Imagine a critical product running out of stock just as demand surges, leaving frustrated customers looking elsewhere. Or perhaps the opposite happens-warehouses filled with unsold goods, tying up capital and creating waste. These scenarios are all too common for enterprises, and the stakes are high. Inventory mismanagement costs businesses money and reputation.
The challenge isn't a lack of effort; it's the complexity of modern supply chains. But what if inventory management could be more intelligent, faster, and more adaptive? That's where AI consulting services step in. Let's explore how AI inventory management addresses these challenges, what technologies power it, what its use cases are, and how businesses can benefit from effectively adopting it.
How AI inventory management differs from traditional systems
Traditional inventory management relies on manual processes, historical data analysis, and rule-based systems to track stock levels, forecast demand, and replenish supplies. Rather than relying on manual processes or static forecasting models, Artificial Intelligence in inventory management leverages data-driven algorithms to analyze patterns, predict demand fluctuations, monitor in real time, and optimize stock levels. It connects various data points-sales trends, supplier performance, customer behavior, and more-into a unified system.
For example, instead of reacting to stockouts, an AI system can predict demand spikes in advance and ensure sufficient inventory. Similarly, AI can provide data-driven insights to maintain optimal inventory levels rather than overstocking products in anticipation of demand.
AI inventory management offers a proactive and dynamic solution, enabling businesses to:
- Reduced operational costs through optimized stock levels.
- Increased profitability by minimizing waste and improving resource allocation.
- Improved customer experiences through timely product availability and reliable service.
- Enhanced decision-making with predictive analytics.
- End-to-end visibility with real-time inventory tracking.
- Eliminated manual errors with automated replenishment.
- Accurate demand forecasting for better stock planning.
- Optimized inventory levels to reduce waste and storage costs.
WHITE PAPER
Explore the AI landscape of 2025—get the guide with top trends!
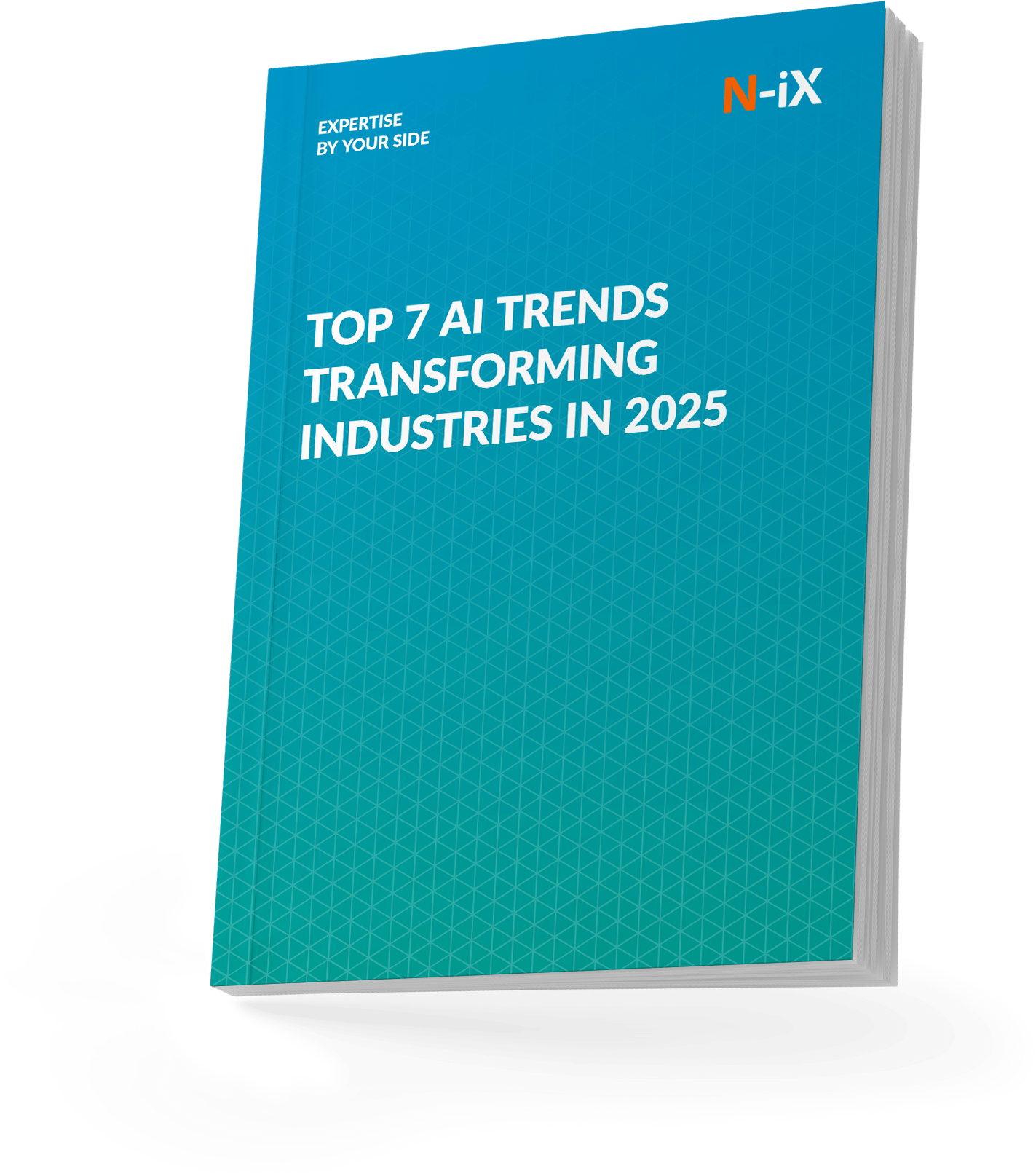
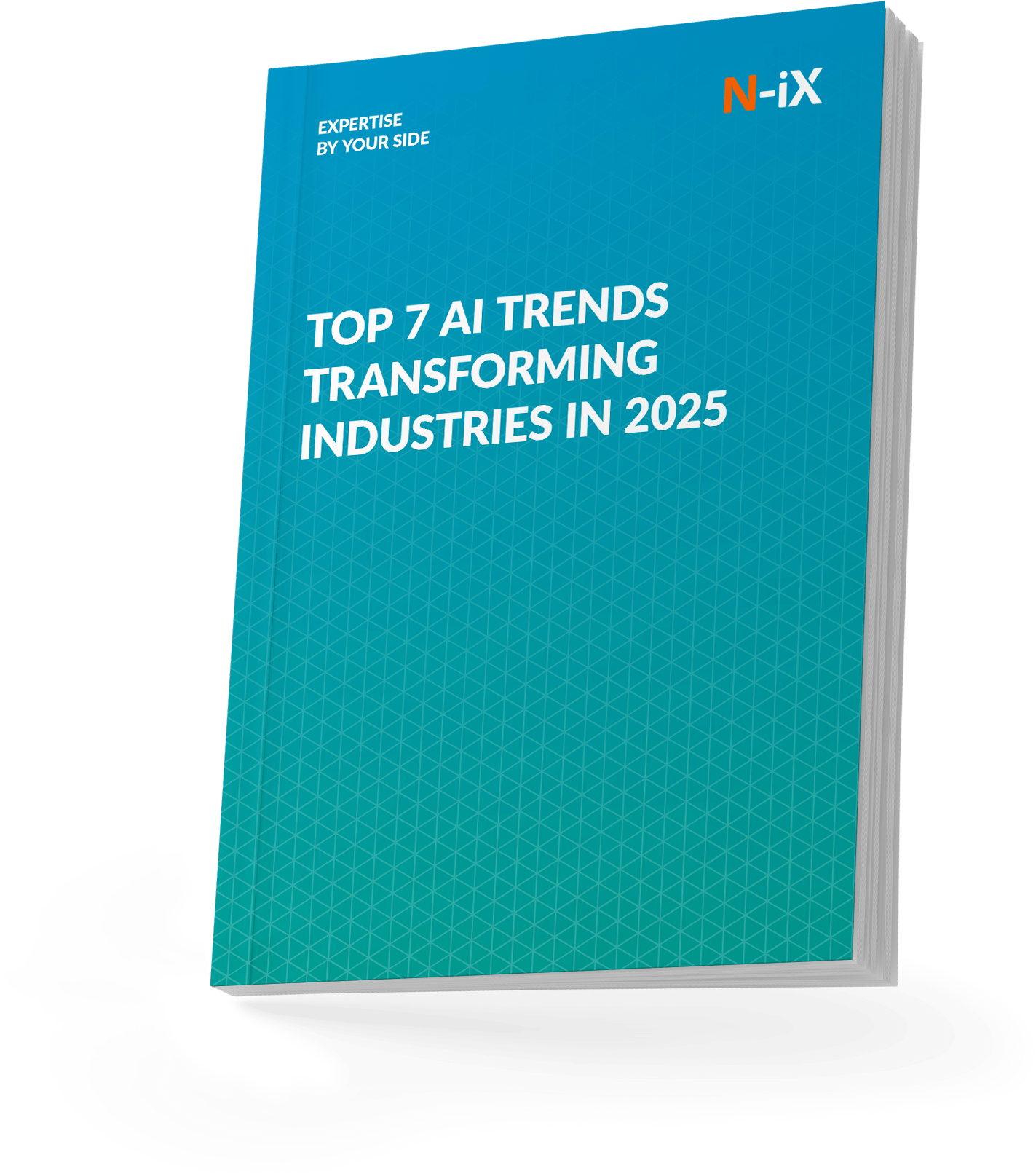
Success!
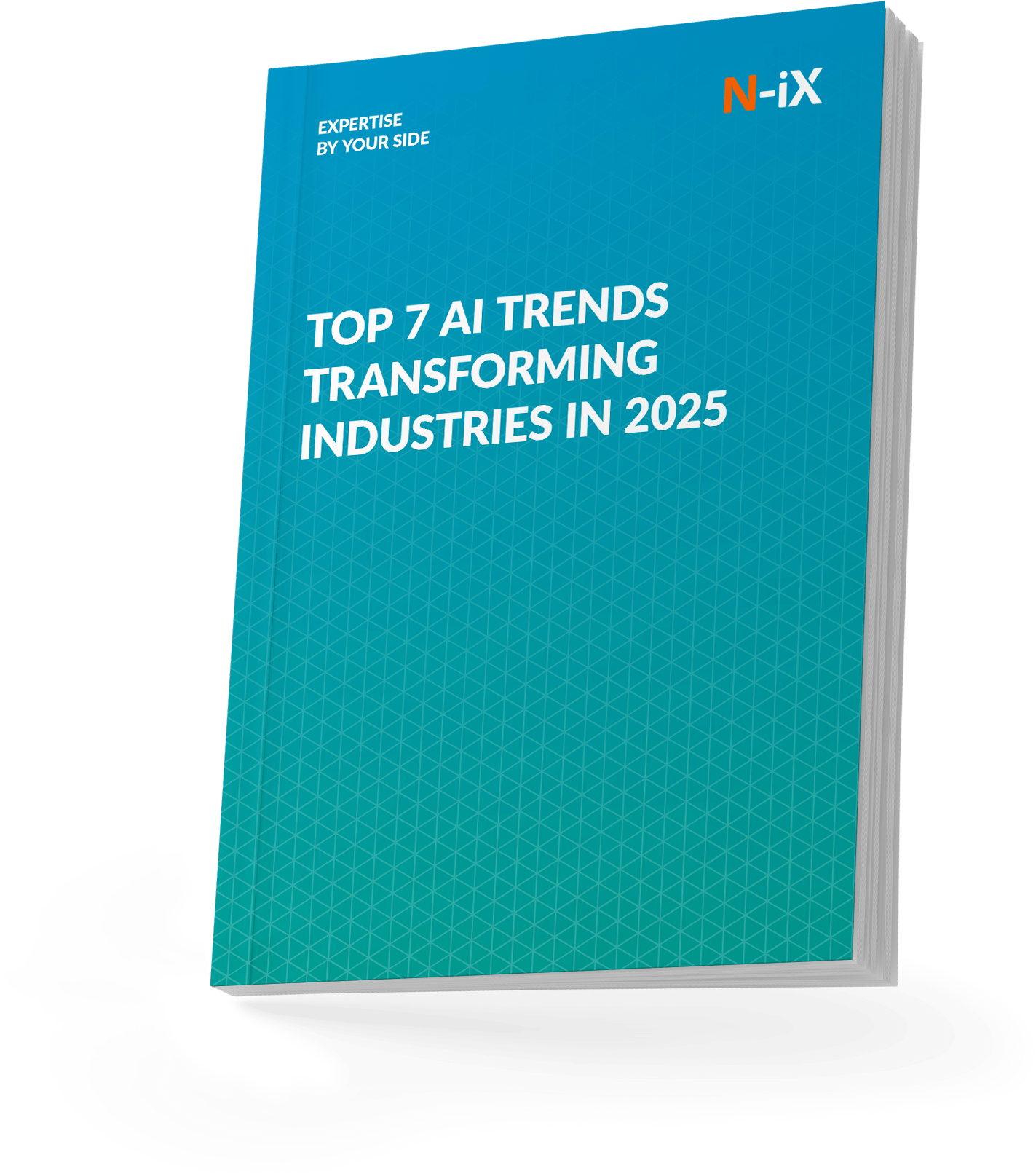
AI technologies behind inventory management
AI in inventory management relies on a combination of advanced technologies to enhance efficiency and accuracy. These technologies process vast amounts of data, identify patterns, and automate critical inventory functions. Let's discover them in detail.
Machine Learning
Machine Learning (ML) enables systems to improve and adapt based on data patterns continuously. Unlike traditional rule-based inventory systems, which rely on predefined thresholds, ML dynamically adjusts to changes in demand, supply chain disruptions, and market trends. Key applications of Machine Learning in inventory management:
- Demand forecasting: ML algorithms analyze historical sales data, seasonal trends, and external variables like economic conditions or social sentiment to accurately predict future inventory needs.
- Anomaly detection: Machine learning detects inconsistencies in stock levels, identifying potential fraud, theft, or reporting errors in real time.
- Inventory optimization: Algorithms determine the ideal stock levels to reduce excess inventory while preventing stockouts, balancing cost efficiency with availability.
- Supplier performance analysis: AI evaluates supplier reliability by analyzing delivery timelines, order accuracy, and defect rates, helping businesses make data-driven procurement decisions.
Through our collaboration with a Global Fortune 100 multinational engineering and technology company, we have helped modernize and scale their logistics and inventory management platform across 400+ warehouses in 60+ countries. By integrating AI-driven automation, ML, and computer vision, we addressed inefficiencies in warehouse operations, enhanced real-time inventory tracking, and streamlined internal logistics.
Read more: Driving logistics efficiency with industrial Machine Learning
Predictive analytics
Predictive analytics uses statistical models, data mining, and AI to anticipate future inventory needs. Traditional inventory planning often relies on past sales data alone, but predictive analytics incorporates multiple factors to provide a more nuanced, forward-looking view. How predictive analytics enhances Inventory management:
- Proactive demand planning: AI models assess various factors-historical demand, supplier performance, lead times, and external market conditions-to ensure the proper inventory levels are maintained.
- Risk mitigation: Predictive models identify potential supply chain disruptions, such as geopolitical instability or raw material shortages, enabling businesses to prepare contingency plans.
- Inventory turnover optimization: Businesses can avoid overstocking slow-moving items and ensure fast-moving products are consistently available, improving cash flow and reducing storage costs.
- Seasonal trend analysis: AI analyzes past seasonal fluctuations, allowing businesses to adjust stock levels ahead of peak periods and prevent shortages or excess inventory.
Computer vision
Computer vision is a key AI technology transforming inventory management by enabling automated stock recognition, tracking, and quality control. By leveraging image recognition and deep learning and computer vision enhance efficiency in warehouses, retail stores, and distribution centers. There are applications of computer vision in inventory management:
- Automated stock counting: AI-powered cameras and drones scan shelves, pallets, and storage areas to detect product quantities, reducing reliance on manual audits.
- Barcode and RFID recognition: Computer vision systems interpret barcodes and RFID tags in real time, improving tracking accuracy and ensuring inventory records are always current.
- Product identification and categorization: AI models analyze product dimensions, packaging, and labels, enabling faster item classification and organization.
- Damage and defect detection: AI-powered inspection systems identify damaged or defective products in warehouses and manufacturing facilities, ensuring only high-quality goods are shipped.
- Automated checkout and loss prevention: Retailers use computer vision to track inventory at self-checkout stations and identify discrepancies between purchases and scanned items.
Natural Language Processing
Natural Language Processing enhances inventory management by enabling AI-powered systems to understand and process human language. This allows for more efficient interactions with inventory databases, supplier communications, and order management systems. Applications of NLP in inventory management:
- Automated order processing: NLP extracts relevant details from emails, purchase orders, and supply chain documents, reducing manual data entry errors and speeding up procurement workflows.
- Sentiment analysis: AI scans customer reviews, social media discussions, and online feedback to detect emerging product demand trends, helping businesses adjust stock levels proactively.
- Supplier communication optimization: NLP-powered AI can process and analyze vendor negotiations, contract terms, and delivery updates.
Use cases of AI inventory management
From retail to manufacturing, AI-driven inventory solutions provide real-time insights, optimize stock levels, and mitigate risks. Below, we examine industry-specific applications of AI in inventory management and how businesses leverage advanced capabilities to improve operational efficiency.
Use cases in retail
Retailers leverage AI to maintain optimal stock levels, anticipate demand shifts, and streamline pricing and replenishment strategies. AI-driven inventory management ensures product availability while minimizing excess inventory and reducing operational costs.
- Demand forecasting: AI-powered models analyze historical sales data, market trends, seasonality, and external variables like economic indicators and social media activity to predict demand.
- Automated replenishment: AI integrates with POS systems and warehouse management software to trigger automated restocking based on real-time inventory levels and predictive insights. This minimizes human intervention and ensures product availability.
- Consumer behavior monitoring: AI inventory management can track economic shifts, weather patterns, and social trends to adjust demand predictions and ensure stock levels match consumer needs.
- Dynamic pricing optimization: AI in retail can dynamically adjusts prices based on real-time demand fluctuations, competitor pricing, and stock availability.
- Returns management: AI optimizes return handling by classifying returned items, predicting resell potential, and efficiently reintegrating inventory.
For example, a retail company prioritizes maintaining a high availability of in-demand products while keeping inventory costs in check. Without AI forecasting and replenishment, it risks missing sales due to stockouts or tying up capital in overstocked items. AI's real-time insights provide the precision to strike the right balance and optimize profitability.
Use cases in supply chain
AI-based inventory management improves efficiency by enhancing transparency, mitigating risks, and optimizing stock movement across networks.
- Supply chain visibility: AI integrates with IoT and RFID technology to track inventory in real-time across warehouses, transportation networks, and retail stores.
- Supplier performance optimization: Inventory management AI analyzes supplier reliability, delivery performance, and historical data to anticipate potential risks and suggest contingency plans.
- Automated material procurement: AI in supply chain streamlines procurement by predicting stock needs, considering lead times, and automating purchase order generation. This ensures stock availability while minimizing carrying costs.
- Inventory redistribution: AI optimizes stock distribution across warehouses and fulfillment centers, dynamically reallocating inventory to match regional demand and reduce logistics costs.
- Logistics optimization: AI-driven logistics platforms analyze transportation data to optimize delivery routes, minimize fuel consumption, and reduce stock replenishment delays.
In the supply chain industry, enterprises may deal with fluctuating supplier lead times, unexpected disruptions, and a growing need for end-to-end visibility. Without AI, manually tracking supplier performance and inventory movement would be inefficient and error-prone. AI in inventory management provides predictive insights, allowing businesses to proactively manage risks, streamline procurement, and optimize logistics costs while ensuring product availability.
Use cases in manufacturing
Manufacturers use AI to align inventory with production schedules, reduce downtime, and minimize waste while ensuring raw materials and components are available when needed.
- Just-in-Time (JIT) inventory management: AI forecasts raw material needs based on production schedules, ensuring stock arrives precisely when required. The application of AI reduces storage costs and prevents over-purchasing.
- Predictive maintenance: AI-powered sensors monitor equipment performance and detect anomalies to predict maintenance needs before failures occur. AI in predictive maintenance prevents unplanned downtime and ensures smooth production flows.
- Inventory tracking: AI-enabled tracking ensures visibility into raw material inventories, preventing shortages that could disrupt production. Automated alerts trigger restocking actions before critical materials run low.
- Waste reduction: AI in manufacturing can analyze production efficiency, material usage, and waste patterns to suggest optimizations that reduce excess material consumption and improve sustainability.
- Automated quality control: AI-powered computer vision systems inspect manufactured goods for defects, ensuring compliance with quality standards and reducing returns and recalls.
In manufacturing, operations where machine downtime or supply chain disruptions could lead to costly production halts. AI's predictive maintenance capabilities can flag failures before they occur, minimizing downtime. AI demand forecasting ensures that the right raw materials are available at the right time, preventing production delays.
Use cases in healthcare
The healthcare industry relies on precise inventory management to ensure the continuous availability of critical medical supplies, pharmaceuticals, and equipment. AI enhances efficiency, minimizes waste, and helps maintain compliance with stringent regulatory standards.
- Inventory tracking: The combination of AI and inventory management can monitor stock levels of essential medical supplies and pharmaceuticals in real-time, ensuring that hospitals and healthcare facilities do not run out of life-saving equipment and medications. Automated alerts trigger reorder processes before shortages occur.
- Expiry date management: AI analyzes usage patterns and expiration dates to prioritize stock rotation, ensuring older items are used first. This reduces waste caused by expired pharmaceuticals and medical supplies.
- Predictive demand forecasting: AI algorithms predict demand for medical inventory based on patient volume trends, seasonal disease patterns, and public health data. This allows hospitals to prepare for surges in demand and avoid overstocking costly inventory.
- Automated procurement: AI assesses supplier performance, delivery timelines, and reliability to recommend the best sourcing options for hospitals and pharmacies. It also automates procurement processes, reducing manual intervention and ensuring timely restocking.
For example, an extensive hospital network faces frequent shortages of essential drugs due to unpredictable demand and delays in supplier deliveries. The hospital maintains optimal stock levels while reducing excess inventory by implementing AI-powered demand forecasting and automated procurement.
Use cases in logistics and warehousing
In logistics and warehousing, AI enhances real-time visibility, streamlines storage and retrieval processes, and improves supply chain efficiency.
- Real-time inventory tracking: AI integrates with IoT devices, RFID tags, and warehouse management systems (WMS) to provide end-to-end monitoring of inventory across multiple locations.
- Warehouse slotting: AI-powered algorithms analyze inventory turnover rates, product dimensions, and picking frequency to optimize warehouse layouts. Dynamic slotting ensures that high-demand products are easily accessible, reducing retrieval times.
- Automated stock replenishment: AI forecasts demand and automatically triggers replenishment based on historical trends, seasonality, and real-time order data. Using AI for inventory management minimizes the risk of stockouts and prevents overstocking of slow-moving items.
- Autonomous warehouse operations: AI-driven robotics, such as Automated Guided Vehicles (AGVs) and robotic arms, assist in picking, packing, and sorting inventory.
- Route optimization: AI analyzes traffic patterns, weather conditions, and order urgency to recommend the most efficient delivery routes. This minimizes transportation costs and improves last-mile delivery efficiency.
If a large logistics company struggles with frequent stock imbalances across its regional distribution centers, it challenges delays in order fulfillment. AI enables to track and identify demand patterns across locations, dynamically redistributing stock to avoid shortages.
Use cases in ecommerce
Ecommerce businesses depend on AI inventory management to maintain seamless operations, optimize stock levels, and enhance customer satisfaction in a highly competitive market.
- Real-time stock synchronization: AI ensures accurate inventory tracking across various sales channels, including online marketplaces, physical stores, and fulfillment centers. This application prevents overselling and stock discrepancies.
- Automated returns management: AI streamlines return processes by analyzing return reasons, automating restocking for undamaged products, and optimizing reverse logistics.
- Demand forecasting: The advanced technologies predict demand spikes during sales events, holidays, and promotions that allows to stock the inventory levels and avoid shortages or excess inventory.
- Personalized inventory recommendations: AI in ecommerce industry analyzes customer behavior, purchase history, and preferences to recommend relevant products.
- Dynamic pricing adjustments: AI continuously monitors competitor pricing, demand fluctuations, and stock levels to adjust pricing strategies in real-time. As a result of AI in inventory management case study, it helps maintain competitiveness while maximizing profitability.
For example, an online retailer struggles with stock shortages during flash sales, leading to lost sales and customer dissatisfaction. AI inventory management helps anticipate demand surges and prevents overselling by synchronizing stock levels across multiple sales channels.
Implementing an AI for inventory management: What should you pay attention to?
Adopting AI in inventory management is not just about integrating new technology-it requires a strategic approach to data, system compatibility, and operational change management. N-iX works with enterprises to implement AI solutions that optimize inventory processes and reduce inefficiencies. Below are the key considerations when integrating AI into inventory management.
Data integration
AI's effectiveness depends on the accuracy and comprehensiveness of the data it processes. If historical inventory records, sales data, and supply chain inputs contain errors, the AI model's predictions will be flawed. Before implementation, we assess:
- Are inventory records comprehensive, including supplier lead times, seasonal trends, and demand fluctuations?
- How reliable are stock levels, order history, and sales forecasts?
- Are different business units using standardized formats and metrics for inventory tracking?
We focus on data cleansing, normalization, and integration with enterprise resource planning (ERP), warehouse management systems (WMS), and supply chain platforms to ensure a successful AI deployment.
Integration with existing systems
The integration of AI must seamlessly integrate with existing business infrastructure. Many enterprises operate across multiple platforms-ERP, WMS, order management, and supplier relationship management (SRM) systems. Fragmented technology stacks create barriers to AI implementation. We consider the following considerations:
- Does the AI solution integrate with existing software?
- Can real-time inventory data be exchanged across platforms?
- Does the AI model align with the company's IT strategy?
We ensure a smooth transition by developing integration roadmaps, comprehensive enterprise AI strategy, identifying potential system bottlenecks, and leveraging cloud-based AI platforms for scalable deployment.
Scalability
AI inventory management software must evolve alongside business growth, adapting to increasing complexity, expanding operations, and shifting market dynamics. A rigid system that cannot scale efficiently leads to bottlenecks, inefficiencies, and outdated forecasting models. We assess these factors for AI scaling solutions.
- AI models must handle large datasets, increase order volumes, and expand supply chain networks without performance degradation. Scalability requires an adaptive machine learning framework that continuously refines its forecasting models, improving predictions as new data becomes available.
- Managing inventory across multiple sales channels-including brick-and-mortar stores, e-commerce platforms, warehouses, and third-party logistics providers (3PLs)-demands real-time inventory visibility and seamless synchronization.
- A scalable AI solution should dynamically adjust stocking strategies, modify replenishment triggers, and optimize distribution based on real-time demand signals.
We build enterprise-grade AI inventory management solutions that scale with evolving business demands, ensuring efficiency gains without costly system overhauls. Our ISG-recognized data engineering capabilities and certified team of experts in data and cloud technologies provide:
- Design AI models that scale with transaction volumes, maintaining predictive accuracy as businesses expand.
- Develop modular AI architectures that seamlessly integrate with ERP, WMS, and supply chain platforms.
- Leverage cloud-based infrastructure for scalable computing power and real-time data processing.
- Enable automated cross-channel synchronization to unify inventory data across all sales and fulfillment points.
Data governance framework
AI models are only as effective as the data they process. A strong data governance framework ensures data consistency, accuracy, and accessibility across all inventory touchpoints. We implement AI-powered data cleansing and normalization techniques. Specifically, work on:
- Centralized inventory data management to unify datasets across ERP, WMS, and supply chain systems.
- Automated data validation to detect and correct inconsistencies in stock records.
- Seamless integration with IoT sensors, RFID systems, and POS data for real-time inventory tracking.
- Scalable data architecture that can process high transaction volumes without compromising accuracy.
Final thoughts
Inventory optimization goes beyond cost control. It is about accurately forecasting, managing, and distributing the right inventory to the right locations at the right time to meet demand efficiently. The days of manual, paper-based inventory tracking are fading, replaced by intelligent systems capable of optimizing operations and supporting long-term growth.
However, successful AI implementation goes beyond adopting new software, it requires careful planning, high-quality data, and a clear roadmap. We work with enterprises to implement AI and make it work for their industry challenges, whether it's predictive demand forecasting in retail, supply chain visibility in logistics, or real-time stock monitoring in ecommerce. With a team of 200+ AI and ML experts, we drive measurable business outcomes-lower costs, reduced waste, improved customer satisfaction, and increased supply chain resilience.
Consider not whether AI should be part of your inventory strategy but how soon you can start leveraging its potential for your needs.
Have a question?
Speak to an expert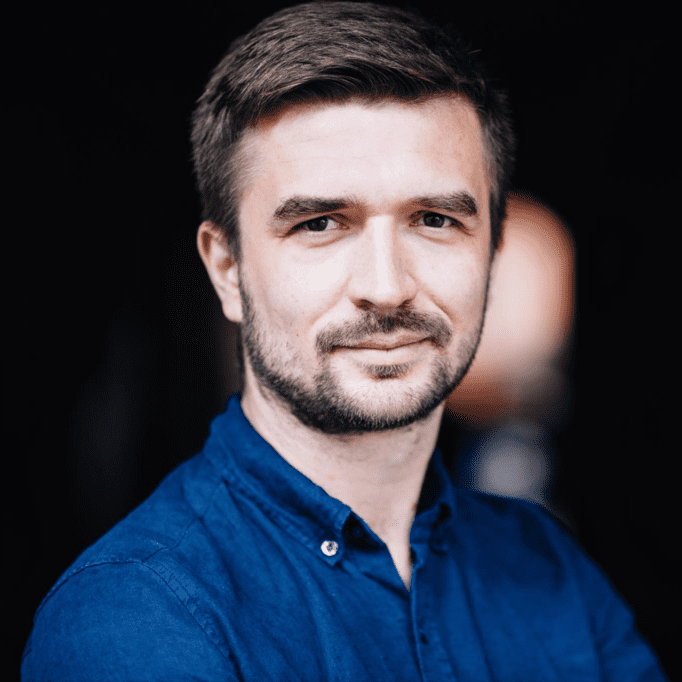