AI is no longer a distant concept for enterprises-it's a competitive necessity. The conversation has shifted from whether AI should be adopted to when and how to deliver tangible business value. However, diving straight into full-scale AI implementation without validation is a gamble. Enterprises face a real risk of expending significant resources-both time and money-on projects that may not deliver the expected results.
Too many businesses rush to implement AI without testing its relevance and viability in a real-world, controlled setting. AI, while powerful, is not a one-size-fits-all solution. Without a strategic validation phase, companies risk deploying AI solutions that don't scale, aren't cost-effective, or fail to address key business challenges.
AI Proof of Concept (PoC) can help to avoid these pitfalls. This strategic approach allows businesses to test AI models in a low-risk environment before committing to full-scale implementation. Rather than going all-in on untested AI solutions, a PoC offers a structured, low-risk approach to assess both technical feasibility and business impact. Let's explore how an AI PoC can save resources, optimize outcomes, and prepare the way for scalable AI integration.
What is AI Proof of Concept?
In contrast to a full-scale AI implementation, a PoC is a low-risk, short-term project that works as a practical experiment. While a full implementation depends on deploying AI across entire systems or operations, a PoC tests a limited aspect of AI on a smaller, controlled scale. It's a strategic move that helps businesses protect themselves from the potential downsides of jumping directly into a costly AI deployment without proving the concept's value.
The goal of a PoC AI is to validate whether a proposed AI system can meet the desired objectives and deliver measurable value before committing significant resources to a full-scale implementation. It enables enterprises to understand the technical and business viability of AI. Let's be clear: A PoC isn't meant to solve every problem or be your final product. Instead, it answers two critical questions:
- Can this AI solution work for your specific needs?
- Will it deliver measurable business value?
When is an AI PoC needed?
Not every AI project requires a PoC, but it's an indispensable step for certain business scenarios. Here are specific reasons why your AI project would benefit from starting with a PoC:
- If the business problem is not fully understood or clearly defined, a gen AI PoC is essential. A PoC helps refine this understanding, creating realistic expectations about what AI can-and cannot-achieve for your business.
- If the potential value of the AI solution is uncertain, a PoC is needed to assess whether the proposed AI solution will deliver enough value to justify the effort, time, and financial investment. This evaluation informs whether to proceed with full-scale development or rethink the project's approach.
- If there are uncertainties in the workflow or development plan, a PoC becomes necessary. Early validation prevents workflow issues from escalating during full-scale implementation.
- If there are potential limitations regarding data quality, operational constraints, or technical challenges, running an AI PoC is important. The PoC helps uncover these limitations early, allowing one to address them and reducing the risk of failure during later stages.
- If there is any doubt about the technical feasibility of the AI solution, a PoC is required. AI engineers test the AI model within your existing infrastructure and operational framework to determine whether the technology can be implemented effectively.
- If the project is high-risk, involving substantial financial investment or operational impact, a PoC is necessary. Testing AI feasibility in such scenarios reduces the likelihood of costly failures.
- If the ROI of an AI solution is unclear, a PoC helps provide clarity. By testing the AI solution on a smaller scale, enterprises can assess the financial benefits before committing to a larger rollout.
How the AI PoC development process looks like
At N-iX, delivering impactful AI solutions starts with a structured Proof of Concept process. Below is a deep dive into each crucial step in executing a flawless AI PoC.
1. Planning
Every successful AI Proof of Concept project begins with a well-defined plan. We work closely with key stakeholders to identify the business challenges AI can address and align the goals of the PoC with your long-term strategy. We discuss pain points and desired outcomes in detail with your team.
But here's what makes it special: We focus on selecting the use cases with the highest potential for impact, whether enhancing operational efficiency, reducing costs, or driving innovation. And there's more: we also assemble the right team in place, combining the expertise of data scientists, AI specialists, and industry-specific professionals to provide a holistic approach.
2. Data preparation
Data is the core component of any Artificial Intelligence and Machine Learning development initiative. The success of a gen AI PoC depends on the quality, relevance, and completeness of the data used. Our team dedicates substantial effort to data collection and preparation, making sure the datasets for model training accurately reflect the problem at hand.
Data engineers gather data from various sources and clean it to remove inconsistencies, fill in missing information, and structure it for optimal AI processing. The goal is to provide the AI model with clean, meaningful data to generate actionable insights. Data quality directly affects the performance of the AI model, making this a critical phase in the PoC process.
In a recent project with Gogo, a global in-flight internet provider, data preparation was key in improving predictive models for antenna failures. Our team collected vast amounts of in-flight performance data, cleaned and structured it to maintain accuracy, and used it to train AI models. By preparing high-quality, relevant data, we were able to develop models that predicted antenna failures with over 90% accuracy, significantly reducing downtime and improving overall customer experience. Read how we managed to achieve these outcomes in the case study.
3. Model development
Once the data is ready, the next phase begins with selecting the most appropriate AI model based on the business objectives identified during planning. At N-iX, we tailor the model selection to each client's unique needs, addressing the challenges outlined.
Whether the solution requires machine learning, deep learning, or natural language processing, our team evaluates different models to find the best fit. Model training follows, where we iteratively refine the model to learn from the data, continuously improving its performance to meet the defined benchmarks.
For one of our ecommerce clients, we conducted a PoC to test the integration of Machine Learning for churn prediction. During this phase, our team focused on data collection, cleansing, and preparation to maintain the highest data quality. We built a prototype to test the viability of churn prediction models, applying MLOps best practices to automate marketing campaigns based on churn probabilities. This PoC allowed us to validate the technical feasibility, refine workflows, and validate alignment with business objectives before scaling to full implementation. Discover more details on how PoC helped our client improve customer retention and acquisition.
Read more: Enhancing ecommerce services with ML-powered churn prediction calculation
4. Testing and evaluation
We conduct thorough performance assessments to measure the model's accuracy, speed, and scalability. However, technical performance alone isn't enough. We also assess the business impact-whether the model delivers measurable improvements in critical areas such as cost reduction, operational efficiency, or customer satisfaction.
Based on these assessments, we refine the model further, adjusting parameters and retraining it to enhance accuracy and reliability. We confirm that AI solutions are technically sound and capable of delivering real-world business value.
5. Deployment
The final piece of the puzzle is deployment. Once the AI model has been validated through testing and refinement, the final step is integrating the solution into your business environment. At N-iX, we prioritize seamless integration with existing systems and workflows to minimize disruption. The process involves embedding the AI model into your enterprise's infrastructure-whether it's a cloud-based system, an on-premise solution, or a hybrid setup.
Our focus here is on operationalizing the AI solution, guaranteeing it can process real-time data, generate insights, and contribute to the overall business strategy. Continuous monitoring and support are also critical at this stage, as the model evolves with your changing needs and continues to deliver value over time. That means we are always ready to address evolving risks and optimize the AI model, for example, for scaling or high-volume requests.
WHITE PAPER
Explore the AI landscape of 2025—get the guide with top trends!
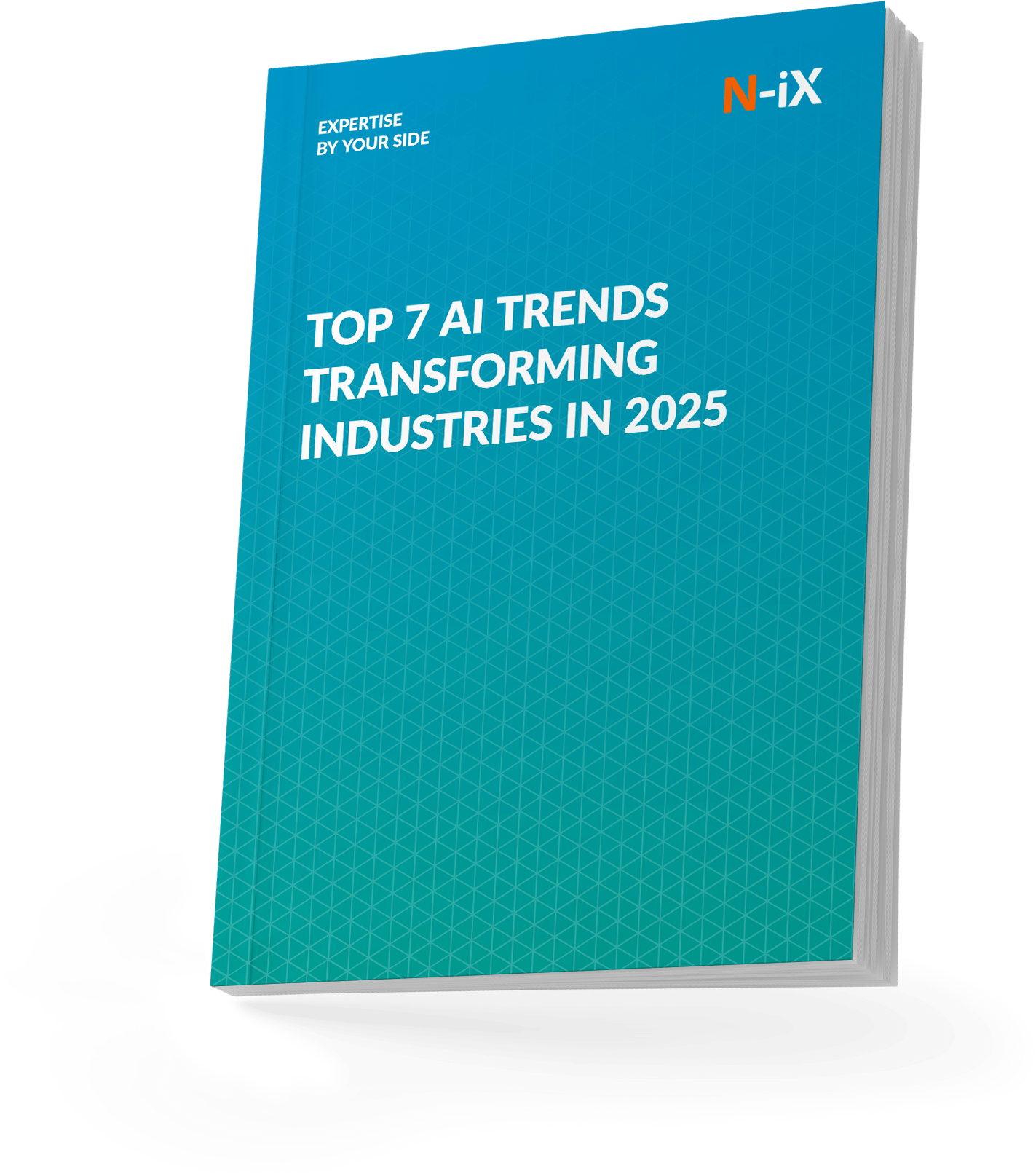
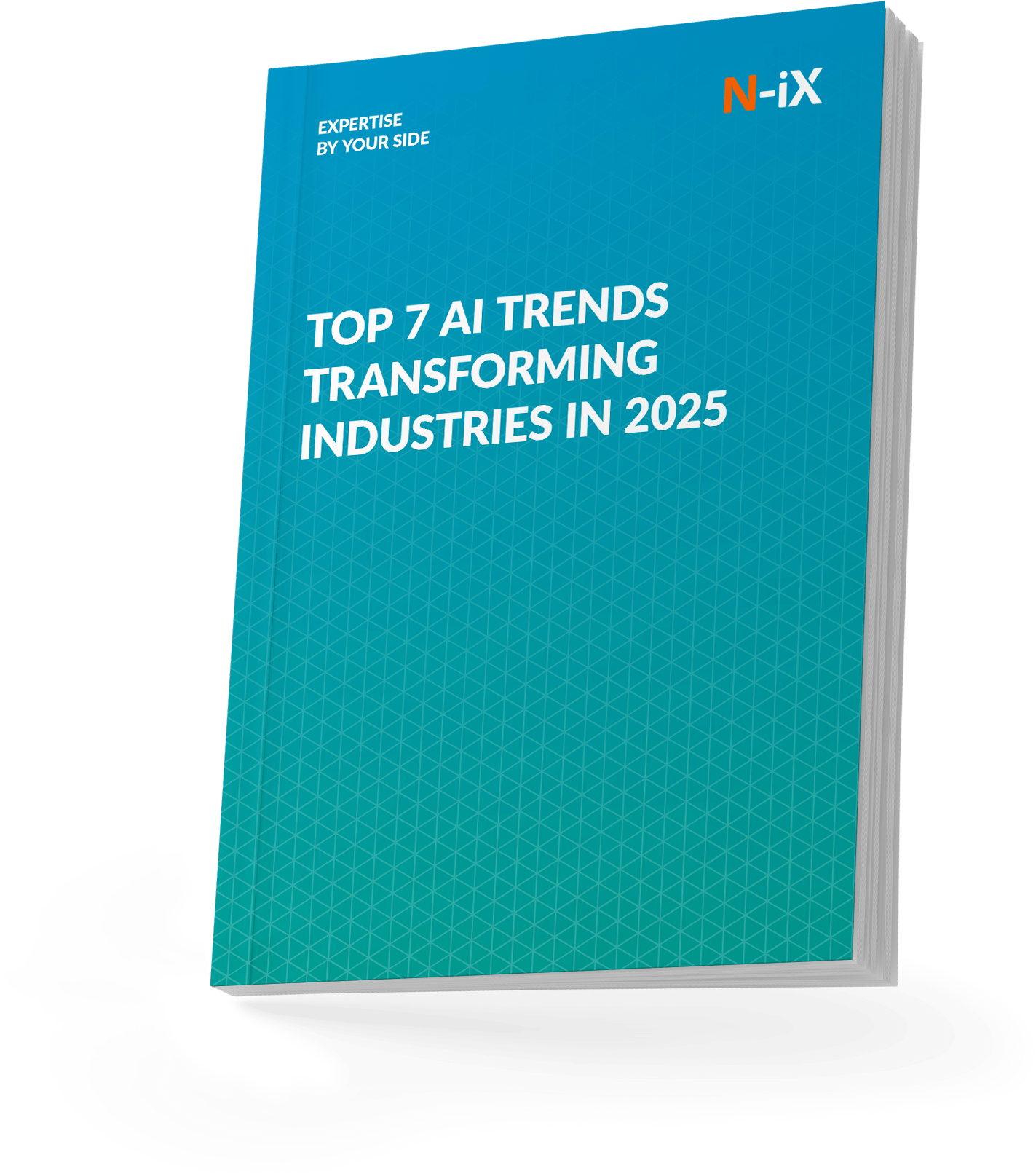
Success!
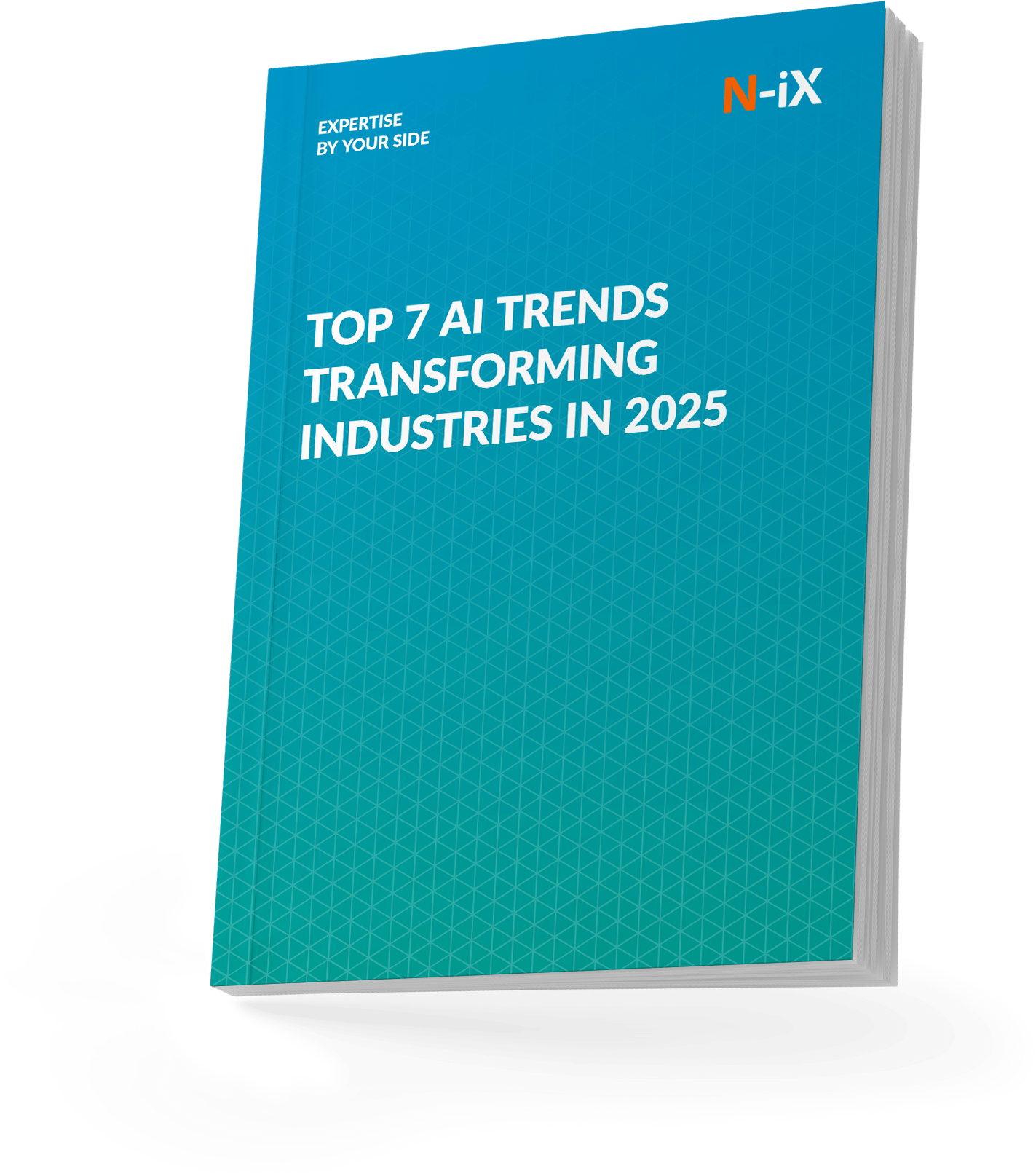
What happens after the PoC project ends?
At N-iX, once an AI Proof of Concept has proven successful, we help enterprises move from concept validation to full-scale implementation. Here's how we guide enterprises through the full-scale AI implementation process.
Refining the AI solution based on PoC insights
The PoC phase is not just about testing a solution; it provides valuable insights into the AI model's performance, data quality, and technical requirements. We take the insights from the PoC AI to fine-tune the AI model, controlling it can handle larger data volumes and more complex tasks as it scales. Achieving this could involve adjusting algorithms, optimizing for real-time data processing, or upgrading the model's accuracy based on feedback from the PoC.
Scaling AI across the enterprise often requires additional infrastructure. N-iX supports businesses migrating to scalable cloud environments like AWS, Azure, or Google Cloud, adapting the infrastructure to meet the increased demand and support AI performance at scale.
Seamless integration into existing business systems
Integrating AI solutions fully into an enterprise's existing systems is a critical and complicated step. At N-iX, we work closely with IT and operations teams to integrate AI models smoothly into the broader digital ecosystem without disrupting workflows or business continuity.
AI models need to integrate seamlessly with legacy systems and existing enterprise software. N-iX builds custom APIs and connectors to facilitate smooth communication between AI systems and other business platforms, minimizing disruption. Moreover, we help enterprises embed AI into business processes, such as automating routine tasks or enhancing decision-making capabilities. This can result in considerable time and cost savings.
Scaling the AI solution
Scaling an AI solution from PoC AI to enterprise-wide deployment requires expanding the technology to consistent performance across the organization. Our AI teams leverage cloud-based solutions to make AI systems scale flexibly. Cloud platforms provide elastic computing power, allowing AI systems to grow in line with the business's evolving needs. By utilizing cloud services, enterprises can handle larger datasets, more users, and higher processing demands.
AI systems deployed at scale often need to process vast amounts of data. N-iX provides the expertise to build robust data pipelines and optimize AI models for big data environments, managing the increased data load without compromising performance.
Continuous AI model improvement and retraining
As part of full-scale implementation, N-iX sets up processes for continuous monitoring and retraining of AI models. Models stay accurate and relevant as new data becomes available and business conditions change.
To enhance the value of AI, we build real-time feedback loops into the system, allowing AI models to learn from new inputs and adjust outputs accordingly. As a result, AI will adapt to new patterns or business changes, making the solution more dynamic and responsive.
Monitoring and maintenance
Once AI is fully operational, it's crucial to have robust monitoring systems in place to track the AI solution's performance and its impact on business outcomes. We implement real-time monitoring tools to track AI performance, quickly identifying and addressing any issues. We continuously track metrics like accuracy, speed, and system uptime to meet business expectations.
Beyond technical performance, we help enterprises measure the business impact of AI implementation. This includes tracking key metrics like ROI, cost savings, efficiency improvements, and overall business growth.
Final thoughts
Jumping straight into full-scale AI deployment without this validation is risky. For decision-makers, a Proof of Concept provides peace of mind. You're not diving into full-scale AI implementation blind-you're validating your approach, identifying risks, and aligning the project with your company's goals. This process helps you avoid costly mistakes and positions your AI efforts for real impact.
At N-iX, we're here to guide you through this process. At N-iX, we have extensive experience guiding businesses through the complexities of AI adoption, ensuring that every phase from PoC AI to full-scale deployment is executed precisely. Our approach is to work closely with your team to navigate these challenges and optimize the AI solution for your unique business context.
- We have a team of over 200 AI experts who are experienced in various types of Machine Learning, data science, and AI solutions
- N-iX has delivered 60+ data projects and helped clients in telecom, fintech, automotive, agritech, manufacturing, and other domains build and scale effective Data analytics ecosystems and optimize costs.
- We have been a long-term technical partner for Fortune 500 companies, helping them launch their AI and Big Data directions and migrate to the cloud.
- Our certified partnership with Google, AWS, and Microsoft provides our AI developers with state-of-the-art tools to create and deliver secure solutions for highly regulated industries like healthcare, finance, pharma, and biotech.
The PoC phase is the first step toward exploring AI's full potential, and we help you take it with confidence. Let's build the future of your business with AI, one step at a time.
Have a question?
Speak to an expert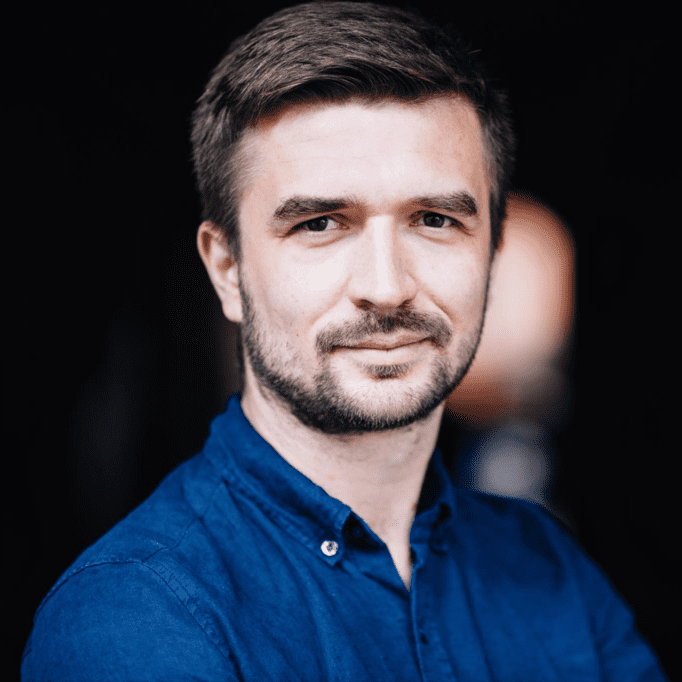