Risk management involves predicting potential losses and finding the strategy that is most likely to minimize them. AI, fundamentally a probability calculation tool—it fits perfectly into this process. From the moment a customer completes a registration form to the strategies deployed at the end of the customer lifecycle, AI in risk management enhances every step of the process. Innovation in data science has long played a key role in banking and financial software development. With the recent surge in AI advancements, 80% of credit-issuing organizations plan to integrate AI into business processes within the next year [1]. The industry recognizes AI's potential to enhance decision-making, streamline operations, and reduce risks in the financial sector. However, there are still questions surrounding the potential applications and consequences of AI. Let’s explore what AI can achieve in credit risk management and the best practices for its implementation.
How AI improves risk management across the customer lifecycle
Traditional credit scoring models rely on fixed, conventional criteria—such as income, employment history, credit scores, and repayment history—to assess creditworthiness. While completely reasonable to the human eye, rigid systems might fail to capture nuanced or dynamic indicators of a borrower's financial health or changing circumstances. Accenture finds that the financial impact of inefficiencies in loan underwriting will cost the financial industry $160B over the next five years [2]. AI in credit scoring can evaluate risk factors purely based on data and real outcomes without being influenced by conventional assumptions or biases that can cloud judgment. Let’s look at the top use cases for AI in credit risk management:
Read more: AI in Fintech: Use cases and best practices
Loan processing
AI in credit risk management can be employed starting from the very first time the customer fills out a loan application and continuing until the loan is repaid. At each stage, the algorithms estimate the risk and choose the most effective strategy to mitigate it:
- Application. Generative AI can turn loan applications into an intuitive dialogue in natural language or provide real-time assistance with pre-made forms. Simplifying the process and clarifying any potential confusion instantly improves the user experience and reduces errors. Fewer mistakes ensure more accurate data for risk evaluation, minimizing errors in assessing an applicant's risk profile.
- Document verification: AI algorithms streamline the verification of documents like IDs, tax returns, and pay stubs, ensuring accuracy, authenticity, and compliance while reducing manual errors. Predefined rules do not limit these systems; they can handle various document types, formats, and languages, allowing them to service more applicants. While verifying documents, AI tools can view a comprehensive picture of the application to spot inconsistencies, detect heightened fraud risks, and alert the system.
- Credit check: AI can run both traditional and flexible credit checks, offering a more comprehensive view of the applicant's financial behavior. Transaction histories, payment records, and employment data can be evaluated using algorithms to evaluate the applicant’s financial stability and repayment capacity.
- Collateral evaluation: When relevant, the same principle can help instantly evaluate the collateral for a loan. A more accurate model reduces the risk of under- or overvaluing collateral, protecting the lender in case of default by ensuring they can recover the loan amount through the pledged assets.
- Decision: By combining all variables, AI can process available data points to predict approval or rejection decisions in real-time. This increased speed significantly improves the customer experience, whether the organization allows the AI to make decisions autonomously or merely suggest likely outcomes. If the customer does not qualify for the product, personalization features might direct the rejected applicants toward alternative products or adjust qualifying terms. Offering alternatives increases the chances that a customer will find a suitable product, potentially leading to higher overall approval rates.
- Back office automation: While processing the application, AI can automatically extract, process, and organize data from loan applications and supporting documents. This eliminates the need for manual data entry, reduces errors, and speeds up the application review process. AI can classify and route documents to the appropriate departments or personnel based on their content.
Credit risk modeling
AI in credit risk management for direct credit risk prediction has a number of significant advantages over traditional models.
First, AI is capable of working with unstructured data, such as emails or transaction histories, which traditional models typically cannot process. This allows AI to draw insights from a wider range of data sources, providing a more comprehensive view of an applicant's financial behavior and creditworthiness.
Second, AI models can learn and adapt over time, continuously refining their predictions as new data becomes available, whereas traditional models rely on static rules and historical patterns that may become outdated. Third, AI can process and analyze data in real-time, enabling faster, more accurate credit decisions, reducing processing times, and improving operational efficiency for lenders. Overall, AI is a superior mathematical model for credit risk prediction.
However, credit risk prediction is a highly sensitive area of financial institution's operations, and decisions about its modification are serious. Building a model that would take advantage of all the benefits of AI while avoiding the downsides is complex. We will talk about it in the next section.
Personalized offering and pricing
With increased automation and AI’s ability to process vast amounts of data quickly, financial providers can now continuously tailor offerings based on specific customer risk profiles. If an applicant's credit card spending exceeds their ability to repay timely, the algorithm may tighten the loan terms to reduce risk. As a borrower’s circumstances change—such as an increase in income or a decrease in debt—AI can instantly modify terms, keeping products relevant and appealing. This dynamic personalization boosts approval rates for suitable candidates and reduces customer churn, as borrowers are more likely to stay engaged with products that fit their current financial situations.
Research by Forrester indicates that personalized banking experiences can increase the effectiveness of cross-selling products by nearly four times [3]. It can be reasonably inferred that applying similar personalization strategies in lending could yield equally significant improvements, leading to higher engagement and more successful loan offers.
Additionally, generative AI plays a crucial role in terms of transparency by communicating with the customers in their preferred manner and level of expertise. This helps borrowers understand what factors influence their offers and what they can do to improve their credit standing. A transparent, data-driven system empowers customers to make better financial decisions, improving their eligibility for more attractive terms in the future.
Ultimately, AI-driven personalization creates a financial ecosystem that benefits both lenders and borrowers. Lenders can reduce risk by offering precise, individualized terms, while borrowers receive incentives to maintain healthier financial habits, promoting overall financial well-being.
Read more: A guide to personalized banking implementation
Portfolio monitoring
AI in credit risk management can be used to detect early warning signs of potential defaults, such as changes in the macroeconomic climate. The algorithms can spot trends in spending and repayments across customers and suggest adjustments to mitigate the heightened risk.
Even before such an event occurs, AI can simulate stress scenarios like economic downturns or market volatility to assess how a portfolio would perform under adverse conditions. These simulations use data-driven segmentation of assets (rather than relying on human-defined categories) to evaluate how well the portfolio is diversified. Using AI in credit risk management provides a more accurate understanding of risk distribution across different asset segments, ensuring better preparedness for financial shocks.
Being more informed on the risk exposure of one’s portfolio also aids in compliance with regulatory requirements, automating the generation of real-time compliance reports and reducing manual intervention.
Collections and communication optimization
AI in credit risk management can predict which borrowers are likely to miss payments, enabling early interventions with tailored solutions to improve recovery rates. The algorithms would prioritize collection efforts by ranking accounts according to repayment likelihood, allowing teams to focus on high-risk cases and allocate resources more efficiently.
AI also creates personalized repayment plans that consider a borrower's financial situation, making terms more flexible and increasing the chance of successful debt recovery while preserving a positive customer relationship. Additionally, AI in credit risk management identifies the best communication channels and timing based on past behavior, enhancing engagement without being overly aggressive. It can even recommend which collection agents should handle specific cases, matching them based on customer needs—whether it's a more empathetic approach for distressed customers or a skilled negotiator for high-risk cases.
Finding the confidence to implement AI in credit risk management
While AI offers many advantages in credit risk management, it faces challenges related to data quality, model transparency, and regulation compliance. In the EU, regulators have established three requirements for AI models used in credit risk prediction to ensure safe and ethical implementation: Explainability, Fairness, and Robustness. These criteria ensure that AI decisions are transparent, unbiased, and reliable even in changing conditions. Similar guidelines are expected to be adopted in other regions as AI use in finance continues to grow.
Explainability
AI models are "black boxes," meaning they don't explain how their predictions are made. This lack of transparency can make it difficult for regulators, lenders, and borrowers to understand and assign responsibility for credit decisions. There are multiple ways to provide some explainability to the model’s predictions:
- Feature importance is a technique thatidentifies which variables or inputs impact the model’s predictions most. For instance, an AI model might show that credit score, debt-to-income ratio, and recent missed payments are the most influential factors in determining credit risk.
- Monotonicity constraints ensure that the relationship between specific input features and the risk score does not change, meaning that a variable can always negatively or positively influence the risk. This ensures that the model reacts to input in decently predictable ways and will not generate different outcomes from the same input.
- Counterfactual explanations provide insight into how changes in certain inputs would alter the model’s predictions. For example, if a loan application was rejected, the model can generate scenarios like, "If your income had been $5,000 higher or your debt $10,000 lower, your loan would have been approved." This allows applicants or stakeholders to see exactly what factors influenced the decision and how they can adjust to improve future outcomes, offering a clear and actionable understanding of the AI’s reasoning.
Other techniques can be used to understand AI’s reasoning better, but ultimately, the exact weights the model assigns to parameters are hidden.
Fairness
AI systems are only as good as the data they're trained on. If historical data contains biases—like lending decisions that unfairly discriminate against certain groups—AI models might perpetuate or even worsen these biases.
To prevent this, the model should be trained on large, diverse datasets that don't include demographic data but still represent a wide range of backgrounds. This will not allow AI to discriminate based on secondary demographic characteristics, like zip codes or employment history. A common mistake in compiling training data is balancing the dataset as a whole but neglecting to distribute the rejected group. Since rejections are a smaller data subset, any statistical errors or biases in this group are amplified, potentially leading to unfair credit risk assessments. Fairness metrics such as demographic parity, equal opportunity, or disparate impact can check if the rejection rate is consistent across various demographic groups.
Robustness
AI models must be robust and adaptable to shifts in data, market conditions, or events like economic crises. Without robustness, a model might become unhelpful when faced with new data patterns or disruptions. For example, if the economic situation worsens, the model might reject all applications since they don’t meet the requirements established before the downturn. To maintain reliability, models must be regularly tested, stress-tested, and updated to reflect changing realities and risks.
AI models need to be trained on a diverse set of data sources that capture a wide range of economic conditions, customer behaviors, and demographic factors. This prevents the model from overfitting to specific patterns or biases found in limited datasets. Data scientists have a set of tools that help manage the training of a model and make sure it is appropriately effective across all types of inputs.
While AI can automate much of the decision-making process, there is a risk of over-reliance. Data scientist and credit risk analyst oversight is still needed to ensure that AI models align with business and ethical guidelines and to make exceptions for edge cases where the AI might misinterpret data. Ultimately, implementing AI is not about eliminating all AI-related risks but rather creating systems and tools that allow businesses to confidently identify and manage those risks within the boundaries of the company's risk tolerance and culture.
Why choose N-iX to build your credit risk AI model
When you partner with N-iX to build an AI model for credit risk management, you can expect:
- Expertise in AI and ML: We have a strong track record in designing AI solutions to boost efficiency and push innovation in finance and banking, among other industries. Our developers and finance industry experts are experienced in creating secure solutions and navigating complex industry regulations.
- Operational maturity: We offer one-stop-shop AI outsourcing services with secure, ready-to-use infrastructure aligned with the industry standards and data processing requirements (ISO, GDPR) and development methodologies.
- Delivery excellence: Our certified partnership with Google, AWS, and Microsoft provides our AI developers with state-of-the-art tools to create and deliver secure solutions for highly regulated industries.
- Proven track record: We have a proven track record of successful AI development projects. For instance, we've helped a large provider of prepaid cards and current accounts from the UK to develop an ML-powered payment processing solution.
WHITE PAPER
Stay ahead in banking—explore 6 tech trends shaping the industry in 2025!
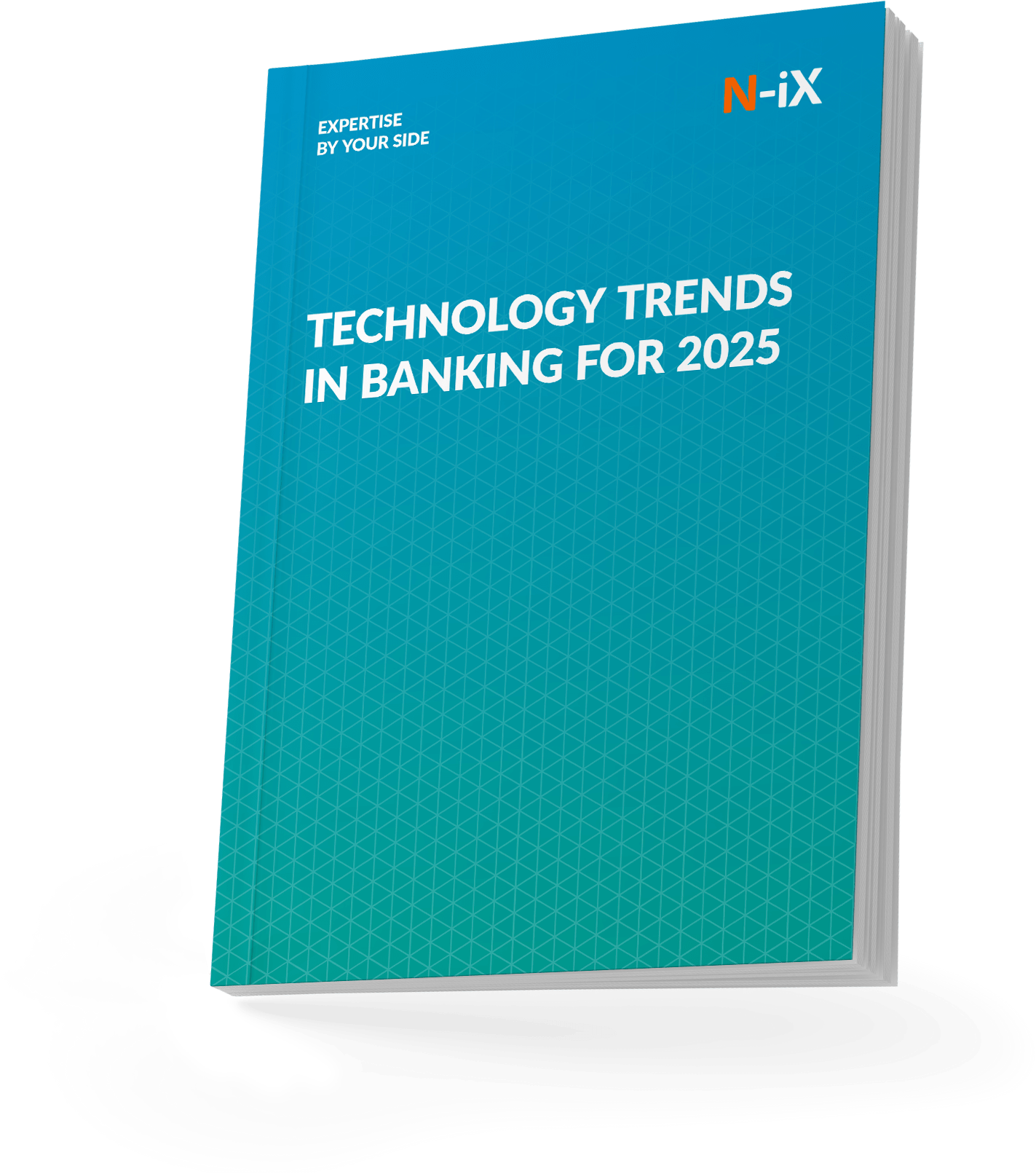
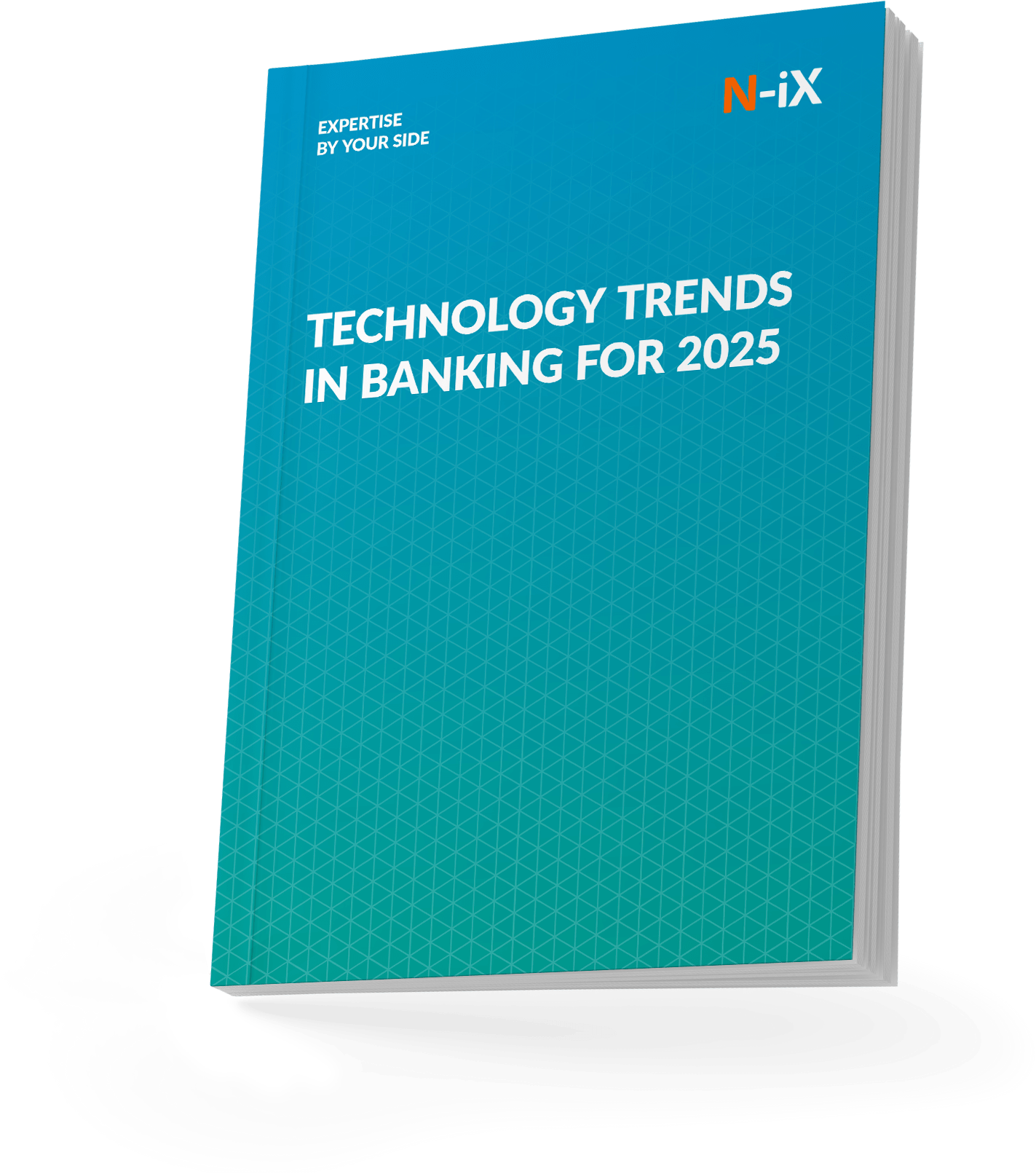
Success!
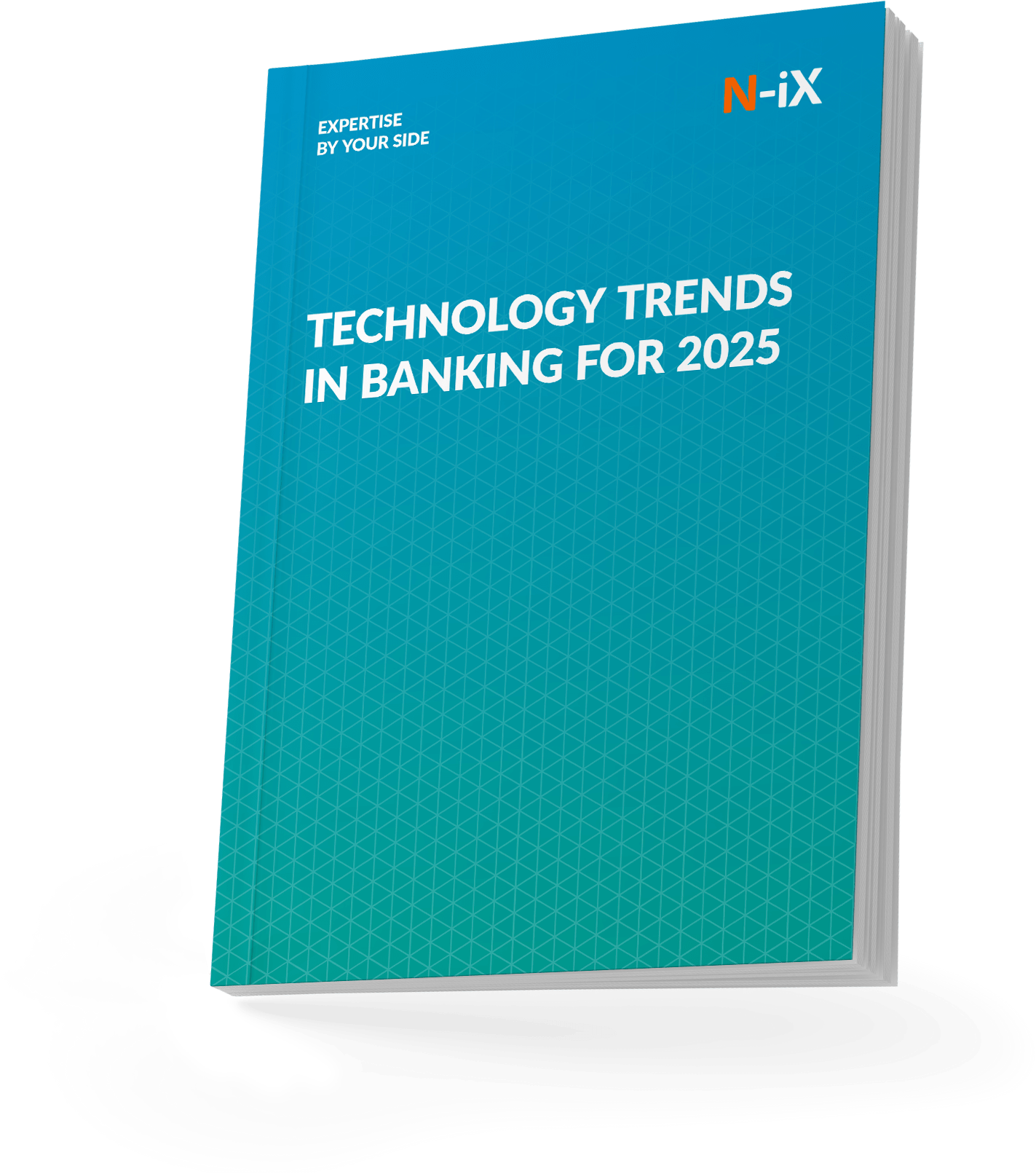
Wrap up
AI is revolutionizing credit risk management by enabling data-driven decisions that are faster, more precise, and adaptable to changing financial landscapes. As AI technology continues to mature, it offers a path to enhanced efficiency and accuracy, from streamlining loan processing to improving portfolio monitoring. Yet, successful implementation requires careful consideration of transparency, fairness, and robustness to ensure the benefits of AI are fully realized without compromising ethical and regulatory standards.
References
- Embracing generative AI in credit risk, McKinsey 2024
- AI in loan underwriting, LeewayHertz
- Unlocking Hyper-Personalization At Hyper-Scale, Forrester 2023
Have a question?
Speak to an expert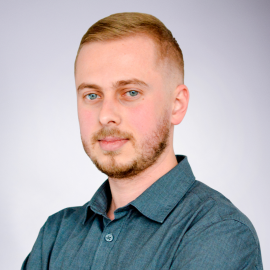