AI enhances robotic capabilities, enabling machines to perform complex tasks with autonomy, precision, and adaptability. While traditional robots excel at high-speed, repetitive tasks in controlled environments, they follow pre-programmed instructions and lack the ability to learn or adapt. Unlike conventional robots, AI-powered ones can perceive, analyze, and respond to their environment in real time. Artificial Intelligence in robotics allows machines to optimize workflows, improve accuracy, and adapt to new conditions without manual reprogramming.
However, successfully implementing AI in robotics requires specialized expertise in both robotics and AI. Businesses must ensure seamless AI integration, real-time processing, and scalable automation, which can be challenging without the proper technical foundation. This is why companies turn to robotics consulting and AI consulting services to develop high-performance AI-driven robotic solutions.
What are the most efficient use cases of AI-powered robotics? How to implement AI in robotics and avoid making costly mistakes? Let’s find out.
Technologies that power AI in robotics
Machine Learning
Machine Learning enables robots to recognize patterns, learn from data, and improve their performance over time without requiring manual reprogramming. Instead of following rigid, pre-defined rules, ML models generalize from past experiences, allowing robots to adapt to new environments, optimize movements, and enhance decision-making.
ML enables robots to perform predictive maintenance, dynamic path planning, and real-time decision-making, making it a core component of autonomous robotics.
Deep Learning
Unlike traditional ML, which often requires feature engineering, Deep Learning (DL) allows robots to automatically extract relevant information from raw data, making it particularly powerful for perception and high-level reasoning.
DL enhances a robot’s ability to process unstructured data, recognize patterns, and continuously refine its performance, making it essential for modern robotics applications such as autonomous navigation, industrial automation, and medical robotics.
Computer Vision
Instead of relying solely on predefined rules, machines equipped with Computer Vision (CV) analyze images and video streams to detect patterns, recognize objects, and precisely track movement. This capability is required for robots operating in unpredictable environments where real-time perception and decision-making are required.
Advancements in 3D mapping, depth perception, and motion tracking have pushed the boundaries of what robots can perceive and analyze. The ability to interpret spatial relationships with greater accuracy allows robots to navigate crowded spaces, collaborate with humans more intuitively, and perform complex visual tasks across various industries.
Sensor integration
A robot's ability to function effectively depends on how well it integrates and processes data from multiple sensors. Cameras, LiDAR, ultrasonic sensors, gyroscopes, accelerometers, and radar all play a role in providing real-time environmental data. However, raw sensor data is often noisy, redundant, or incomplete, and therefore, Sensor Fusion is one of the most critical aspects of sensor integration. It combines data from multiple sources to compensate for individual sensor weaknesses. For example:
- Autonomous vehicles: Merge LiDAR, cameras, and radar for reliable obstacle detection in low-visibility conditions.
- Drone navigation: Fuse GPS with inertial measurement units to maintain stability when GPS signals are weak.
- Medical robotics: Combine force sensors and computer vision to ensure precision in robotic-assisted surgeries.
N-iX specializes in sensor fusion and real-time data processing, developing solutions that enhance robotic perception, increase situational awareness, and improve overall performance.
Use cases of AI in robotics
AI-driven robotics transforms industries by enhancing automation, optimizing decision-making, and enabling real-time adaptability. Unlike traditional automation, using AI for robotics helps predict failures before they happen, reduce downtime, and create dynamic, responsive workflows. Below is a breakdown of AI’s impact across industries, including proven applications and emerging innovations.
Manufacturing
AI-powered robotics in manufacturing enhances efficiency, improves quality control, and optimizes production workflows.
Autonomous production lines
AI-driven robots self-adjust to material variations, environmental conditions, and product types, ensuring consistent manufacturing without human intervention. Machine learning enables adaptive assembly processes, minimizing defects and optimizing efficiency.
AI-driven predictive maintenance
Instead of waiting for failures, AI models analyze sensor data, predict wear, and schedule maintenance proactively. This approach reduces downtime, extends equipment lifespan, and improves factory efficiency. N-iX leverages AI-driven monitoring systems to ensure real-time anomaly detection in industrial robotics.
Cobots as intelligent assistants
Collaborative robots (cobots) assist in repetitive tasks, assembly, and quality control in factories worldwide. Universal Robots and Fanuc produce cobots that work together with human operators to improve efficiency and safety. Future advancements could include LLM-powered cobots that interpret verbal instructions, structure directives, and orchestrate workflows. While this technology is still emerging, it represents the next phase of AI-driven manufacturing.
Examples:
- Siemens and ABB Robotics implement AI-driven automation in industrial robotics;
- Tesla uses AI-powered robots for adaptive manufacturing and real-time quality control;
- N-iX develops AI-based solutions for manufacturing, including defect detection, predictive maintenance, and smart control systems.
Supply chain and industrial operations
AI optimizes logistics and supply chains by reducing inefficiencies, improving inventory management, and enabling predictive planning.
Dynamic demand forecasting and logistics optimization
AI analyzes historical and real-time data to predict demand shifts, adjust production schedules, and optimize supply chain operations. SAP and Microsoft leverage AI-powered predictive supply chain models to improve resource allocation. N-iX integrates AI-driven forecasting solutions that minimize shortages and prevent inventory overload.
Intelligent warehouse automation
AI-powered robots adjust picking, sorting, and transport strategies dynamically, ensuring fast and accurate order fulfillment. Unlike rigid automation, AI-based warehouse robotics react to real-time demand, traffic flow, and equipment conditions.
Connected supply chain and predictive workflows
AI eliminates blind spots in logistics by synchronizing production, transportation, and inventory data, creating a self-optimizing ecosystem that reduces inefficiencies and predicts bottlenecks before they occur.
Examples:
- Ocado applies AI-driven robotics for high-speed order processing;
- N-iX develops AI solutions for warehouse robotics, autonomous logistics, and predictive analytics to improve supply chain efficiency.
Learn more about robotics in logistics
Healthcare
AI-driven robotics transforms healthcare through automated diagnostics, robotic-assisted surgeries, and predictive patient care.
Embedded robotic medical assistants
AI-powered medical devices monitor vital signs, detect anomalies, and schedule doctor visits autonomously. Smart implants for heart monitoring and glucose regulation already exist, but fully autonomous AI-driven healthcare decision-making remains an emerging field.
AI-enabled remote surgery
AI-assisted robotic surgery is widely adopted in medical centers worldwide, with systems like the Da Vinci Surgical System enabling surgeons to perform minimally invasive procedures with enhanced precision and control.
AI-based neurological monitoring and intervention
AI-powered neurological monitoring systems are advancing the early detection and management of seizures, neurological disorders, and mental health conditions. AI-driven EEG analysis platforms track brain activity in real time, identifying abnormal patterns linked to epileptic seizures, sleep disorders, and cognitive impairments. Some AI-driven platforms also provide real-time alerts to medical professionals or caregivers, enabling faster response times and reducing risks for patients with chronic neurological conditions.
Examples:
- Medtronic has developed AI-assisted closed-loop neuromodulation systems, which adjust electrical stimulation levels in real time to treat conditions such as Parkinson’s disease and epilepsy;
- Neuralink is advancing brain-computer interfaces (BCIs) that could enable AI-assisted communication and movement restoration for patients with neurological impairments;
- N-iX works on AI-driven medical imaging analysis, robotic process automation in healthcare, and predictive diagnostics to support healthcare professionals.
Agriculture
AI-driven robotics automates crop monitoring, livestock care, and precision farming, improving efficiency, sustainability, and yield.
AI-powered precision farming
AI-powered drones and robotic systems analyze soil quality, moisture levels, and plant health, optimizing irrigation and fertilization based on real-time conditions. This approach improves sustainability and maximizes crop yield.
Autonomous harvesting and sorting
AI-powered robotic harvesters identify ripe produce, sort crops, and adjust harvesting strategies dynamically. Automated sorting systems improve efficiency and reduce post-harvest losses.
Livestock monitoring and automated care
AI-powered systems track livestock health, detect early signs of disease, and automate veterinary care. These robots reduce human labor costs and improve overall herd management.
Examples:
- John Deere develops AI-powered tractors with autonomous navigation;
- Agrobot produces robotic harvesters for precision fruit picking;
- Blue River Technology applies ML to detect and manage weeds;
- N-iX develops AI models for agricultural automation and predictive analytics to optimize resource use and productivity.
Automotive
AI in robotics enhances vehicle automation, predictive maintenance, and driver safety, making mobility smarter and more efficient.
AI-powered predictive safety systems
AI anticipates unpredictable pedestrian behavior, such as a child running into the street to retrieve a ball. Advanced driver assistance systems (ADAS) integrate sensor fusion, deep learning, and trajectory prediction to initiate proactive safety measures, reducing accident risks.
Autonomous vehicle optimization
AI enhances real-time sensor fusion, decision-making, and object recognition, ensuring self-driving cars adapt to dynamic road conditions. However, autonomous vehicles still face legal, ethical, and technological challenges before full adoption.
AI-driven automotive production and maintenance
AI-powered vehicle telemetry analysis detects mechanical wear, predicts failures, and schedules proactive maintenance. These solutions reduce downtime, improve vehicle longevity, and enhance fleet efficiency.
Examples:
- Tesla develops AI-powered autonomous driving systems;
- Mobileye enhances driver-assistance technologies with Deep Learning;
- Waymo advances the development of fully autonomous vehicles
- N-iX develops AI-based automotive diagnostics, advanced driver assistance systems, and autonomous mobility solutions for the automotive industry.
Implementing AI in robotics: A step-by-step approach by N-iX
N-iX provides end-to-end AI-driven robotics solutions, ensuring seamless integration, optimal performance, and long-term scalability. Our experts combine deep expertise in Machine Learning, Computer Vision, Sensor Fusion, and AI model optimization to help your company leverage robotics for automation, precision, and efficiency.
1. Feasibility assessment
Our AI and robotics specialists begin with a comprehensive assessment of your business objectives and operational challenges. We analyze your existing infrastructure, data availability, and integration requirements to determine the best approach for AI deployment.
Our team also conducts a cost-benefit analysis, ensuring the proposed solution aligns with your budget, scalability goals, and expected ROI. Success metrics—such as increased efficiency, improved accuracy, or reduced operational costs—are established to guide the implementation process.
2. Process simulation
Before full-scale deployment, we develop digital twins and AI-driven simulations to test robotic workflows in a controlled environment. Through advanced simulation tools, our AI specialists validate how robots interact with complex environments, unstructured data, and dynamic tasks.
Whether your project requires autonomous navigation, intelligent sorting, robotic precision handling, or predictive maintenance, simulations allow us to eliminate inefficiencies, refine AI behavior, and ensure seamless integration before physical deployment.
3. Robotics implementation
Once the AI models are optimized, our team integrates robotics systems into your operational environment, ensuring smooth interaction between AI algorithms, sensor networks, and mechanical components.
We configure real-time data processing, edge AI computing, and cloud connectivity, enabling your AI-powered robots to analyze sensor inputs, make autonomous decisions, and adapt to new conditions instantly.
4. Stabilization phase
Following deployment, our AI engineers work closely with your team to monitor, refine, and optimize robotic performance. We analyze real-time operational data to improve AI accuracy, enhance robotic movement precision, and adjust to evolving production variables.
Feedback from your operators and robotics teams is incorporated to fine-tune AI models for better adaptability, efficiency, and reliability. Our experts provide hands-on training and support, ensuring smooth collaboration between human workers and AI-powered robotic systems.
5. Expansion and optimization
Once your AI-driven robotic systems are stabilized, N-iX helps scale your automation capabilities across multiple production lines, warehouses, medical facilities, or agricultural operations. Our AI specialists continuously refine machine learning models, allowing your robots to adapt to new environments, handle more complex tasks, and improve decision-making over time.
We also integrate advanced AI functionalities, such as predictive analytics for supply chain automation, real-time anomaly detection in manufacturing, or AI-powered surgical robotics enhancements. Regular system updates and performance monitoring ensure your AI-driven robotics solutions remain competitive, efficient, and future-proof.
Common pitfalls in AI-driven robotics and solutions by N‑iX
Implementing AI in robotics comes with challenges that affect efficiency, adaptability, and cost. N-iX ensures AI models are scalable, accurate, and cost-effective, mitigating key risks with advanced development strategies.
Data quality and availability
AI-powered robots depend on large datasets for training and real-time decision-making. Incomplete, biased, or noisy data reduces model accuracy, causing errors in object detection and decision-making. Many industries struggle with limited labeled datasets, making it difficult to develop reliable robotic perception systems.
N-iX enhances data reliability through advanced preprocessing, synthetic data generation, and domain adaptation. Our experts build custom pipelines to filter noise, balance datasets, and improve accuracy. Where real-world data is scarce, synthetic data augmentation ensures AI models remain robust, while sensor fusion improves perception and reliability.
Limited generalization and adaptability
AI models trained in controlled environments often fail in real-world, unpredictable settings. A robot optimized for structured warehouse navigation may struggle outdoors, requiring frequent retraining.
We improve AI adaptability with transfer learning, reinforcement learning, and domain adaptation. Our models adjust to new environments without full retraining, reducing downtime and increasing scalability. Reinforcement learning enables robots to self-optimize based on real-time feedback, ensuring consistent performance in dynamic conditions.
High deployment and maintenance costs
AI-driven robotics requires significant investment in hardware, training, and maintenance. Ongoing model updates and system optimization increase costs, making AI adoption expensive for many businesses.
N-iX reduces costs with modular AI development, cloud-based deployment, and automation-driven MLOps. Our pre-trained models and transfer learning techniques lower training expenses while maintaining high accuracy. Cloud-integrated robotics solutions optimize resource allocation, allowing businesses to scale AI automation without excessive infrastructure costs.
N-iX technology stack for AI-driven robotics
N-iX provides full-cycle AI robotics development, integrating model-based design, real-time control, and cloud infrastructure to build intelligent, scalable robotic systems. Our approach leverages both traditional and model-based methodologies, allowing us to optimize development time, enhance accuracy, and improve system verification.
We combine MATLAB/Simulink modeling, ROS2 for robotic control, and AWS for cloud-based fleet management to create AI-powered robotics solutions that are adaptive, efficient, and enterprise-ready.
1. Development, modeling, and simulation
To ensure high-precision robotic behavior, N-iX utilizes advanced simulation environments for prototyping, testing, and optimization before real-world deployment.
- Model-based development with MATLAB & Simulink: Our experts use MATLAB & Simulink along with Simulink, SimScape, SimMechanics, Control System Toolbox, and Stateflow to model complex robotic systems, design control algorithms, and perform physics-based simulations. These tools help streamline system behavior representation, automate code generation, and improve verification processes.
- Advanced AI and robotics system toolboxes: We integrate ROS Toolbox and Robotics System Toolbox to seamlessly connect AI-driven simulation models with real-world robotic frameworks, allowing an efficient transition from simulation to deployment.
- 3D simulation for virtual testing: Platforms like Gazebo and NVIDIA Isaac Sim allow us to train AI models in high-fidelity, physics-based environments, enabling robots to navigate, interact, and make intelligent decisions before physical deployment.
- Programming languages: Our engineers develop AI perception models, motion planning algorithms, and real-time control systems using Python for machine learning and data analysis and C++ for high-performance robotics applications.
2. Middleware for robot control
To ensure seamless communication between AI models, robotic hardware, and enterprise systems, N-iX integrates advanced middleware solutions for real-time robotic control.
- ROS2 (Robot Operating System 2): Our robotics engineers implement ROS2-based frameworks for navigation (Nav2), motion planning (MoveIt 2), and embedded systems (Micro-ROS), enabling adaptive and precise robotic movements.
- Data Distribution Service (DDS) for scalable robotics: We utilize DDS to enable real-time data exchange across distributed robotic systems, ensuring reliable and low-latency communication in multi-robot environments.
- High-performance transport mechanisms: Our solutions incorporate FastRTPS and CycloneDDS, optimizing robot-to-robot and robot-to-cloud communication for high-speed decision-making in industrial automation, autonomous vehicles, and AI-driven logistics.
3. Cloud infrastructure
For large-scale robotics deployments, cloud-based management and AI-driven updates are critical. N-iX integrates scalable cloud solutions to optimize remote monitoring, real-time AI updates, and fleet coordination.
AWS RoboMaker for scalable robotics deployments: We leverage AWS RoboMaker to enable cloud-based simulation, AI-driven model updates, and remote fleet management, allowing our clients to scale robotic operations efficiently while maintaining real-time performance monitoring.
Transform your manufacturing processes with AI: Discover key use cases in the white paper!
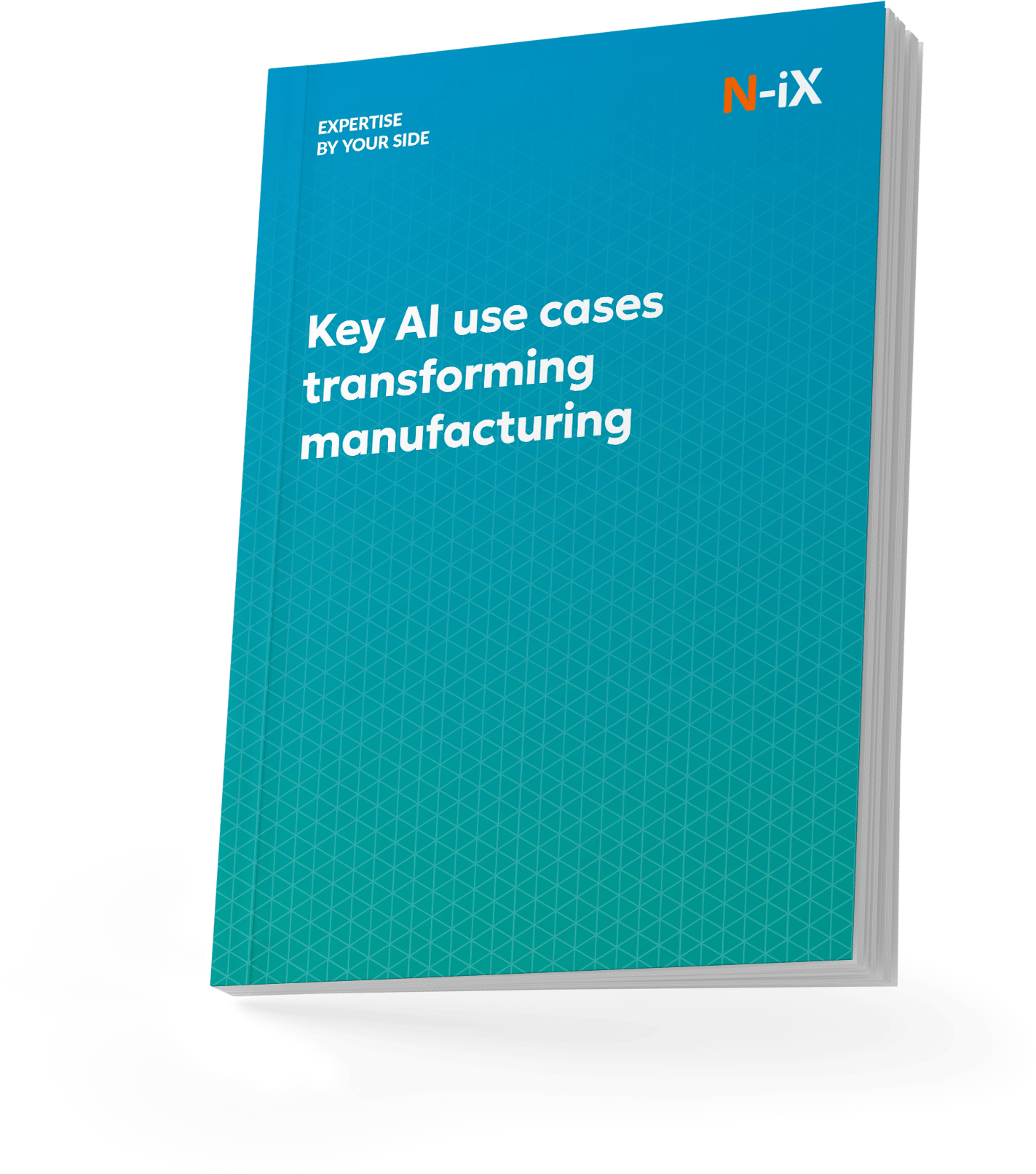
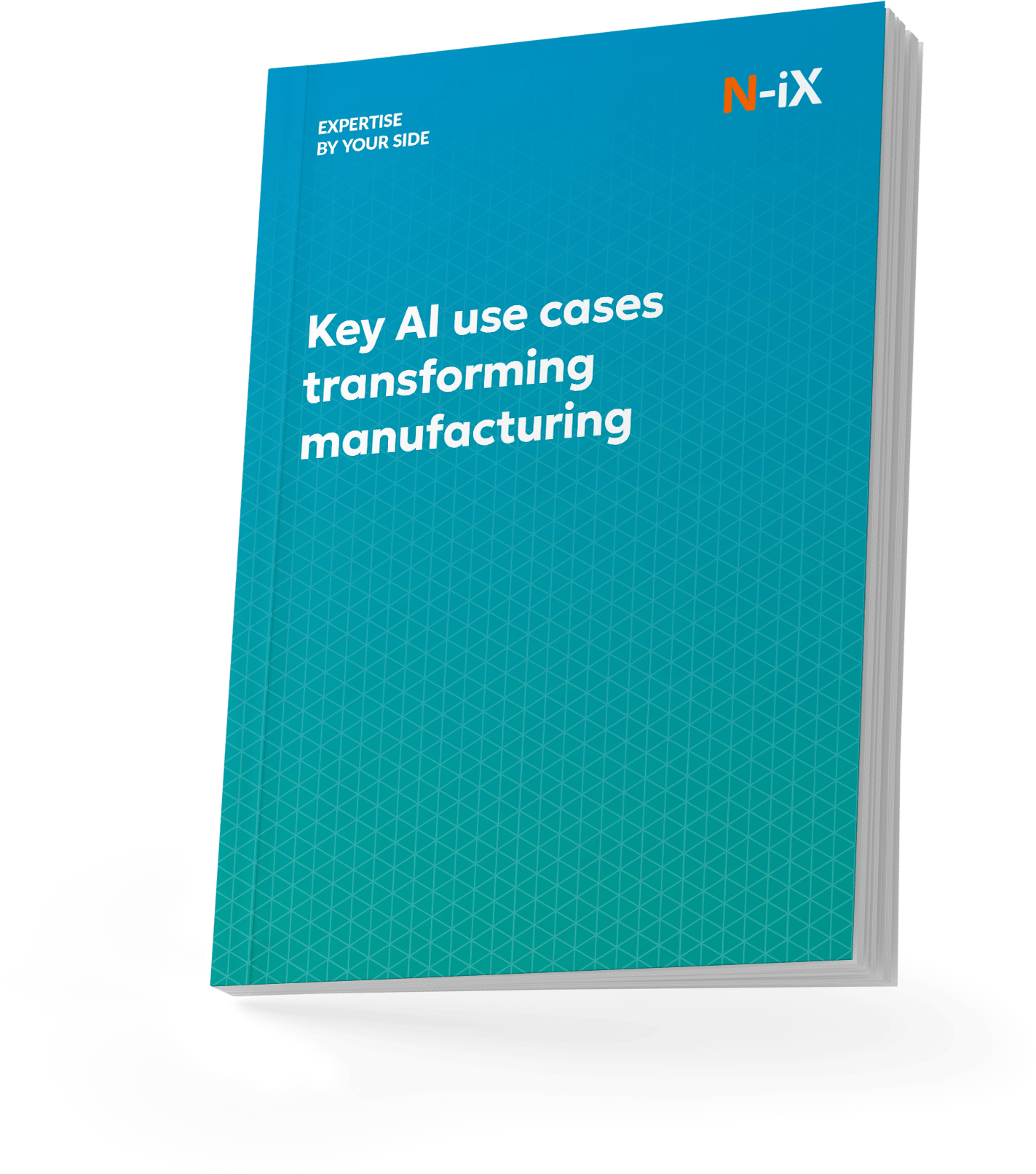
Success!
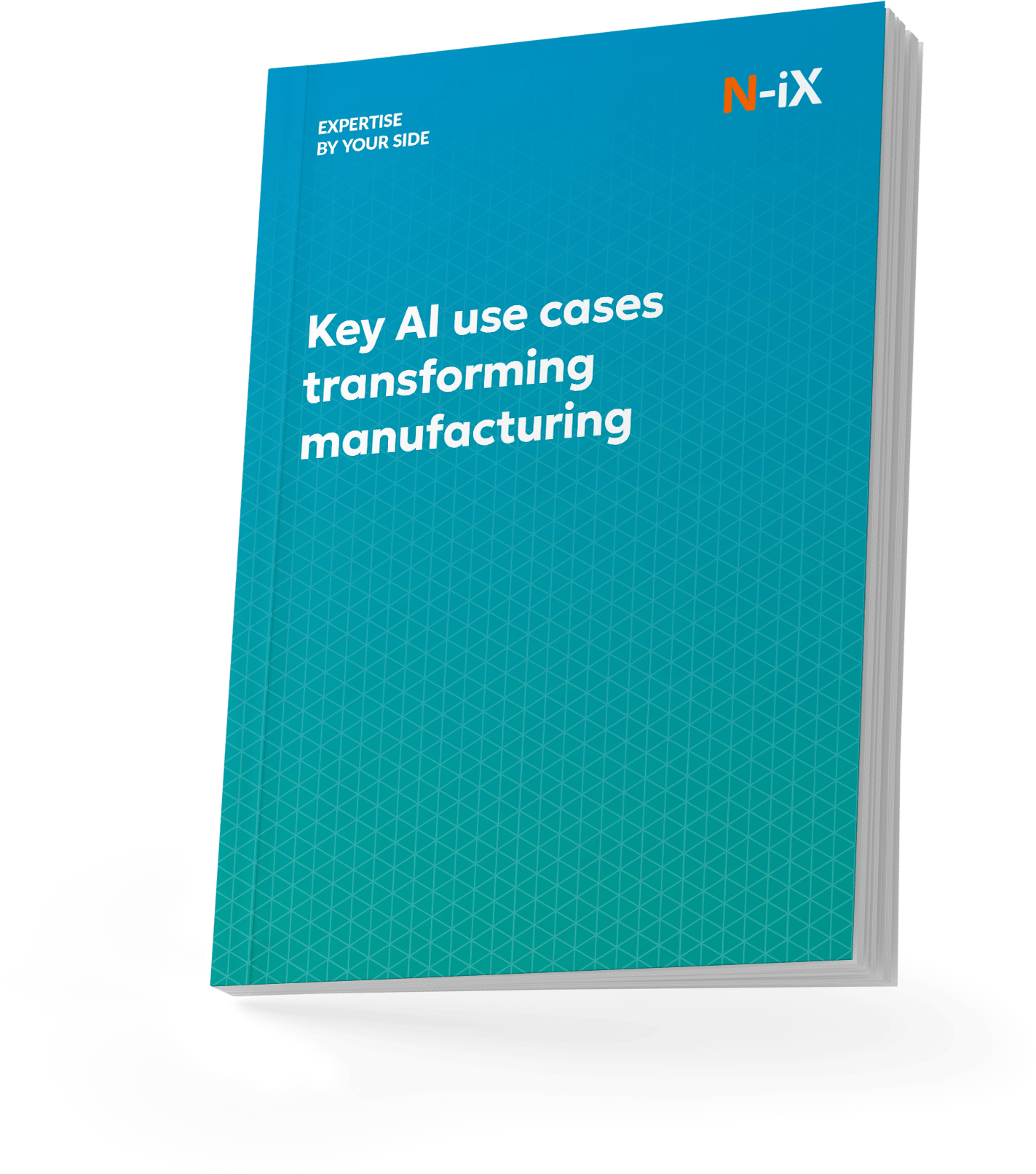
Conclusion
AI-powered robotics transforms industries by eliminating inefficiencies, enabling predictive automation, and enhancing real-time decision-making. While many AI-driven applications are already deployed, others remain in active development due to technological, regulatory, and ethical considerations.
As AI continues to evolve, robotics will become more autonomous, adaptive, and capable of handling complex workflows with minimal human intervention. N-iX is driving this transformation by developing AI-powered solutions for industrial automation, predictive maintenance, healthcare diagnostics, autonomous systems, and smart supply chain management, helping businesses scale automation with efficiency, precision, and reliability.
Have a question?
Speak to an expert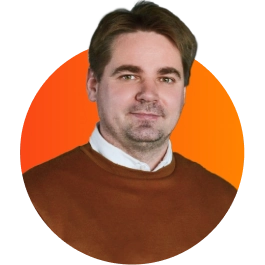