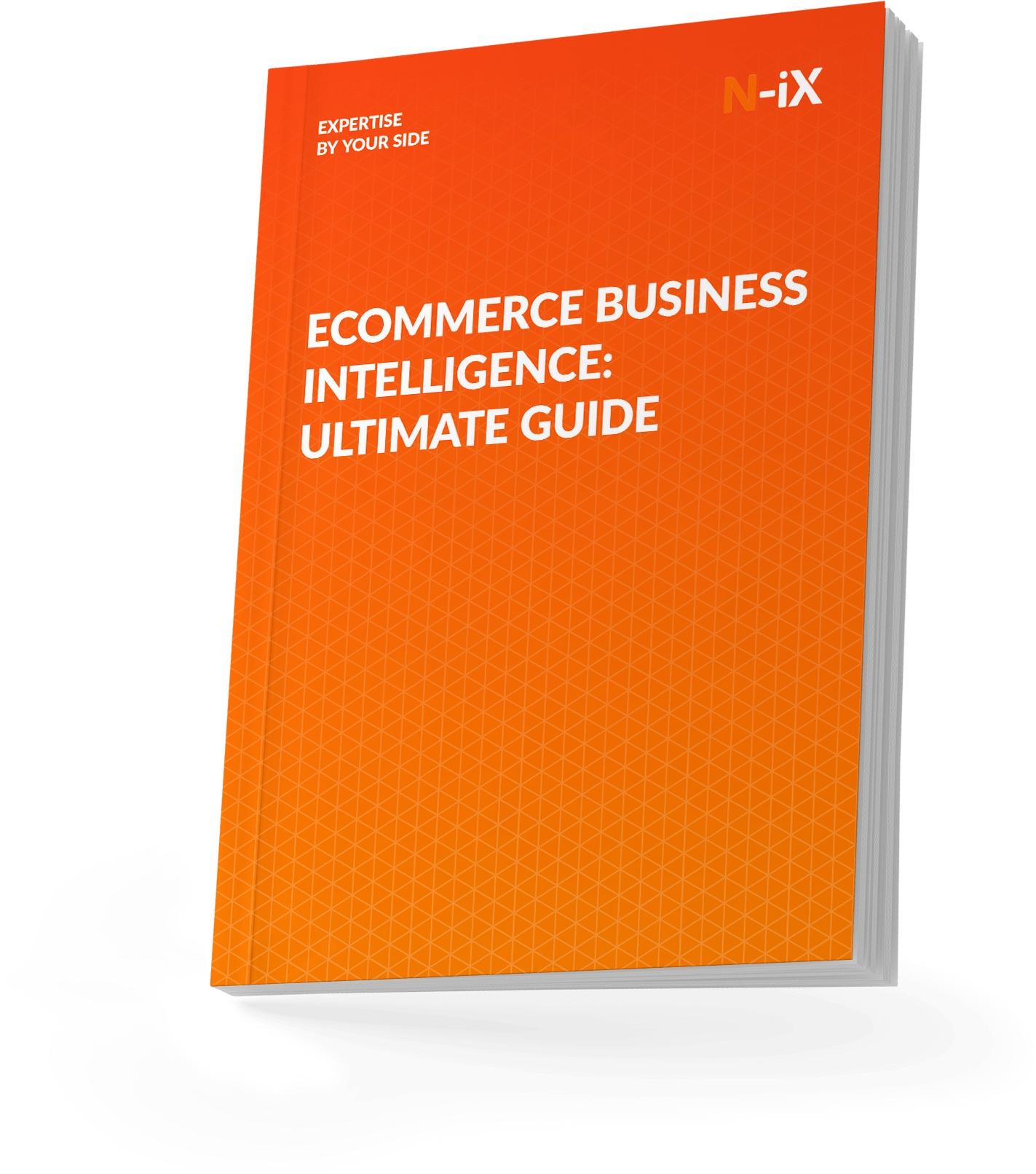
Success!
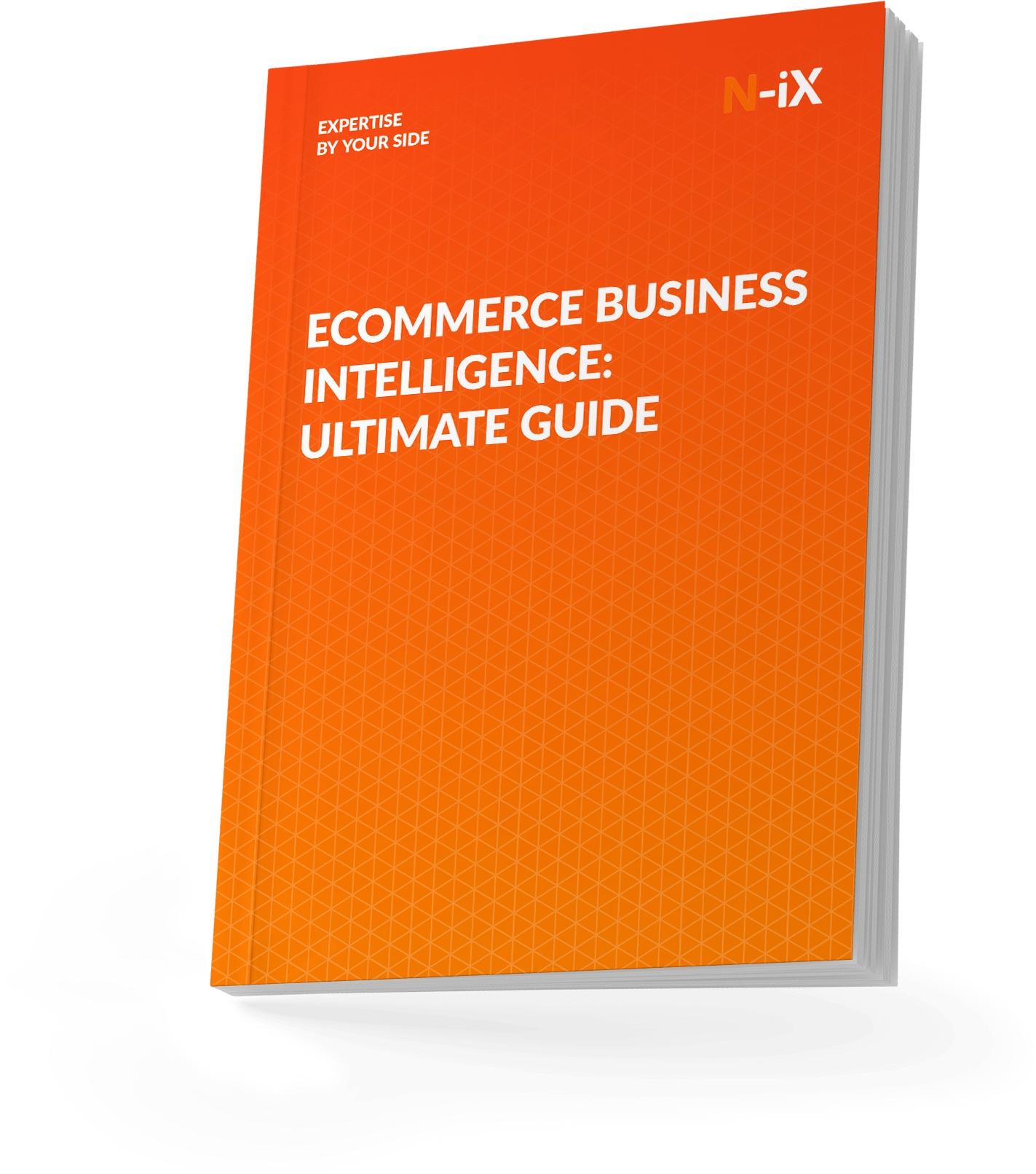
Wrap up
Comprehensive data analytics solutions are extremely valuable in ecommerce for many reasons. They empower businesses to understand and optimize their operations, consumer behaviors, and market trends. In the broader context of data management, big data analytics helps organizations transition from merely managing data to actively using it as a strategic asset. Moreover, acquiring a more advanced toolbox and implementing big data analytics can become a way to tackle data silos and improve data processing, storage, and retrieval capabilities. Experienced Big Data development services provider can guide you through the implementation process, making progress easy. Ultimately, understanding your business is the only way to target shortfalls and foster advantages.
N-iX develops comprehensive data analytics solutions in ecommerce
For over 22 years, N-iX has been helping businesses maximize the benefits of their data. More than 200 data experts at N-iX adhere to industry-leading practices and comply with security certifications such as GDPR, ISO 27001:2013, ISO 9001:2015, and PCI DSS. We create comprehensive data governance and data analytics solutions that provide valuable insights, enhance security, and allow for an increasingly convenient and personalized shopping experience.
Read more: Driving growth in ecommerce with a comprehensive data analytics solution
References:
- Nosto
- 49 Cart Abandonment Rate Statistics 2024, Baymard
- Keep Your Customers Coming Back With a Recurring Revenue Sales Model, Business.com 2024
- Customer Service Trends for 2022, Stella Connect
Have a question?
Speak to an expert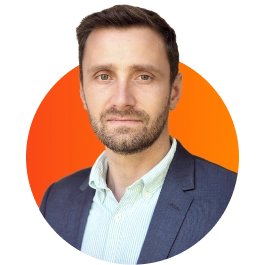